Non-invasive arterial blood pressure measurement and SpO 2 estimation using PPG signal: a deep learning framework
BMC medical informatics and decision making(2023)
摘要
Background Monitoring blood pressure and peripheral capillary oxygen saturation plays a crucial role in healthcare management for patients with chronic diseases, especially hypertension and vascular disease. However, current blood pressure measurement methods have intrinsic limitations; for instance, arterial blood pressure is measured by inserting a catheter in the artery causing discomfort and infection. Method Photoplethysmogram (PPG) signals can be collected via non-invasive devices, and therefore have stimulated researchers’ interest in exploring blood pressure estimation using machine learning and PPG signals as a non-invasive alternative. In this paper, we propose a Transformer-based deep learning architecture that utilizes PPG signals to conduct a personalized estimation of arterial systolic blood pressure, arterial diastolic blood pressure, and oxygen saturation. Results The proposed method was evaluated with a subset of 1,732 subjects from the publicly available ICU dataset MIMIC III. The mean absolute error is 2.52 ± 2.43 mmHg for systolic blood pressure, 1.37 ± 1.89 mmHg for diastolic blood pressure, and 0.58 ± 0.79% for oxygen saturation, which satisfies the requirements of the Association of Advancement of Medical Instrumentation standard and achieve grades A for the British Hypertension Society standard. Conclusions The results indicate that our model meets clinical standards and could potentially boost the accuracy of blood pressure and oxygen saturation measurement to deliver high-quality healthcare.
更多查看译文
关键词
Deep learning,Blood pressure,Photoplethysmogram,Oxygen saturation,Hypertension assessment,Digital health
AI 理解论文
溯源树
样例
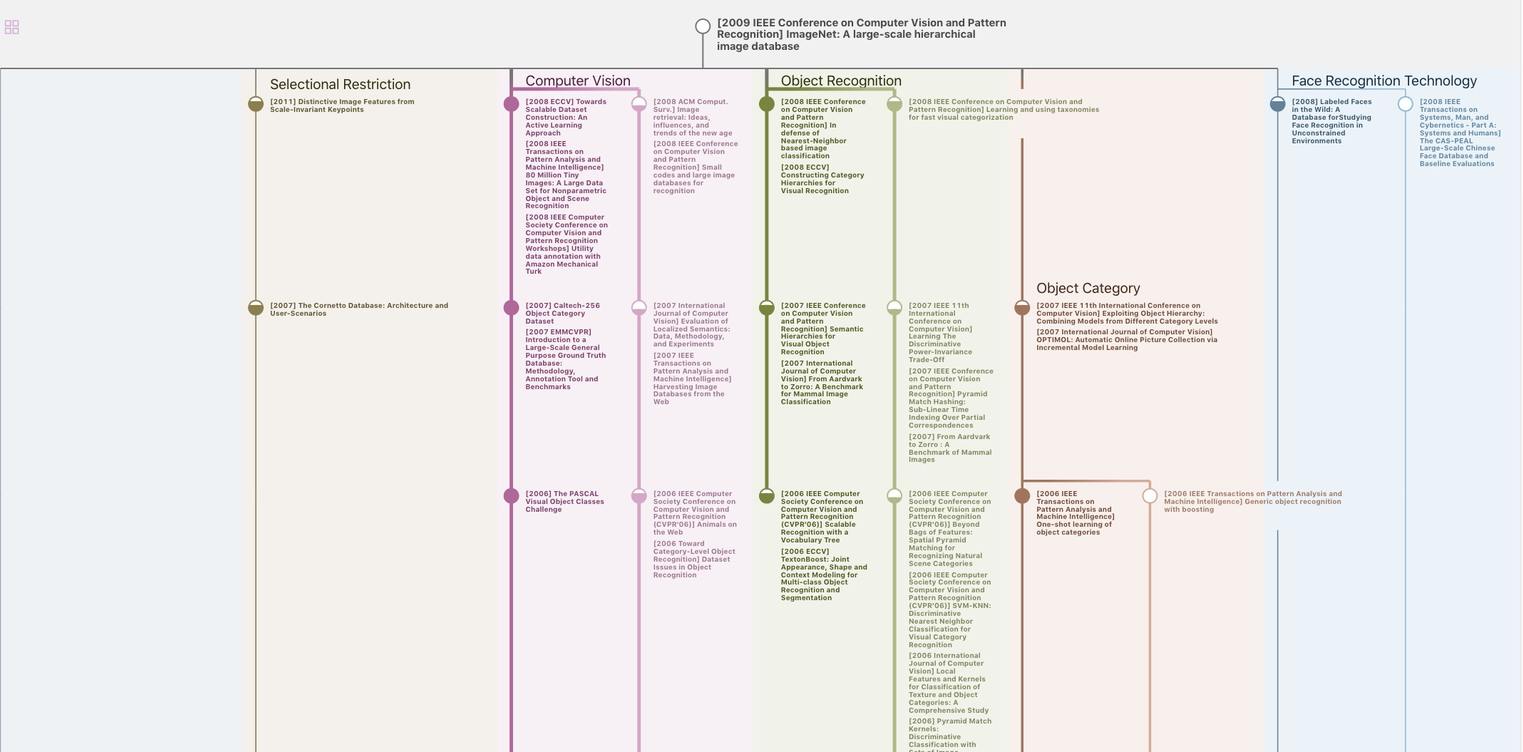
生成溯源树,研究论文发展脉络
Chat Paper
正在生成论文摘要