U-CE: Uncertainty-aware Cross-Entropy for Semantic Segmentation
CoRR(2023)
摘要
Deep neural networks have shown exceptional performance in various tasks, but their lack of robustness, reliability, and tendency to be overconfident pose challenges for their deployment in safety-critical applications like autonomous driving. In this regard, quantifying the uncertainty inherent to a model's prediction is a promising endeavour to address these shortcomings. In this work, we present a novel Uncertainty-aware Cross-Entropy loss (U-CE) that incorporates dynamic predictive uncertainties into the training process by pixel-wise weighting of the well-known cross-entropy loss (CE). Through extensive experimentation, we demonstrate the superiority of U-CE over regular CE training on two benchmark datasets, Cityscapes and ACDC, using two common backbone architectures, ResNet-18 and ResNet-101. With U-CE, we manage to train models that not only improve their segmentation performance but also provide meaningful uncertainties after training. Consequently, we contribute to the development of more robust and reliable segmentation models, ultimately advancing the state-of-the-art in safety-critical applications and beyond.
更多查看译文
关键词
semantic segmentation,uncertainty-aware,cross-entropy
AI 理解论文
溯源树
样例
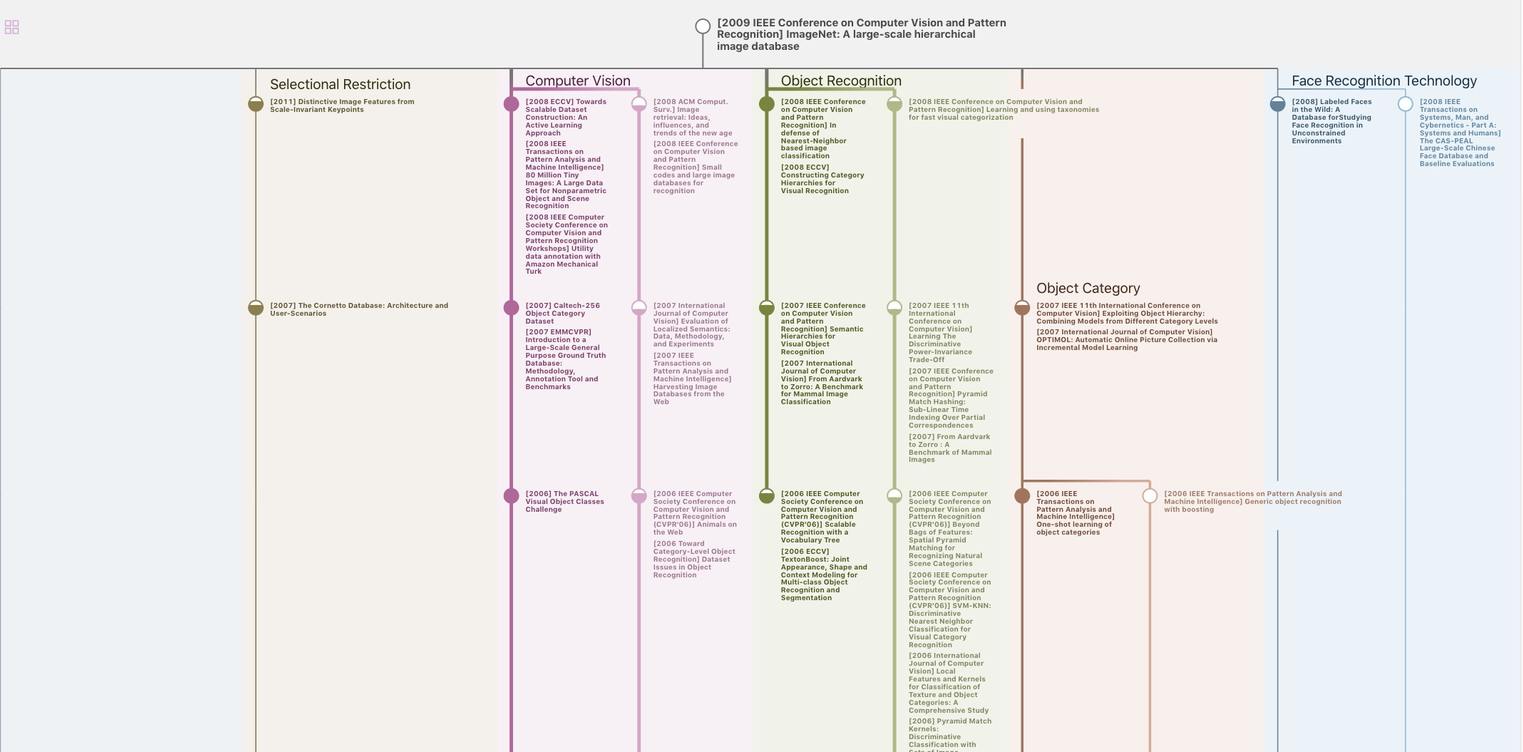
生成溯源树,研究论文发展脉络
Chat Paper
正在生成论文摘要