Alleviating the Long-Tail Problem in Conversational Recommender Systems
PROCEEDINGS OF THE 17TH ACM CONFERENCE ON RECOMMENDER SYSTEMS, RECSYS 2023(2023)
摘要
Conversational recommender systems (CRS) aim to provide the recommendation service via natural language conversations. To develop an effective CRS, high-quality CRS datasets are very crucial. However, existing CRS datasets suffer from the long-tail issue, i.e., a large proportion of items are rarely (or even never) mentioned in the conversations, which are called long-tail items. As a result, the CRSs trained on these datasets tend to recommend frequent items, and the diversity of the recommended items would be largely reduced, making users easier to get bored. To address this issue, this paper presents LOT-CRS, a novel framework that focuses on simulating and utilizing a balanced CRS dataset (i.e., covering all the items evenly) for improving LOng-Tail recommendation performance of CRSs. In our approach, we design two pre-training tasks to enhance the understanding of simulated conversation for long-tail items, and adopt retrievalaugmented fine-tuning with label smoothness strategy to further improve the recommendation of long-tail items. Extensive experiments on two public CRS datasets have demonstrated the effectiveness and extensibility of our approach, especially on longtail recommendation. Our code is publicly available at the link: https://github.com/Oran- Ac/LOT- CRS.
更多查看译文
关键词
Conversational Recommender System,Pre-trained Language Model,Long-tail Problem
AI 理解论文
溯源树
样例
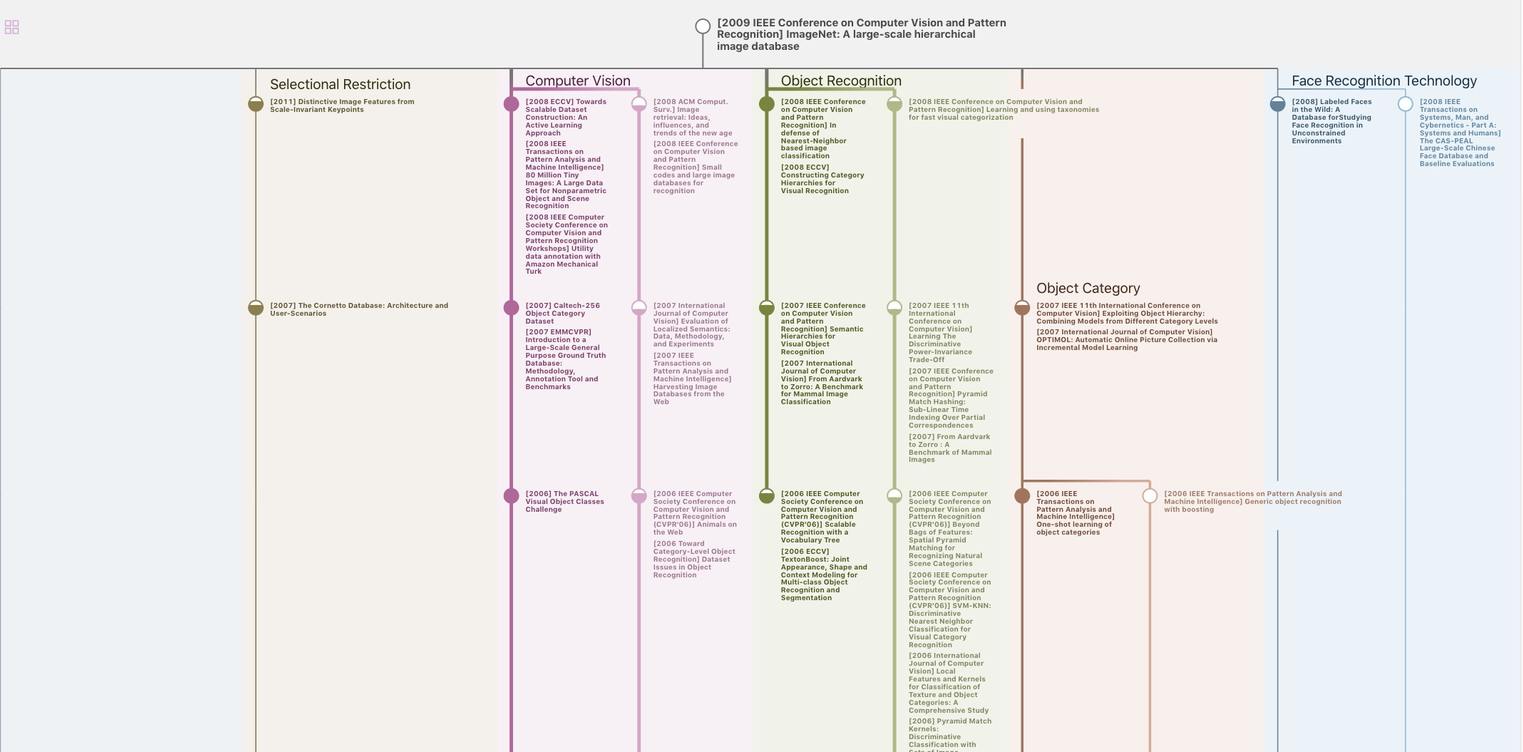
生成溯源树,研究论文发展脉络
Chat Paper
正在生成论文摘要