Sharpness Minimization Algorithms Do Not Only Minimize Sharpness To Achieve Better Generalization
NeurIPS(2023)
摘要
Despite extensive studies, the underlying reason as to why overparameterized neural networks can generalize remains elusive. Existing theory shows that common stochastic optimizers prefer flatter minimizers of the training loss, and thus a natural potential explanation is that flatness implies generalization. This work critically examines this explanation. Through theoretical and empirical investigation, we identify the following three scenarios for two-layer ReLU networks: (1) flatness provably implies generalization; (2) there exist non-generalizing flattest models and sharpness minimization algorithms fail to generalize, and (3) perhaps most surprisingly, there exist non-generalizing flattest models, but sharpness minimization algorithms still generalize. Our results suggest that the relationship between sharpness and generalization subtly depends on the data distributions and the model architectures and sharpness minimization algorithms do not only minimize sharpness to achieve better generalization. This calls for the search for other explanations for the generalization of over-parameterized neural networks.
更多查看译文
关键词
sharpness minimization algorithms,better generalization
AI 理解论文
溯源树
样例
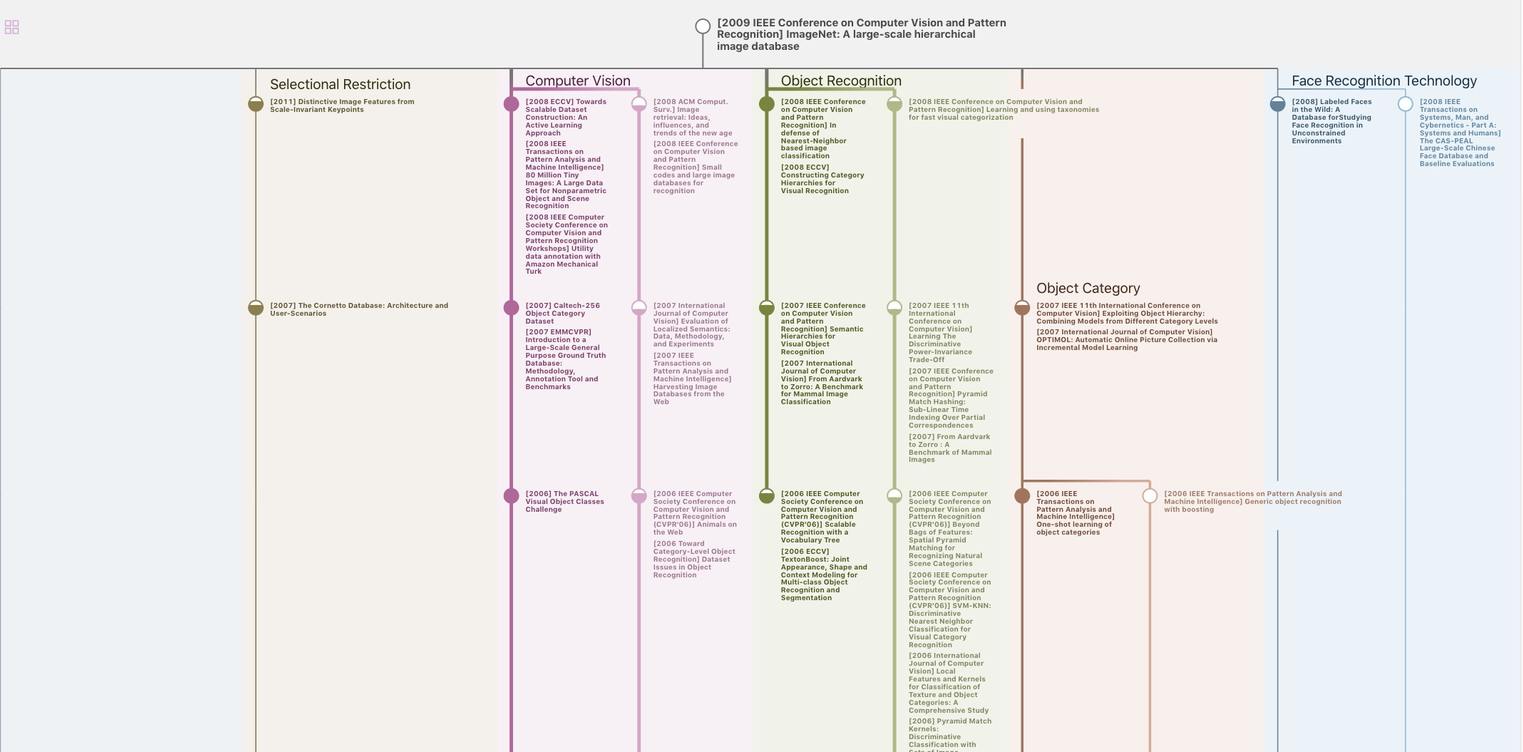
生成溯源树,研究论文发展脉络
Chat Paper
正在生成论文摘要