Beyond Supervised Learning for Pervasive Healthcare
IEEE REVIEWS IN BIOMEDICAL ENGINEERING(2024)
摘要
The integration of machine/deep learning and sensing technologies is transforming healthcare and medical practice. However, inherent limitations in healthcare data, namely scarcity, quality, and heterogeneity, hinder the effectiveness of supervised learning techniques which are mainly based on pure statistical fitting between data and labels. In this article, we first identify the challenges present in machine learning for pervasive healthcare and we then review the current trends beyond fully supervised learning that are developed to address these three issues. Rooted in the inherent drawbacks of empirical risk minimization that underpins pure fully supervised learning, this survey summarizes seven key lines of learning strategies, to promote the generalization performance for real-world deployment. In addition, we point out several directions that are emerging and promising in this area, to develop data-efficient, scalable, and trustworthy computational models, and to leverage multi-modality and multi-source sensing informatics, for pervasive healthcare.
更多查看译文
关键词
Pervasive sensing,healthcare,deep learning,machine learning,real-world applications
AI 理解论文
溯源树
样例
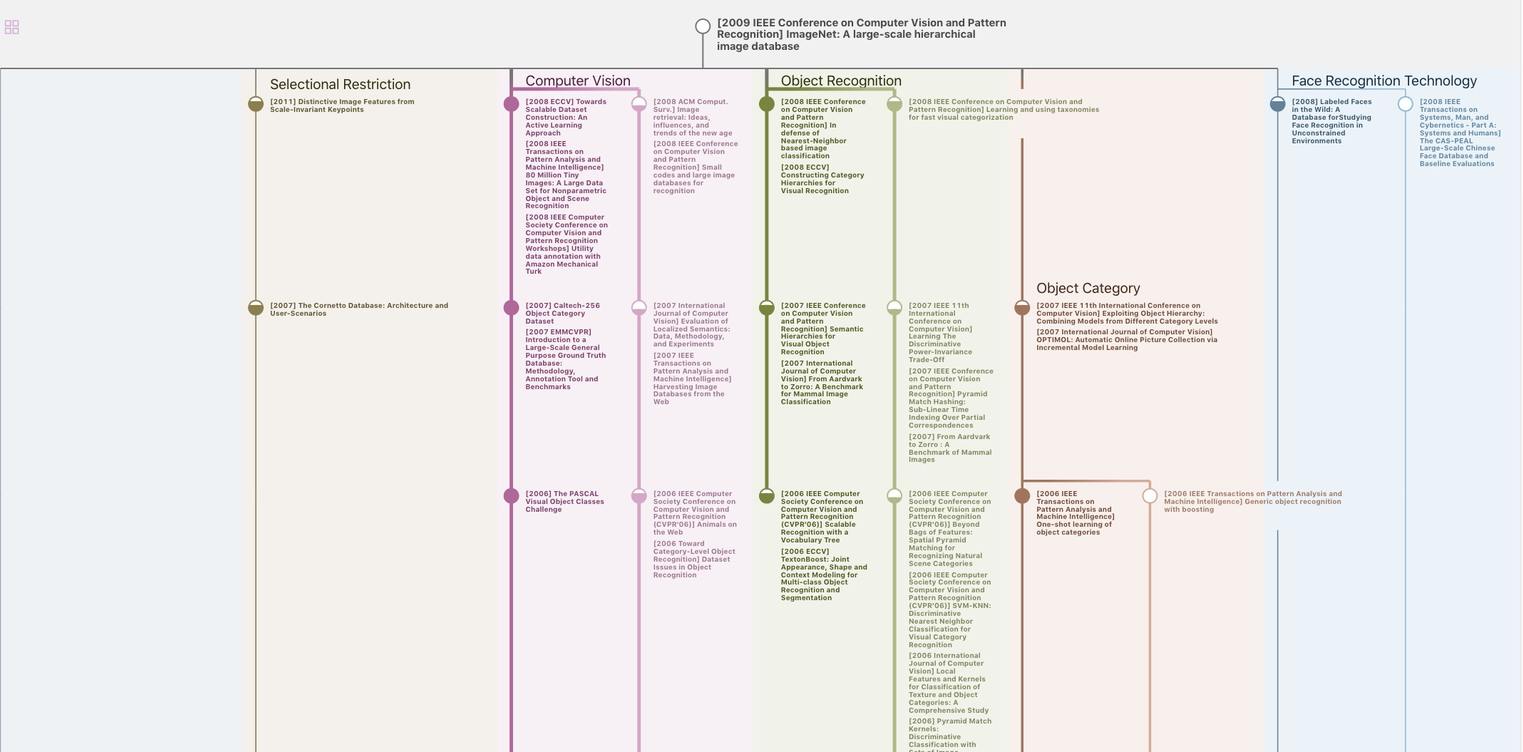
生成溯源树,研究论文发展脉络
Chat Paper
正在生成论文摘要