Transfer learning-based attenuation correction for static and dynamic cardiac PET using a generative adversarial network
European journal of nuclear medicine and molecular imaging(2023)
摘要
Purpose The goal of this work is to demonstrate the feasibility of directly generating attenuation-corrected PET images from non-attenuation-corrected (NAC) PET images for both rest and stress-state static or dynamic [ 13 N]ammonia MP PET based on a generative adversarial network. Methods We recruited 60 subjects for rest-only scans and 14 subjects for rest-stress scans, all of whom underwent [ 13 N]ammonia cardiac PET/CT examinations to acquire static and dynamic frames with both 3D NAC and CT-based AC (CTAC) PET images. We developed a 3D pix2pix deep learning AC (DLAC) framework via a U-net + ResNet-based generator and a convolutional neural network-based discriminator. Paired static or dynamic NAC and CTAC PET images from 60 rest-only subjects were used as network inputs and labels for static (S-DLAC) and dynamic (D-DLAC) training, respectively. The pre-trained S-DLAC network was then fine-tuned by paired dynamic NAC and CTAC PET frames of 60 rest-only subjects to derive an improved D-DLAC-FT for dynamic PET images. The 14 rest-stress subjects were used as an internal testing dataset and separately tested on different network models without training. The proposed methods were evaluated using visual quality and quantitative metrics. Results The proposed S-DLAC, D-DLAC, and D-DLAC-FT methods were consistent with clinical CTAC in terms of various images and quantitative metrics. The S-DLAC (slope = 0.9423, R 2 = 0.947) showed a higher correlation with the reference static CTAC as compared to static NAC (slope = 0.0992, R 2 = 0.654). D-DLAC-FT yielded lower myocardial blood flow (MBF) errors in the whole left ventricular myocardium than D-DLAC, but with no significant difference, both for the 60 rest-state subjects (6.63 ± 5.05% vs. 7.00 ± 6.84%, p = 0.7593) and the 14 stress-state subjects (1.97 ± 2.28% vs. 3.21 ± 3.89%, p = 0.8595). Conclusion The proposed S-DLAC, D-DLAC, and D-DLAC-FT methods achieve comparable performance with clinical CTAC. Transfer learning shows promising potential for dynamic MP PET.
更多查看译文
关键词
Deep learning, Attenuation correction, Myocardial perfusion PET, Myocardial blood flow, Transfer learning
AI 理解论文
溯源树
样例
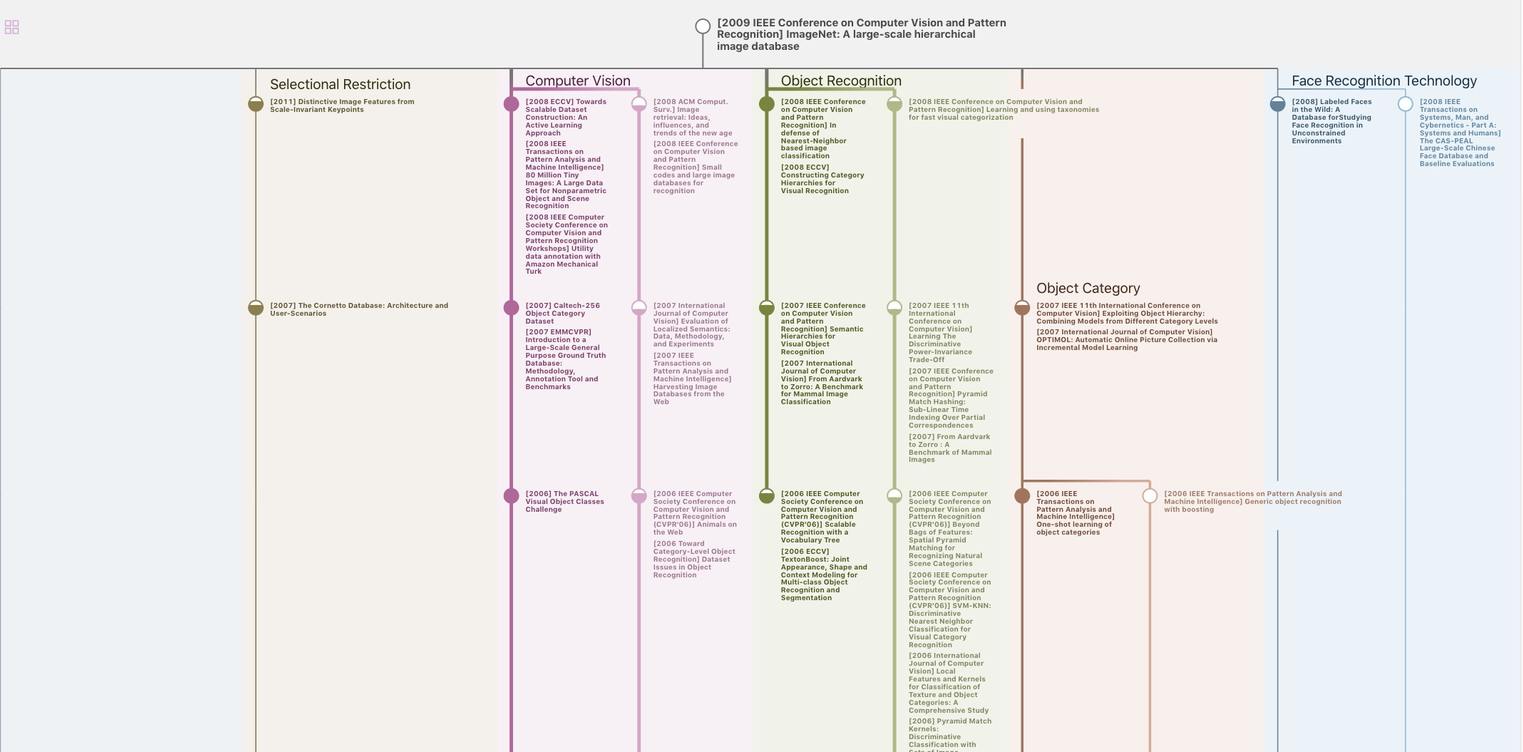
生成溯源树,研究论文发展脉络
Chat Paper
正在生成论文摘要