Neural Operators for Delay-Compensating Control of Hyperbolic PIDEs
CoRR(2023)
摘要
The recently introduced DeepONet operator-learning framework for PDE control is extended from the results for basic hyperbolic and parabolic PDEs to an advanced hyperbolic class that involves delays on both the state and the system output or input. The PDE backstepping design produces gain functions that are outputs of a nonlinear operator, mapping functions on a spatial domain into functions on a spatial domain, and where this gain-generating operator's inputs are the PDE's coefficients. The operator is approximated with a DeepONet neural network to a degree of accuracy that is provably arbitrarily tight. Once we produce this approximation-theoretic result in infinite dimension, with it we establish stability in closed loop under feedback that employs approximate gains. In addition to supplying such results under full-state feedback, we also develop DeepONet-approximated observers and output-feedback laws and prove their own stabilizing properties under neural operator approximations. With numerical simulations we illustrate the theoretical results and quantify the numerical effort savings, which are of two orders of magnitude, thanks to replacing the numerical PDE solving with the DeepONet.
更多查看译文
关键词
control,delay-compensating
AI 理解论文
溯源树
样例
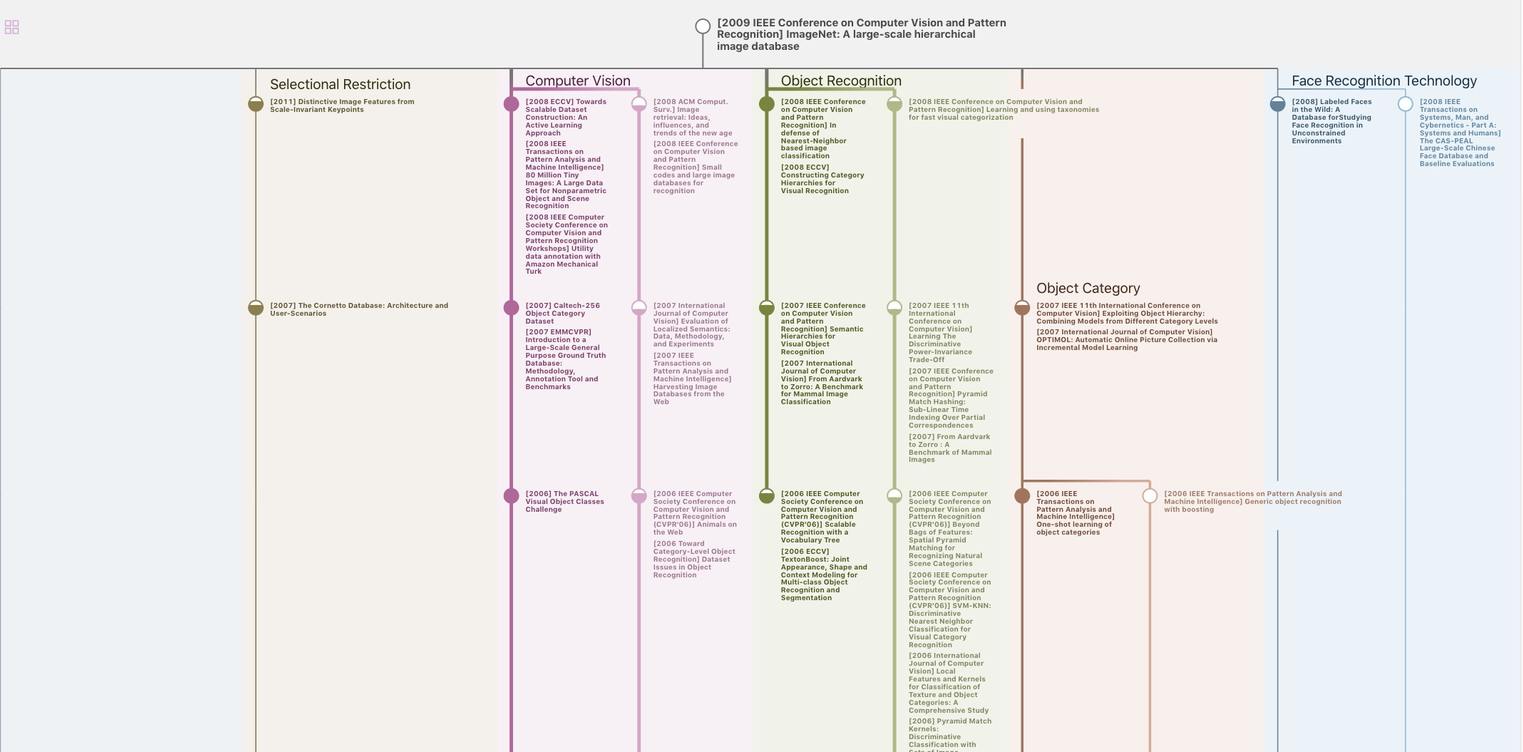
生成溯源树,研究论文发展脉络
Chat Paper
正在生成论文摘要