Clinical Trial Active Learning
14TH ACM CONFERENCE ON BIOINFORMATICS, COMPUTATIONAL BIOLOGY, AND HEALTH INFORMATICS, BCB 2023(2023)
摘要
This paper presents a novel approach to active learning that takes into account the non-independent and identically distributed (non-i.i.d.) structure of a clinical trial setting. There exists two types of clinical trials: retrospective and prospective. Retrospective clinical trials analyze data after treatment has been performed; prospective clinical trials collect data as treatment is ongoing. Typically, active learning approaches assume the dataset is i.i.d. when selecting training samples; however, in the case of clinical trials, treatment results in a dependency between the data collected at the current and past visits. Thus, we propose prospective active learning to overcome the limitations present in traditional active learning methods and apply it to disease detection in optical coherence tomography (OCT) images, where we condition on the time an image was collected to enforce the i.i.d. assumption. We compare our proposed method to the traditional active learning paradigm, which we refer to as retrospective in nature. We demonstrate that prospective active learning outperforms retrospective active learning in two different types of test settings.
更多查看译文
关键词
Active learning,Deep learning,Retinal disease detection,Prospective clinical trials
AI 理解论文
溯源树
样例
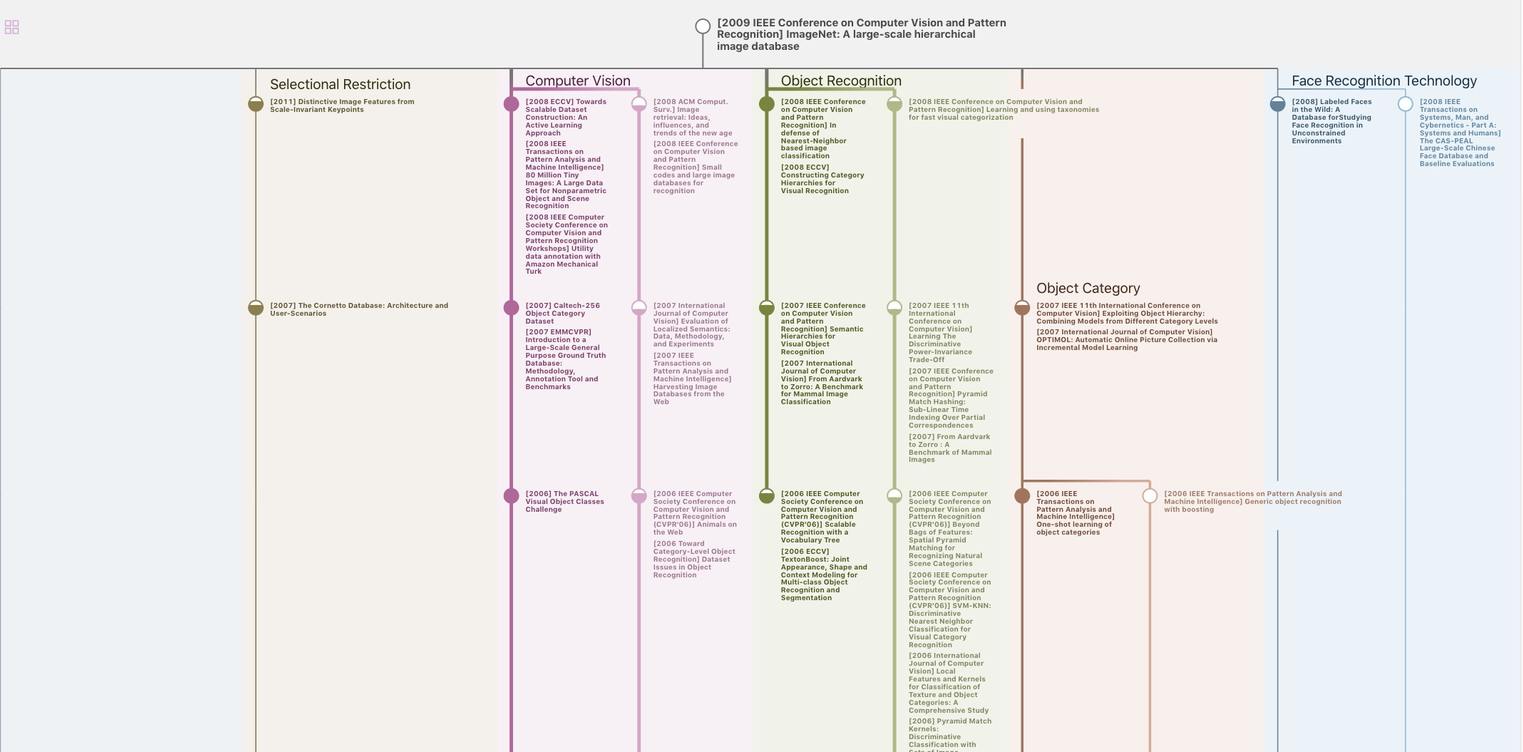
生成溯源树,研究论文发展脉络
Chat Paper
正在生成论文摘要