How to fit transfer models to learning data: a segmentation/clustering approach
Behavior research methods(2023)
摘要
Although transfer models are limited in their ability to evolve over time and account for a wide range of processes, they have repeatedly shown to be useful for testing categorization theories and predicting participants’ generalization performance. In this study, we propose a statistical framework that allows transfer models to be applied to category learning data. Our framework uses a segmentation/clustering technique specifically tailored to suit category learning data. We applied this technique to a well-known transfer model, the Generalized Context Model, in three novel experiments that manipulated ordinal effects in category learning. The difference in performance across the three contexts, as well as the benefit of the rule-based order observed in two out of three experiments, were mostly detected by the segmentation/clustering method. Furthermore, the analysis of the segmentation/clustering outputs using the backward learning curve revealed that participants’ performance suddenly improved, suggesting the detection of an “eureka” moment. Our adjusted segmentation/clustering framework allows transfer models to fit learning data while capturing relevant patterns.
更多查看译文
关键词
Categorization,Segmentation/Clustering,Category transfer models,Generalized Context Model (GCM),Rule-based versus similarity-based presentation order
AI 理解论文
溯源树
样例
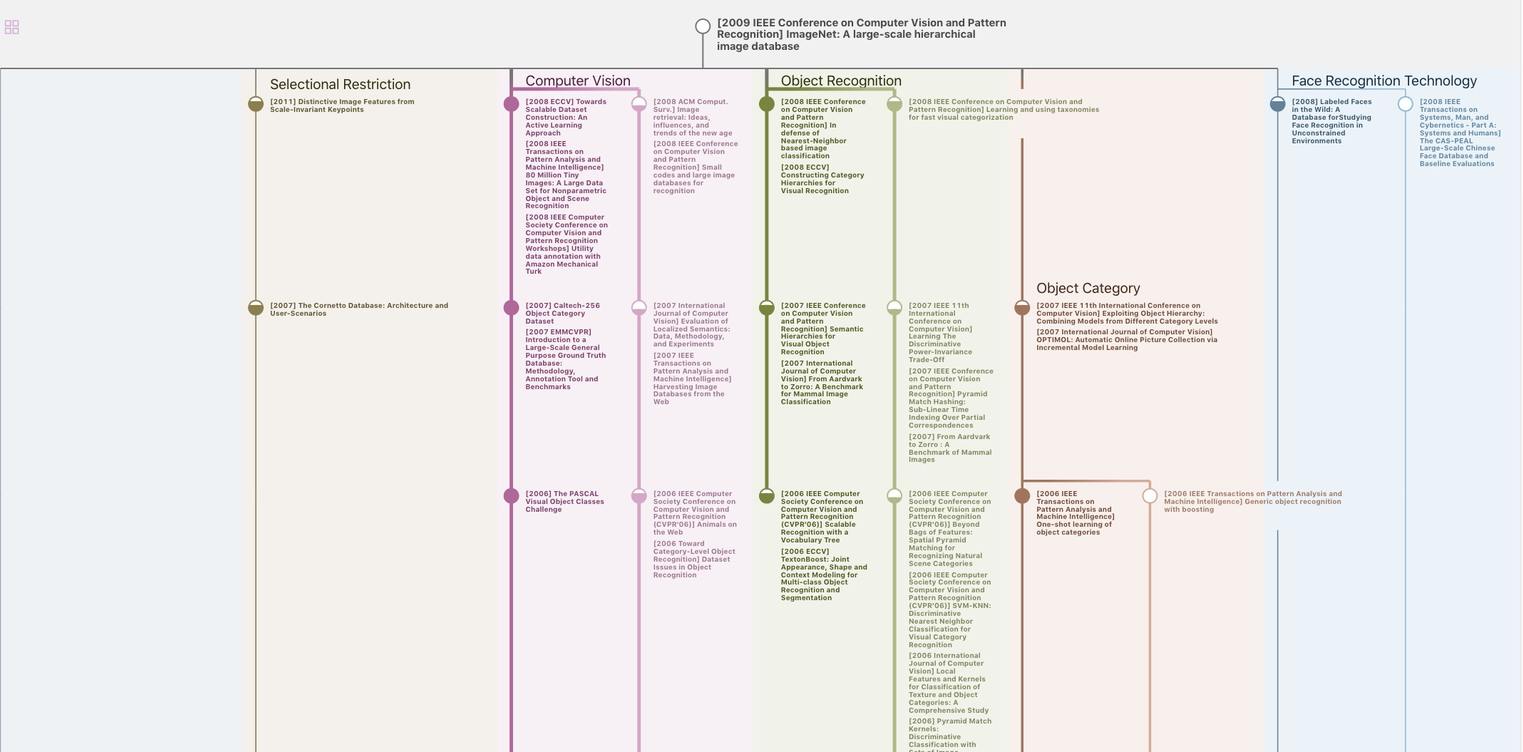
生成溯源树,研究论文发展脉络
Chat Paper
正在生成论文摘要