Risk-optimized Outlier Removal for Robust 3D Point Cloud Classification
CoRR(2023)
摘要
With the growth of 3D sensing technology, deep learning system for 3D point
clouds has become increasingly important, especially in applications like
autonomous vehicles where safety is a primary concern. However, there are also
growing concerns about the reliability of these systems when they encounter
noisy point clouds, whether occurring naturally or introduced with malicious
intent. This paper highlights the challenges of point cloud classification
posed by various forms of noise, from simple background noise to malicious
backdoor attacks that can intentionally skew model predictions. While there's
an urgent need for optimized point cloud denoising, current point outlier
removal approaches, an essential step for denoising, rely heavily on
handcrafted strategies and are not adapted for higher-level tasks, such as
classification. To address this issue, we introduce an innovative point outlier
cleansing method that harnesses the power of downstream classification models.
By employing gradient-based attribution analysis, we define a novel concept:
point risk. Drawing inspiration from tail risk minimization in finance, we
recast the outlier removal process as an optimization problem, named PointCVaR.
Extensive experiments show that our proposed technique not only robustly
filters diverse point cloud outliers but also consistently and significantly
enhances existing robust methods for point cloud classification.
更多查看译文
关键词
outlier removal,classification,cloud,risk-optimized
AI 理解论文
溯源树
样例
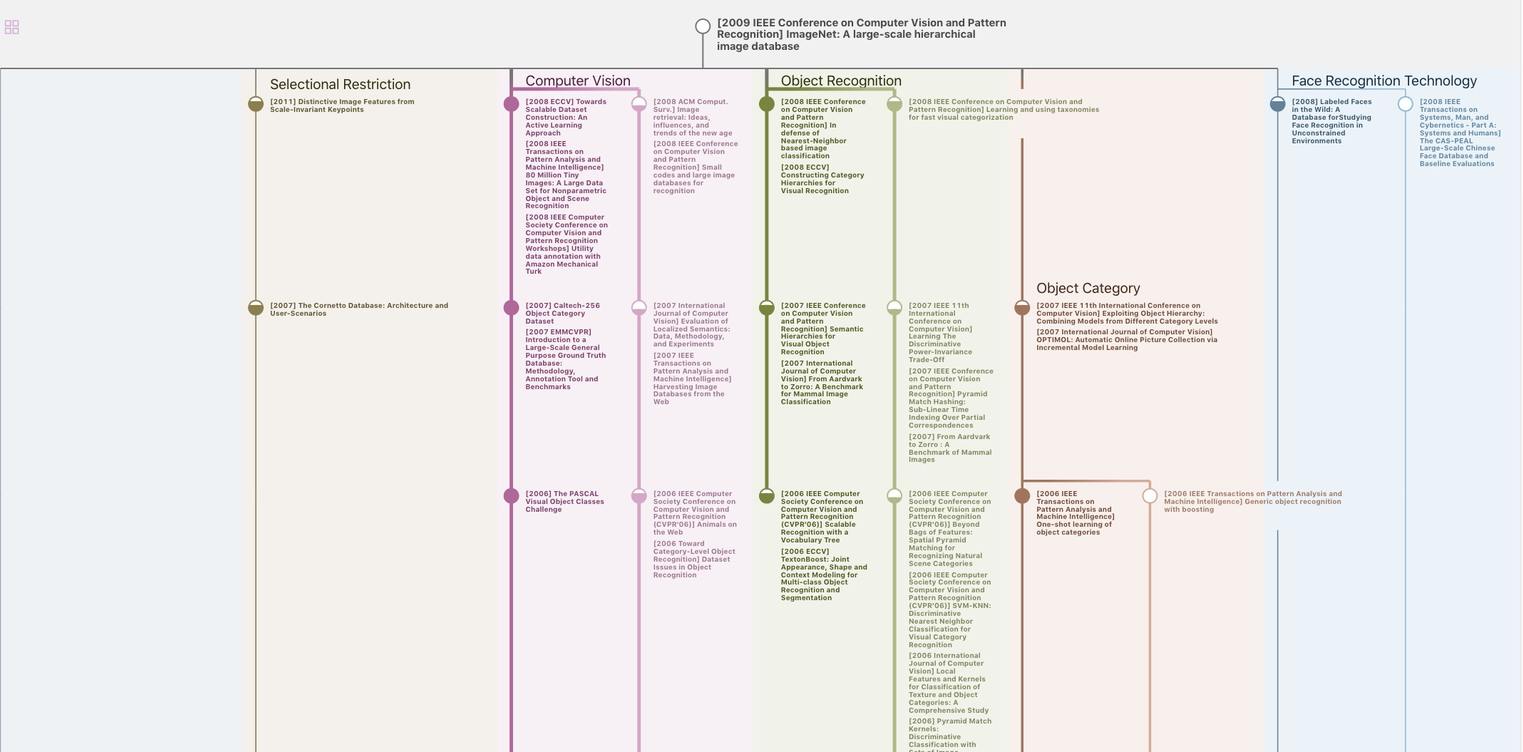
生成溯源树,研究论文发展脉络
Chat Paper
正在生成论文摘要