A Clustering Algorithm for Polygonal Data Applied to Scientific Journal Profiles.
IEEE transactions on pattern analysis and machine intelligence(2023)
摘要
Millions of papers are submitted and published every year, but researchers often do not have much information about the journals that interest them. In this paper, we introduced the first dynamical clustering algorithm for symbolic polygonal data and this was applied to build scientific journals profiles. Dynamic clustering algorithms are a family of iterative two-step relocation algorithms involving the construction of clusters at each iteration and the identification of a suitable representation or prototype (means, axes, probability laws, groups of elements, etc.) for each cluster by locally optimizing an adequacy criterion that measures the fitting between clusters and their corresponding prototypes The application gives a powerful vision to understand the main variables that describe journals. Symbolic polygonal data can represent summarized extensive datasets taking into account variability. In addition, we developed cluster and partition interpretation indices for polygonal data that have the ability to extract insights about clustering results. From these indices, we discovered, e.g., that the number of difficult words in abstract is fundamental to building journal profiles.
更多查看译文
关键词
scientific journal profiles,clustering algorithm,polygonal data
AI 理解论文
溯源树
样例
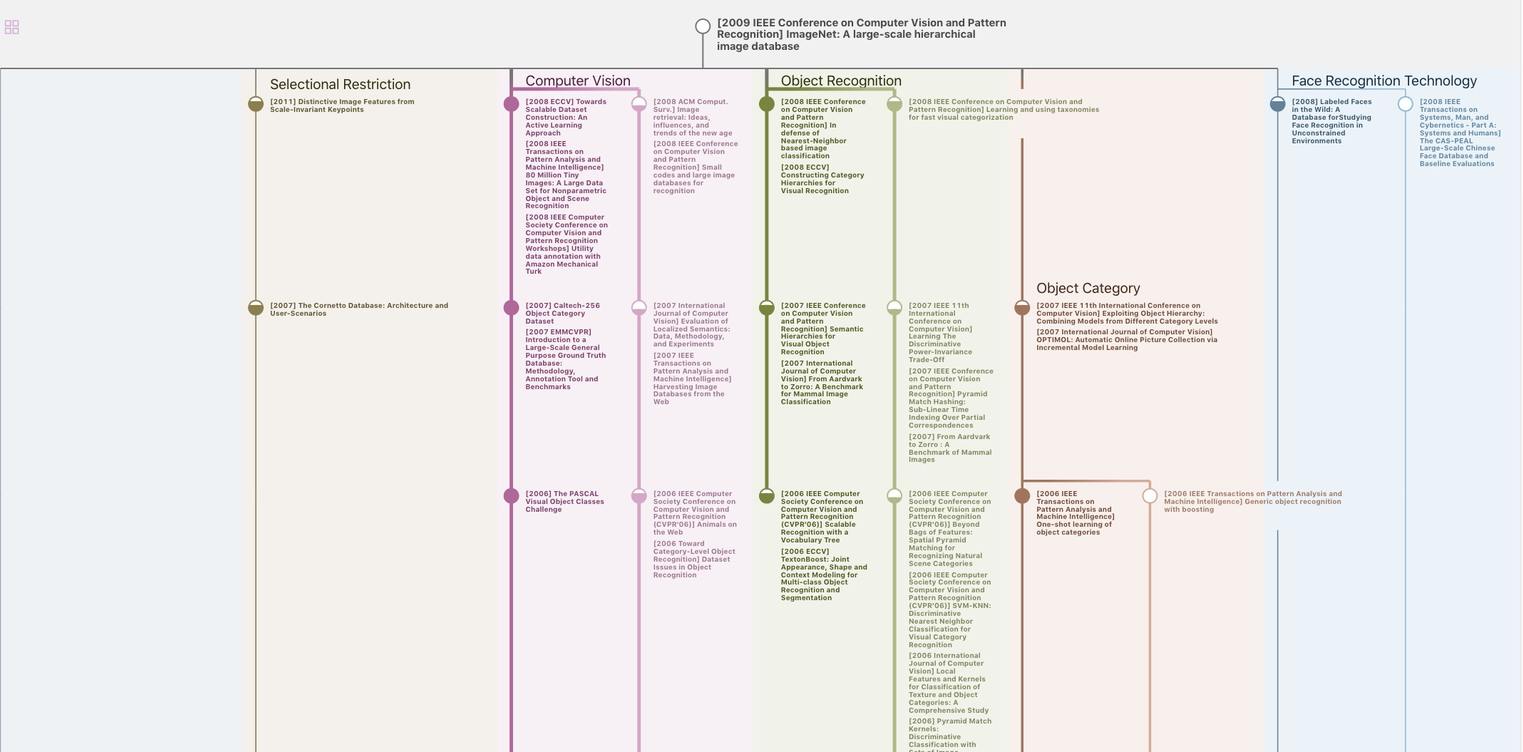
生成溯源树,研究论文发展脉络
Chat Paper
正在生成论文摘要