Addressing caveats of neural persistence with deep graph persistence
CoRR(2023)
摘要
Neural Persistence is a prominent measure for quantifying neural network complexity, proposed in the emerging field of topological data analysis in deep learning. In this work, however, we find both theoretically and empirically that the variance of network weights and spatial concentration of large weights are the main factors that impact neural persistence. Whilst this captures useful information for linear classifiers, we find that no relevant spatial structure is present in later layers of deep neural networks, making neural persistence roughly equivalent to the variance of weights. Additionally, the proposed averaging procedure across layers for deep neural networks does not consider interaction between layers. Based on our analysis, we propose an extension of the filtration underlying neural persistence to the whole neural network instead of single layers, which is equivalent to calculating neural persistence on one particular matrix. This yields our deep graph persistence measure, which implicitly incorporates persistent paths through the network and alleviates variance-related issues through standardisation. Code is available at https://github.com/ExplainableML/Deep-Graph-Persistence .
更多查看译文
关键词
neural persistence,deep,graph
AI 理解论文
溯源树
样例
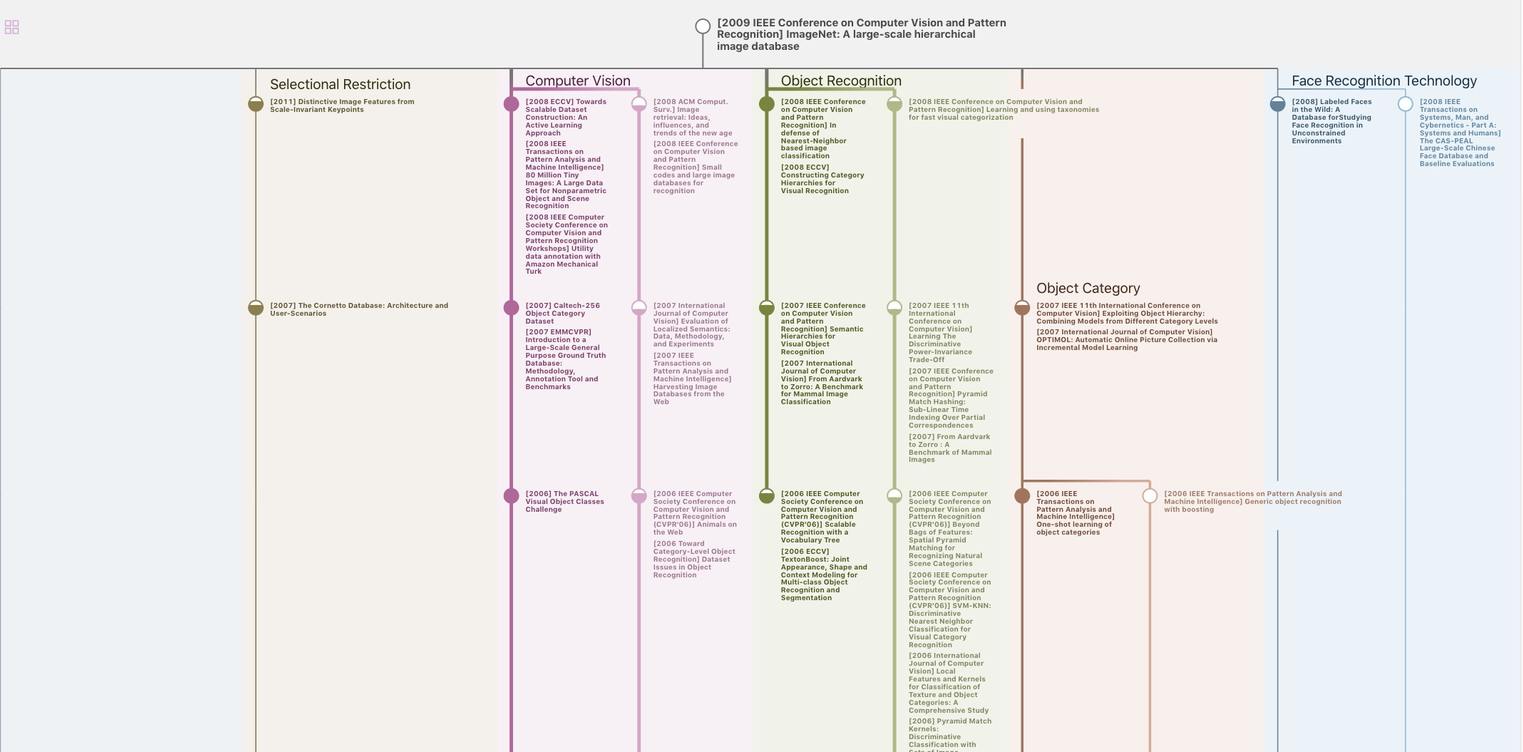
生成溯源树,研究论文发展脉络
Chat Paper
正在生成论文摘要