TinyBird-ML: An ultra-low Power Smart Sensor Node for Bird Vocalization Analysis and Syllable Classification
2023 IEEE International Symposium on Circuits and Systems (ISCAS)(2023)
摘要
Animal vocalisations serve a wide range of vital functions. Although it is possible to record animal vocalisations with external microphones, more insights are gained from miniature sensors mounted directly on animals' backs. We present TinyBird-ML; a wearable sensor node weighing only 1.4 g for acquiring, processing, and wirelessly transmitting acoustic signals to a host system using Bluetooth Low Energy. TinyBird-ML embeds low-latency tiny machine learning algorithms for song syllable classification. To optimize battery lifetime of TinyBird-ML during fault-tolerant continuous recordings, we present an efficient firmware and hardware design. We make use of standard lossy compression schemes to reduce the amount of data sent over the Bluetooth antenna, which increases battery lifetime by 70% without negative impact on offline sound analysis. Furthermore, by not transmitting signals during silent periods, we further increase battery lifetime. One advantage of our sensor is that it allows for closed-loop experiments in the microsecond range by processing sounds directly on the device instead of streaming them to a computer. We demonstrate this capability by detecting and classifying song syllables with minimal latency and a syllable error rate of 7%, using a light-weight neural network that runs directly on the sensor node itself. Thanks to our power-saving hardware and software design, during continuous operation at a sampling rate of 16 kHz, the sensor node achieves a lifetime of 25 hours on a single size 13 zinc-air battery.
更多查看译文
关键词
Wireless sensor node,low power,audio compression,machine learning,bird monitoring,bluetooth low energy
AI 理解论文
溯源树
样例
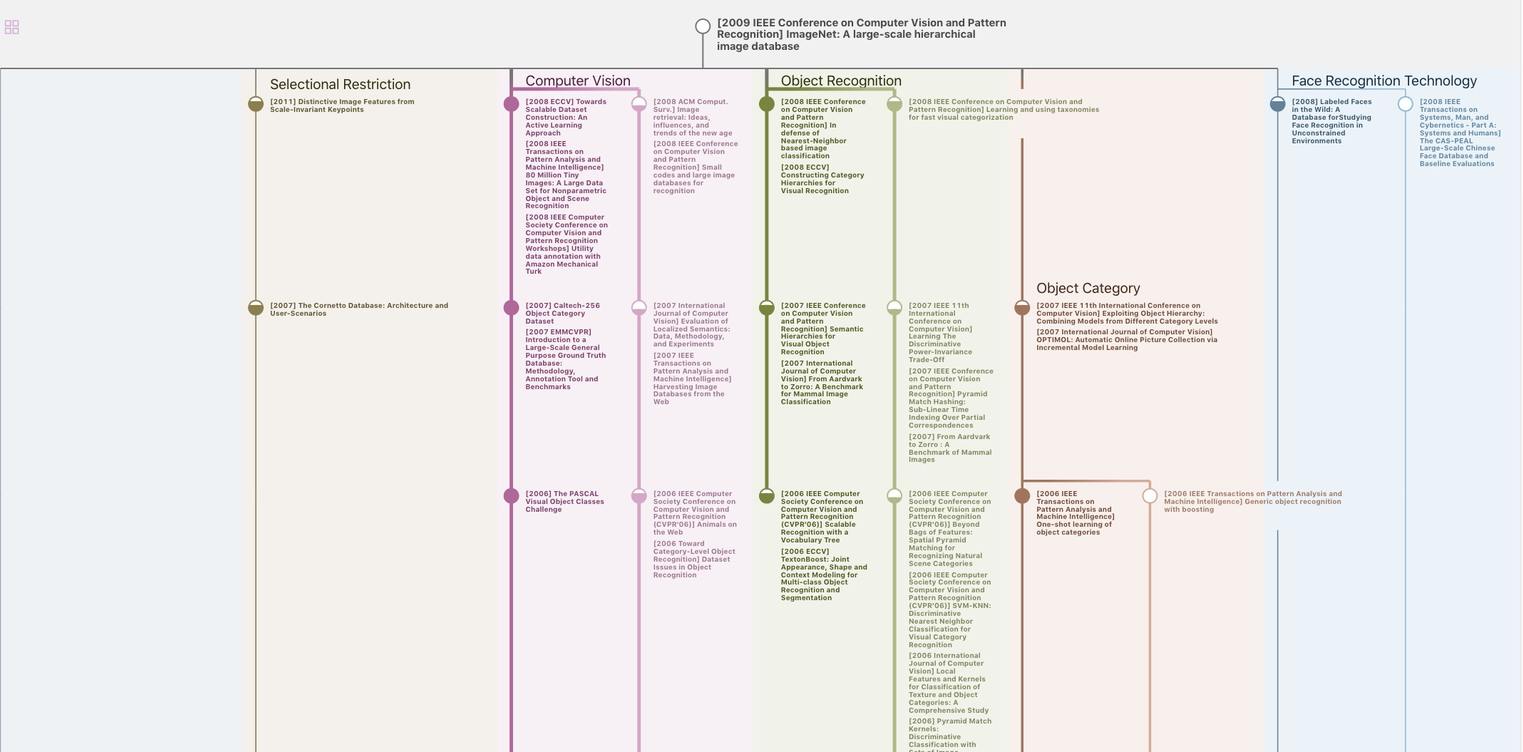
生成溯源树,研究论文发展脉络
Chat Paper
正在生成论文摘要