TokenFlow: Consistent Diffusion Features for Consistent Video Editing
ICLR 2024(2023)
摘要
The generative AI revolution has recently expanded to videos. Nevertheless, current state-of-the-art video models are still lagging behind image models in terms of visual quality and user control over the generated content. In this work, we present a framework that harnesses the power of a text-to-image diffusion model for the task of text-driven video editing. Specifically, given a source video and a target text-prompt, our method generates a high-quality video that adheres to the target text, while preserving the spatial layout and motion of the input video. Our method is based on a key observation that consistency in the edited video can be obtained by enforcing consistency in the diffusion feature space. We achieve this by explicitly propagating diffusion features based on inter-frame correspondences, readily available in the model. Thus, our framework does not require any training or fine-tuning, and can work in conjunction with any off-the-shelf text-to-image editing method. We demonstrate state-of-the-art editing results on a variety of real-world videos. Webpage: https://diffusion-tokenflow.github.io/
更多查看译文
关键词
Computer Vision Applications,Video Editing and Synthesis,Text-to-Image Diffusion Models,Text-driven Video Editing
AI 理解论文
溯源树
样例
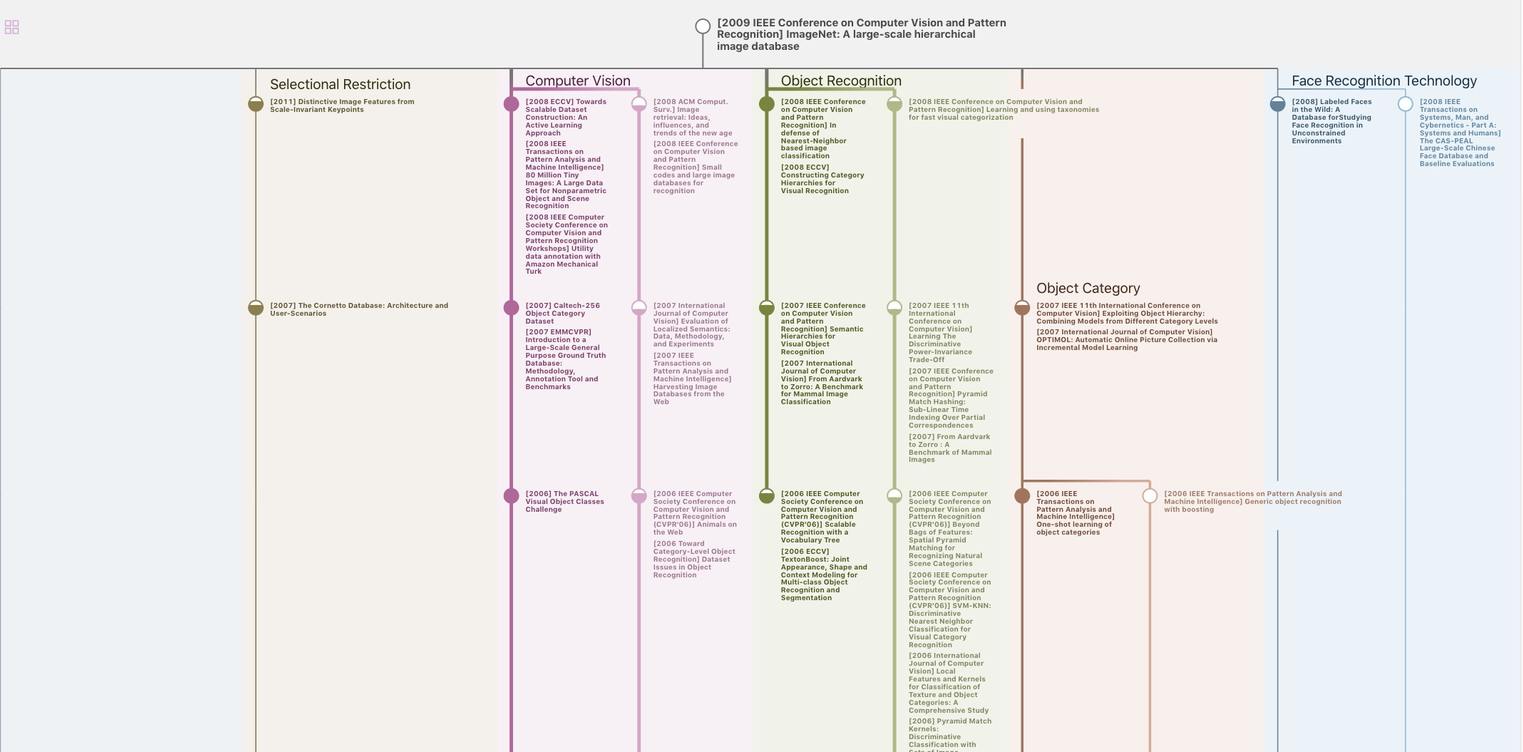
生成溯源树,研究论文发展脉络
Chat Paper
正在生成论文摘要