Learning from Abstract Images: on the Importance of Occlusion in a Minimalist Encoding of Human Poses
CoRR(2023)
摘要
Existing 2D-to-3D pose lifting networks suffer from poor performance in cross-dataset benchmarks. Although the use of 2D keypoints joined by "stick-figure" limbs has shown promise as an intermediate step, stick-figures do not account for occlusion information that is often inherent in an image. In this paper, we propose a novel representation using opaque 3D limbs that preserves occlusion information while implicitly encoding joint locations. Crucially, when training on data with accurate three-dimensional keypoints and without part-maps, this representation allows training on abstract synthetic images, with occlusion, from as many synthetic viewpoints as desired. The result is a pose defined by limb angles rather than joint positions $\unicode{x2013}$ because poses are, in the real world, independent of cameras $\unicode{x2013}$ allowing us to predict poses that are completely independent of camera viewpoint. The result provides not only an improvement in same-dataset benchmarks, but a "quantum leap" in cross-dataset benchmarks.
更多查看译文
关键词
abstract images,occlusion,minimalist encoding
AI 理解论文
溯源树
样例
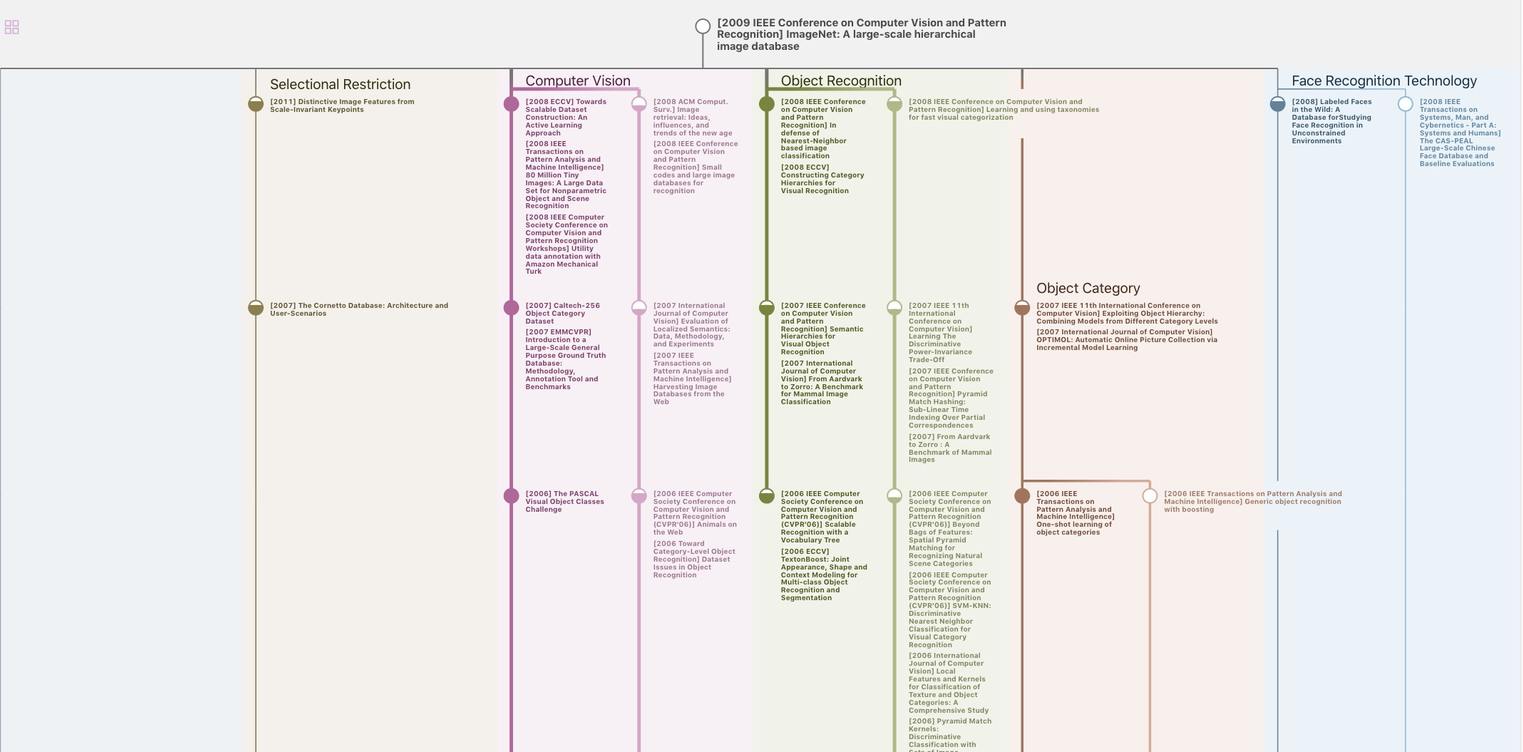
生成溯源树,研究论文发展脉络
Chat Paper
正在生成论文摘要