Reparameterized Policy Learning for Multimodal Trajectory Optimization
ICML 2023(2023)
摘要
We investigate the challenge of parametrizing policies for reinforcement learning (RL) in high-dimensional continuous action spaces. Our objective is to develop a multimodal policy that overcomes limitations inherent in the commonly-used Gaussian parameterization. To achieve this, we propose a principled framework that models the continuous RL policy as a generative model of optimal trajectories. By conditioning the policy on a latent variable, we derive a novel variational bound as the optimization objective, which promotes exploration of the environment. We then present a practical model-based RL method, called Reparameterized Policy Gradient (RPG), which leverages the multimodal policy parameterization and learned world model to achieve strong exploration capabilities and high data efficiency. Empirical results demonstrate that our method can help agents evade local optima in tasks with dense rewards and solve challenging sparse-reward environments by incorporating an object-centric intrinsic reward. Our method consistently outperforms previous approaches across a range of tasks. Code and supplementary materials are available on the project page https://haosulab.github.io/RPG/
更多查看译文
关键词
policy learning,optimization
AI 理解论文
溯源树
样例
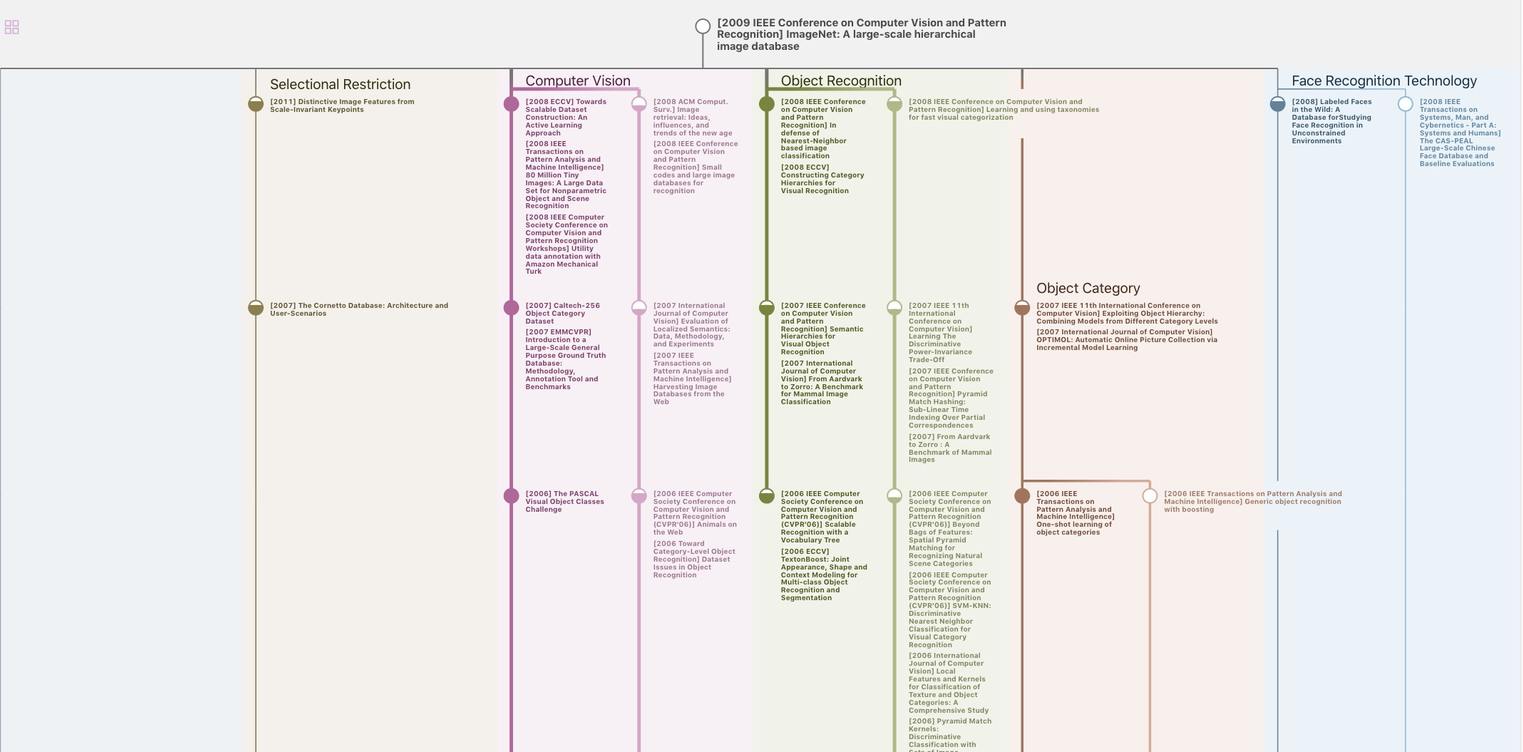
生成溯源树,研究论文发展脉络
Chat Paper
正在生成论文摘要