Identifying document similarity using a fast estimation of the Levenshtein Distance based on compression and signatures
CoRR(2023)
摘要
Identifying document similarity has many applications, e.g., source code analysis or plagiarism detection. However, identifying similarities is not trivial and can be time complex. For instance, the Levenshtein Distance is a common metric to define the similarity between two documents but has quadratic runtime which makes it impractical for large documents where large starts with a few hundred kilobytes. In this paper, we present a novel concept that allows estimating the Levenshtein Distance: the algorithm first compresses documents to signatures (similar to hash values) using a user-defined compression ratio. Signatures can then be compared against each other (some constrains apply) where the outcome is the estimated Levenshtein Distance. Our evaluation shows promising results in terms of runtime efficiency and accuracy. In addition, we introduce a significance score allowing examiners to set a threshold and identify related documents.
更多查看译文
关键词
document similarity,levenshtein distance,compression
AI 理解论文
溯源树
样例
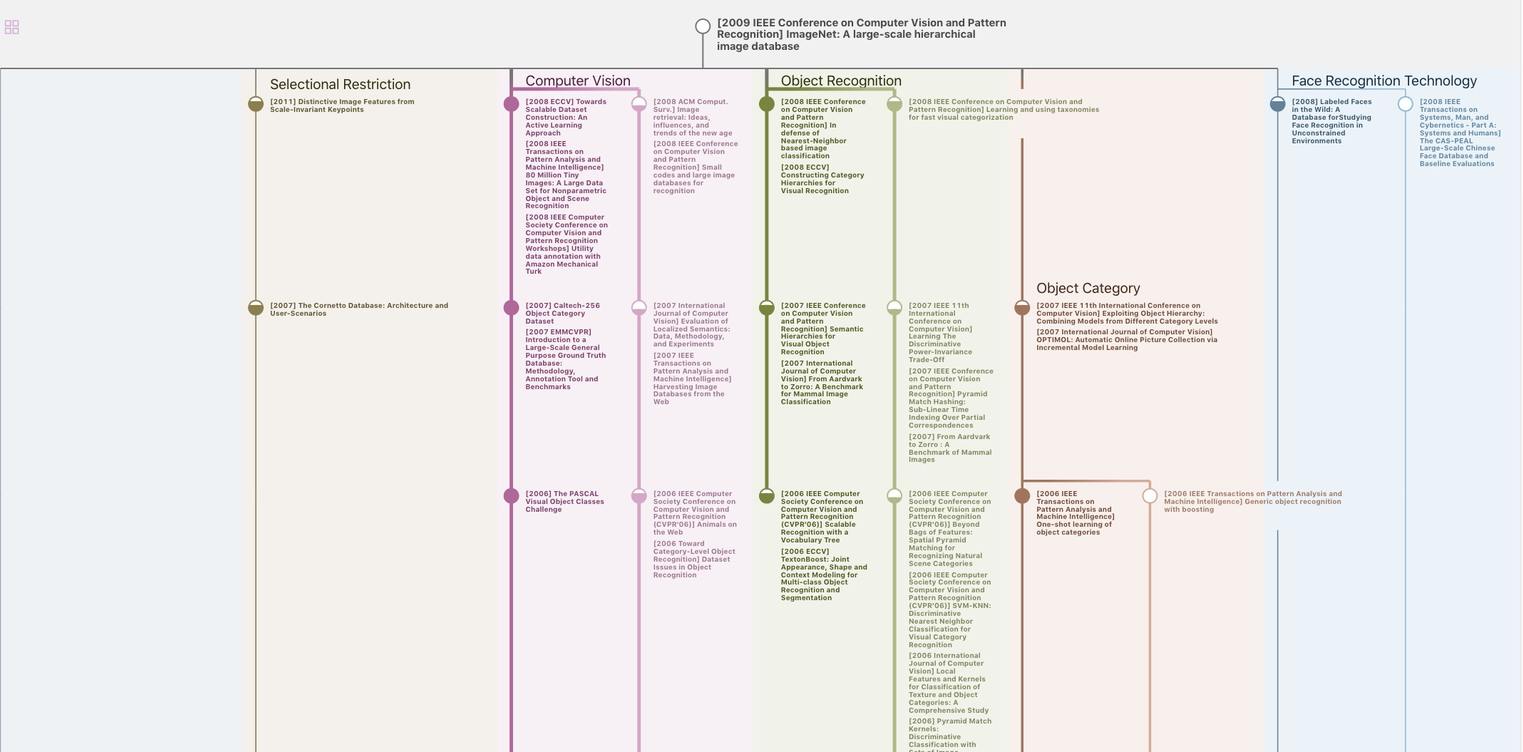
生成溯源树,研究论文发展脉络
Chat Paper
正在生成论文摘要