Promoting Exploration in Memory-Augmented Adam using Critical Momenta
CoRR(2023)
摘要
Adaptive gradient-based optimizers, particularly Adam, have left their mark in training large-scale deep learning models. The strength of such optimizers is that they exhibit fast convergence while being more robust to hyperparameter choice. However, they often generalize worse than non-adaptive methods. Recent studies have tied this performance gap to flat minima selection: adaptive methods tend to find solutions in sharper basins of the loss landscape, which in turn hurts generalization. To overcome this issue, we propose a new memory-augmented version of Adam that promotes exploration towards flatter minima by using a buffer of critical momentum terms during training. Intuitively, the use of the buffer makes the optimizer overshoot outside the basin of attraction if it is not wide enough. We empirically show that our method improves the performance of several variants of Adam on standard supervised language modelling and image classification tasks.
更多查看译文
关键词
adam,exploration,critical momenta,memory-augmented
AI 理解论文
溯源树
样例
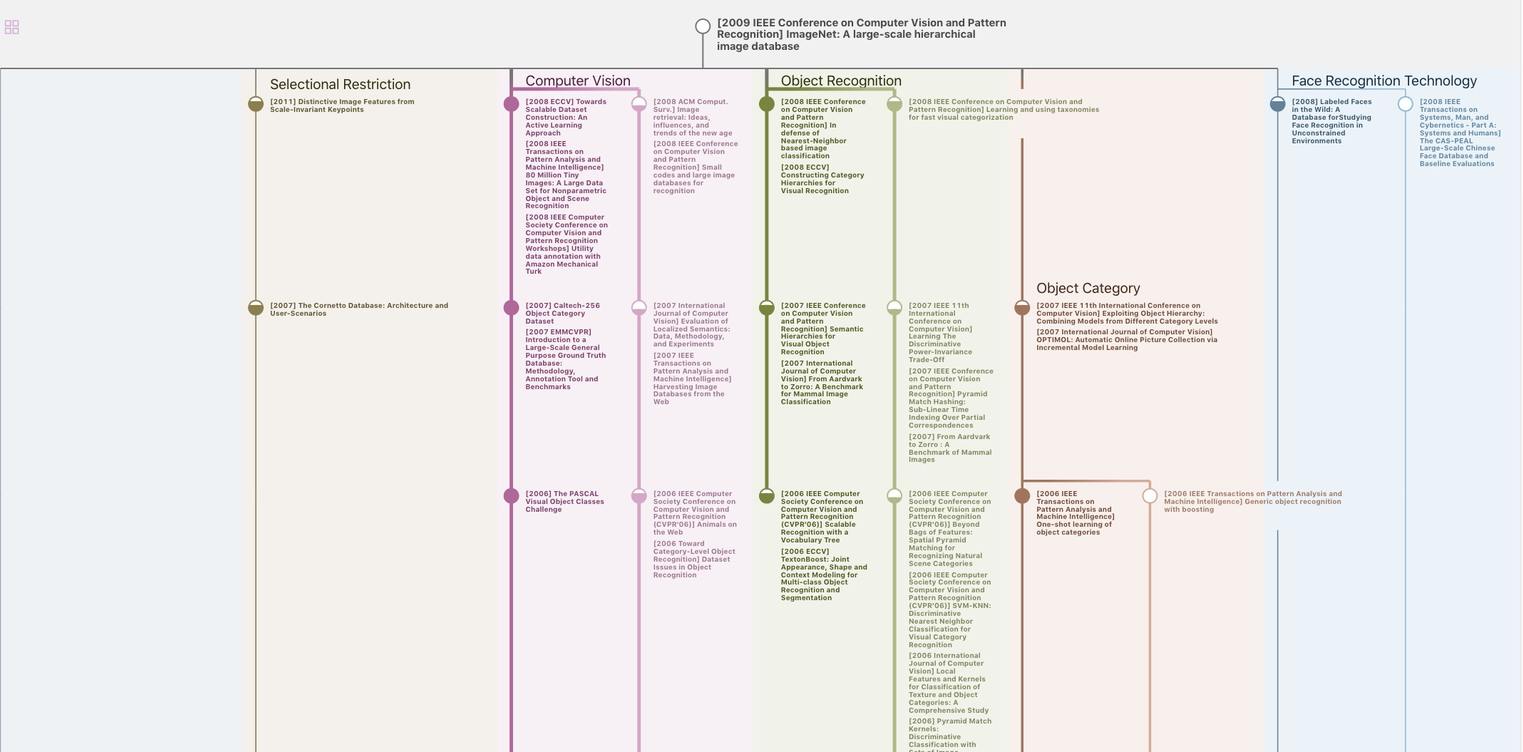
生成溯源树,研究论文发展脉络
Chat Paper
正在生成论文摘要