Heterogeneous Federated Learning: State-of-the-art and Research Challenges
ACM COMPUTING SURVEYS(2024)
摘要
Federated learning (FL) has drawn increasing attention owing to its potential use in large-scale industrial applications. Existing FL works mainly focus on model homogeneous settings. However, practical FL typically faces the heterogeneity of data distributions, model architectures, network environments, and hardware devices among participant clients. Heterogeneous Federated Learning (HFL) is much more challenging, and corresponding solutions are diverse and complex. Therefore, a systematic survey on this topic about the research challenges and state-of-the-art is essential. In this survey, we firstly summarize the various research challenges in HFL from five aspects: statistical heterogeneity, model heterogeneity, communication heterogeneity, device heterogeneity, and additional challenges. In addition, recent advances in HFL are reviewed and a new taxonomy of existing HFL methods is proposed with an in-depth analysis of their pros and cons. We classify existing methods from three different levels according to the HFL procedure: data-level, model-level, and server-level. Finally, several critical and promising future research directions in HFL are discussed, which may facilitate further developments in this field. A periodically updated collection on HFL is available at https://github.com/marswhu/HFL_Survey.
更多查看译文
关键词
Survey,federated learning,trustworthy AI
AI 理解论文
溯源树
样例
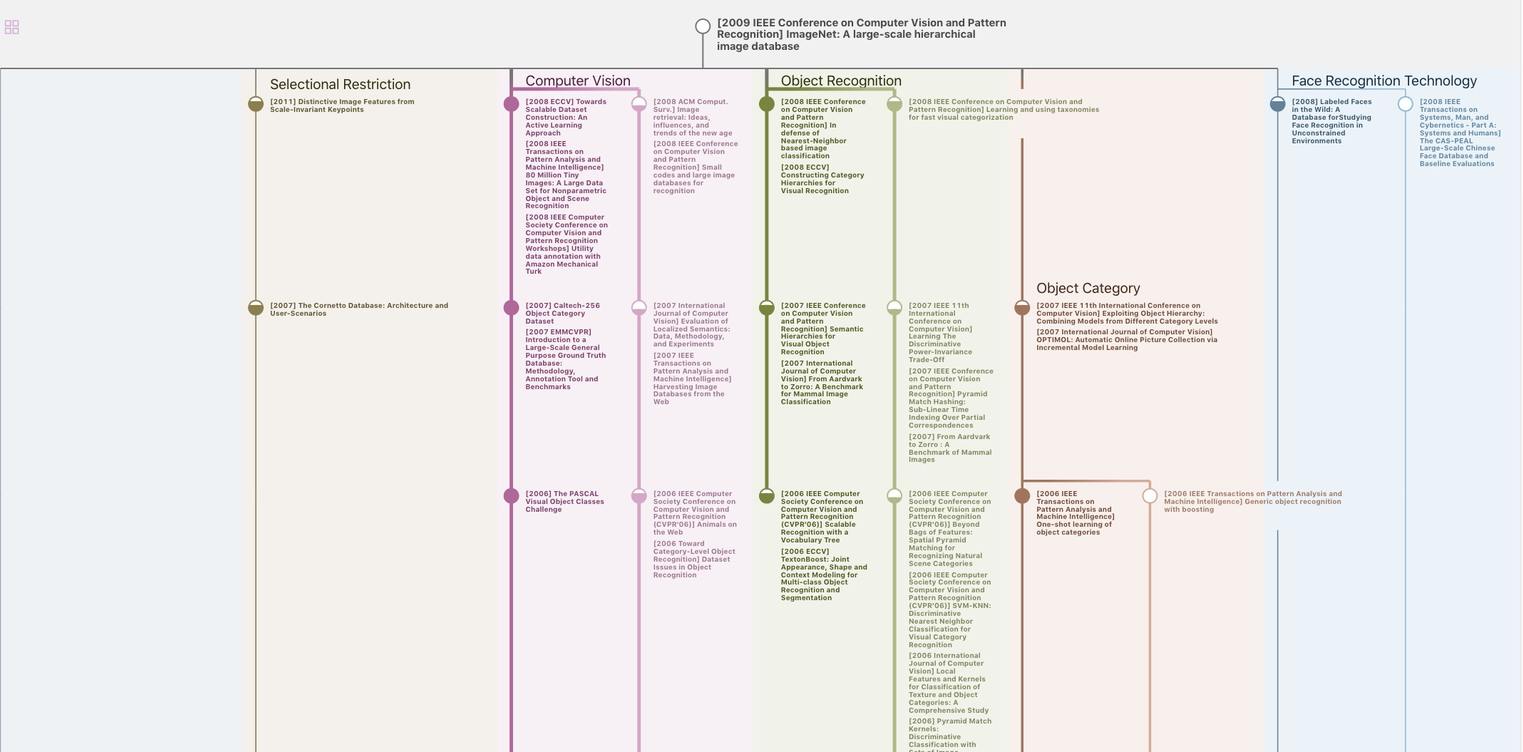
生成溯源树,研究论文发展脉络
Chat Paper
正在生成论文摘要