Metagenomic Binning using Connectivity-constrained Variational Autoencoders
ICML 2023(2023)
摘要
Current state-of-the-art techniques for metagenomic binning only utilize local features for the individual DNA sequences (contigs), neglecting additional information such as the assembly graph, in which the contigs are connected according to overlapping reads, and gene markers identified in the contigs. In this paper, we propose the use of a Variational AutoEncoder (VAE) tailored to leverage auxiliary structural information about contig relations when learning contig representations for subsequent metagenomic binning. Our method, CCVAE, improves on previous work that used VAEs for learning latent representations of the individual contigs, by constraining these representations according to the connectivity information from the assembly graph. Additionally, we incorporate into the model additional information in the form of marker genes to better differentiate contigs from different genomes. Our experiments on both simulated and real-world datasets demonstrate that CCVAE outperforms current state-of-the-art techniques, thus providing a more effective method for metagenomic binning.
更多查看译文
AI 理解论文
溯源树
样例
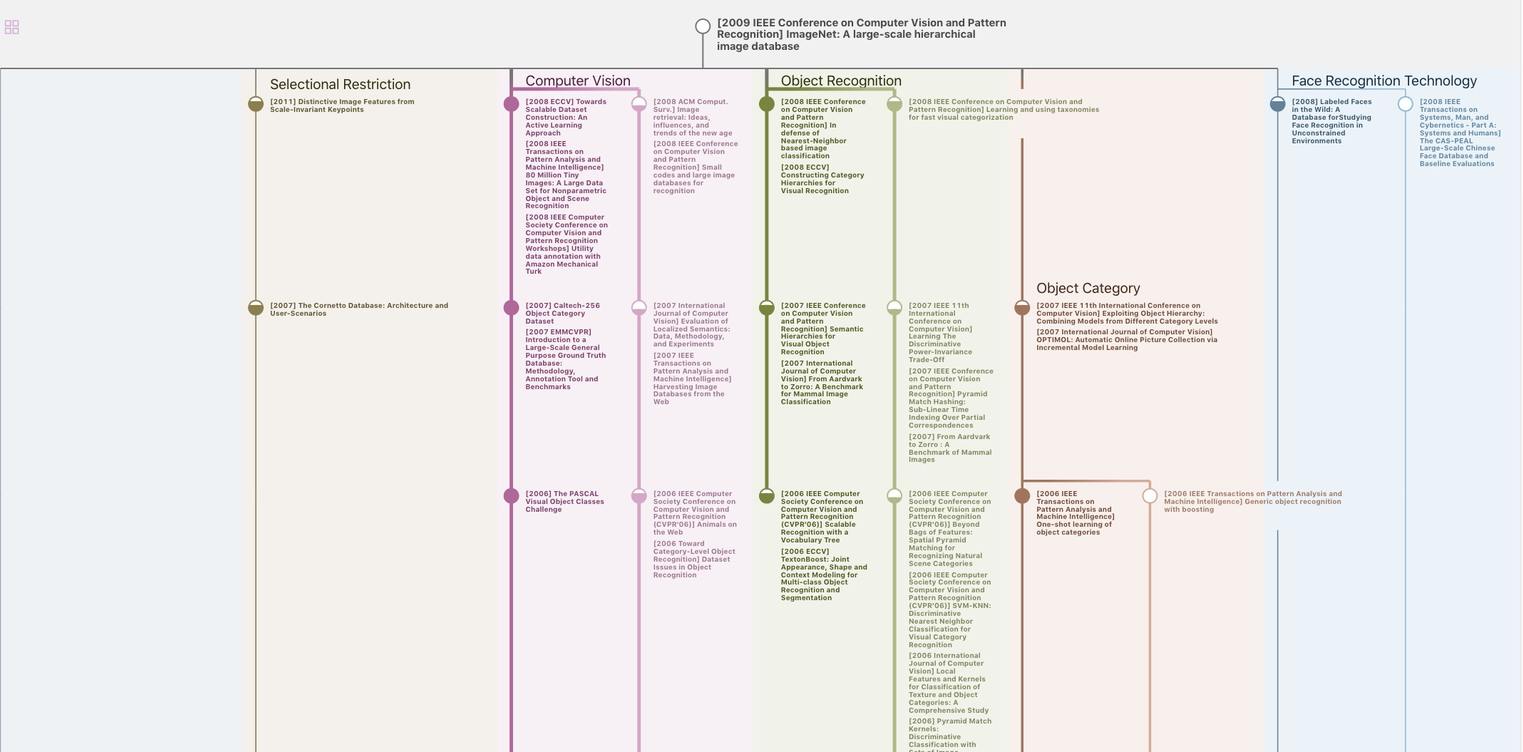
生成溯源树,研究论文发展脉络
Chat Paper
正在生成论文摘要