A General Dual-Branch Framework for Land Cover Mapping Models With Multispectral Data
IEEE Geoscience and Remote Sensing Letters(2023)
摘要
Land cover mapping based on multispectral images can, in principle, be considered an application of semantic segmentation, but land cover mapping inputs include near-infrared (NIR) data in addition to RGB data. It has been experimentally found that LULC mapping performance based on RGB data alone is better than that based on RGB and NIR data when using some established single-branch encoder–decoder models. To address this issue, we propose a dual-branch encoder–decoder (DBED) framework that can be applied to existing encoder–decoder models for semantic segmentation. First, the multispectral input data is divided into two parts: RGB and NIR and fed to the respective branch for encoding. The dual-branch structure facilitates cross-modal complementary information encoding without deteriorating the original RGB modality-specific feature extraction. Second, an attention module named the multispectral attention module (MSAM) is proposed to mine the contextual correlation between the multispectral feature maps, leading to further performance boosting. We apply this framework to three mainstream semantic segmentation models and validate it on the Gaofen Image Dataset (GID). Experimental results show that this structure brings performance improvements. The source code of DBED is publicly available at
https://github.com/MalignusCN/DBED
.
更多查看译文
关键词
land cover mapping models,dual-branch,multi-spectral
AI 理解论文
溯源树
样例
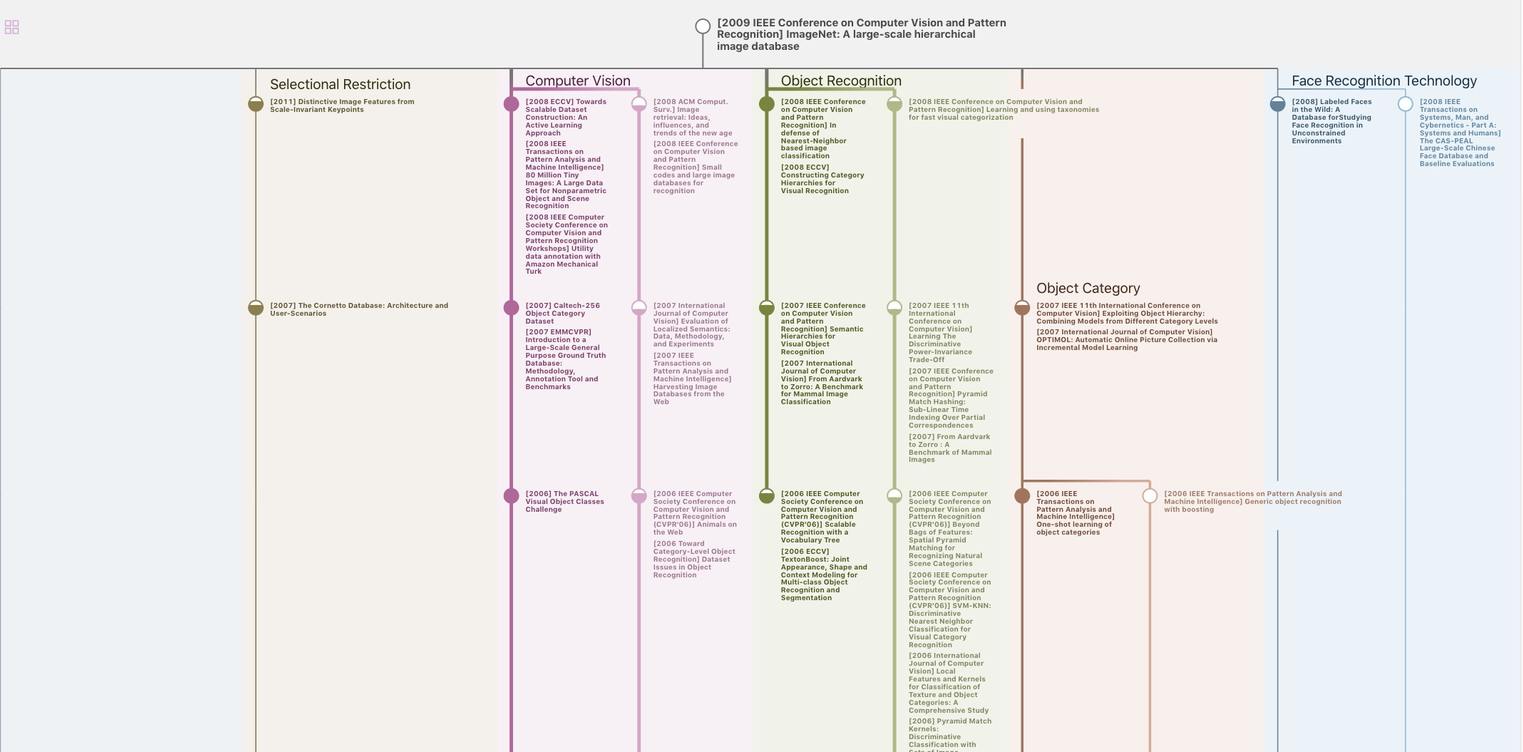
生成溯源树,研究论文发展脉络
Chat Paper
正在生成论文摘要