Lindorm TSDB: A Cloud-native Time-series Database for Large-scale Monitoring Systems
VLDB 2023(2023)
摘要
Internet services supported by large-scale distributed systems have become essential for our daily life. To ensure the stability and high quality of services, diverse metric data are constantly collected and managed in a time-series database to monitor the service status. However, when the number of metrics becomes massive, existing time-series databases are inefficient in handling high-rate data ingestion and queries hitting multiple metrics. Besides, they all lack the support of machine learning functions, which are crucial for sophisticated analysis of large-scale time series. In this paper, we present Lindorm TSDB, a distributed time-series database designed for handling monitoring metrics at scale. It sustains high write throughput and low query latency with massive active metrics. It also allows users to analyze data with anomaly detection and time series forecasting algorithms directly through SQL. Furthermore, Lindorm TSDB retains stable performance even during node scaling. We evaluate Lindorm TSDB under different data scales, and the results show that it outperforms two popular open-source time-series databases on both writing and query, while executing time-series machine learning tasks efficiently.
更多查看译文
关键词
monitoring,lindorm tsdb,cloud-native,time-series,large-scale
AI 理解论文
溯源树
样例
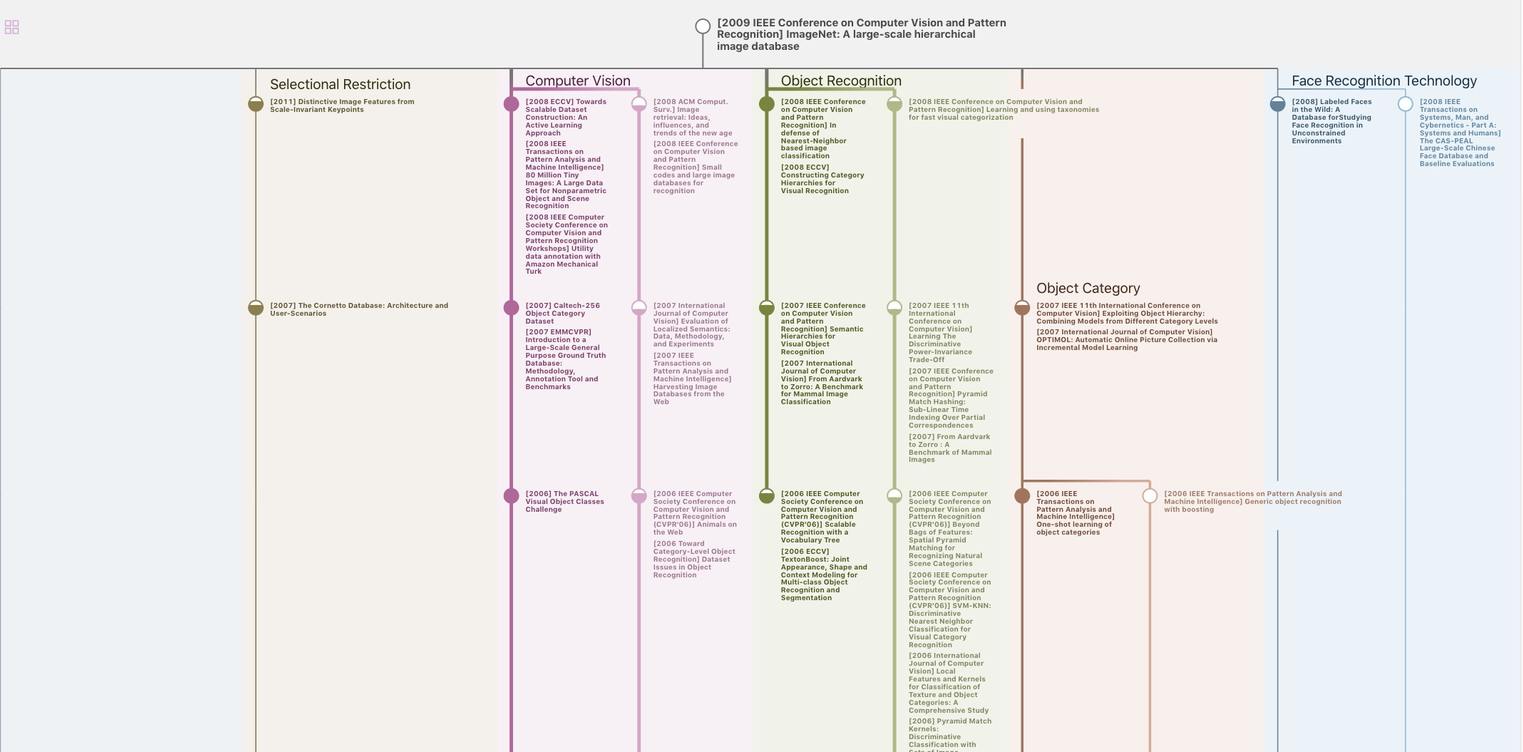
生成溯源树,研究论文发展脉络
Chat Paper
正在生成论文摘要