Multi-scale-based joint super-resolution and inverse tone-mapping with data synthesis for UHD HDR video
Displays(2023)
摘要
The performance of current joint super-resolution (SR) and inverse tone-mapping (ITM) frameworks is limited since they only account for information in small local receptive fields. Moreover, the SDR images in the existing dataset for joint SR-ITM are oversaturated compared to the HDR ground truths. The models trained on this dataset produce HDR images with color shift. This paper proposes a multi-scale-based joint SR-ITM model to reconstruct HR HDR videos. Image features are downsampled to different resolutions to increase the local receptive fields. Thus the proposed model can react to more complex patterns of input images. And we design a novel multi-path residual dense block (MRDB) as the model’s fundamental component to extract features. The proposed MRDBs can improve performance by combining dense feature learning and novel multi-path residual learning. Experiments show that the proposed model outperforms the state-of-the-art methods in terms of quantitative and qualitative results. Furthermore, we propose a data synthesis pipeline to generate perceptually identical SDR images from their HDR counterparts. These SDR-HDR pairs are used to create a new dataset. Experiments demonstrate that models trained with our new dataset prevent color shift and preserve creative intent.
更多查看译文
关键词
data synthesis,multi-scale-based,super-resolution,tone-mapping
AI 理解论文
溯源树
样例
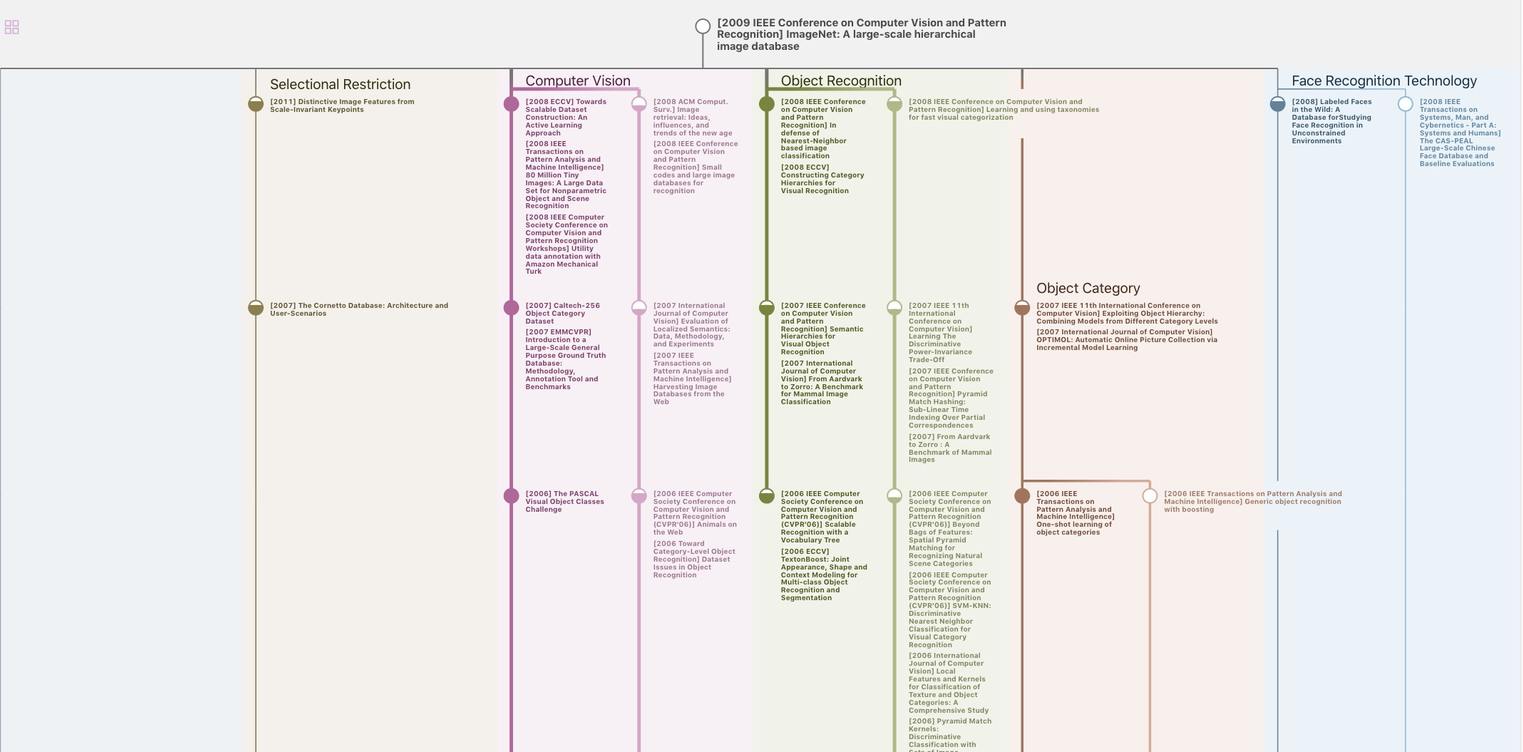
生成溯源树,研究论文发展脉络
Chat Paper
正在生成论文摘要