Predictive control of reactor network model using machine learning for hydrogen-rich gas and biochar poly-generation by biomass waste gasification in supercritical water
ENERGY(2023)
摘要
Supercritical water gasification (SCWG) technology can convert biomass into hydrogen rich gas and biochar. Fluidized bed reactor is promising for the industrialization of this technology, and the reactor dynamic perfor-mance study is of great significance for its scaling up. However, current simulation studies mainly focus on steady-state analysis using Computational Fluid Dynamics (CFD) software, it is difficult to conduct dynamic study due to its high computational costs. To this end, a reactor network model (RNM) that accounts for the flow, heat transfer, and kinetic dynamics for fluidized bed reactor is firstly developed based on the partitioning theory, which can reduce the computation time of dynamic simulation from several days to seconds. Additionally, extensive open-loop simulations of the RNM are carried out to generate the dataset for the development of a recurrent neural network (RNN) model. Additionally, current studies mainly focus on open-loop simulation, closed loop optimization is absent. To this end, a framework for building a machine learning (ML) model and a ML-based nonlinear predictive control scheme is developed. Model predictive control (MPC) schemes based on the RNN model is used to optimize the SCWG process to achieve multiple objectives, such as maximizing hydrogen yield and carbon yield. The open loop simulation results demonstrate that H2 yield decreases from 0.00022 to 0.0002 kg s-1 with temperature reducing from 700 to 600 degrees C. To increase H2 yield to the setpoint, MPC increases temperature and mass flowrate to 708 degrees C and 417.57 kg h-1. Additionally, MPC increases carbon yield and minimizing CO2 yield by decreasing temperature to 475 degrees C and increasing mass flowrate to 407.38 kg h-1.
更多查看译文
关键词
Biomass waste,Hydrogen,Reactor network model,Machine learning,Model predictive control,Dynamic simulation
AI 理解论文
溯源树
样例
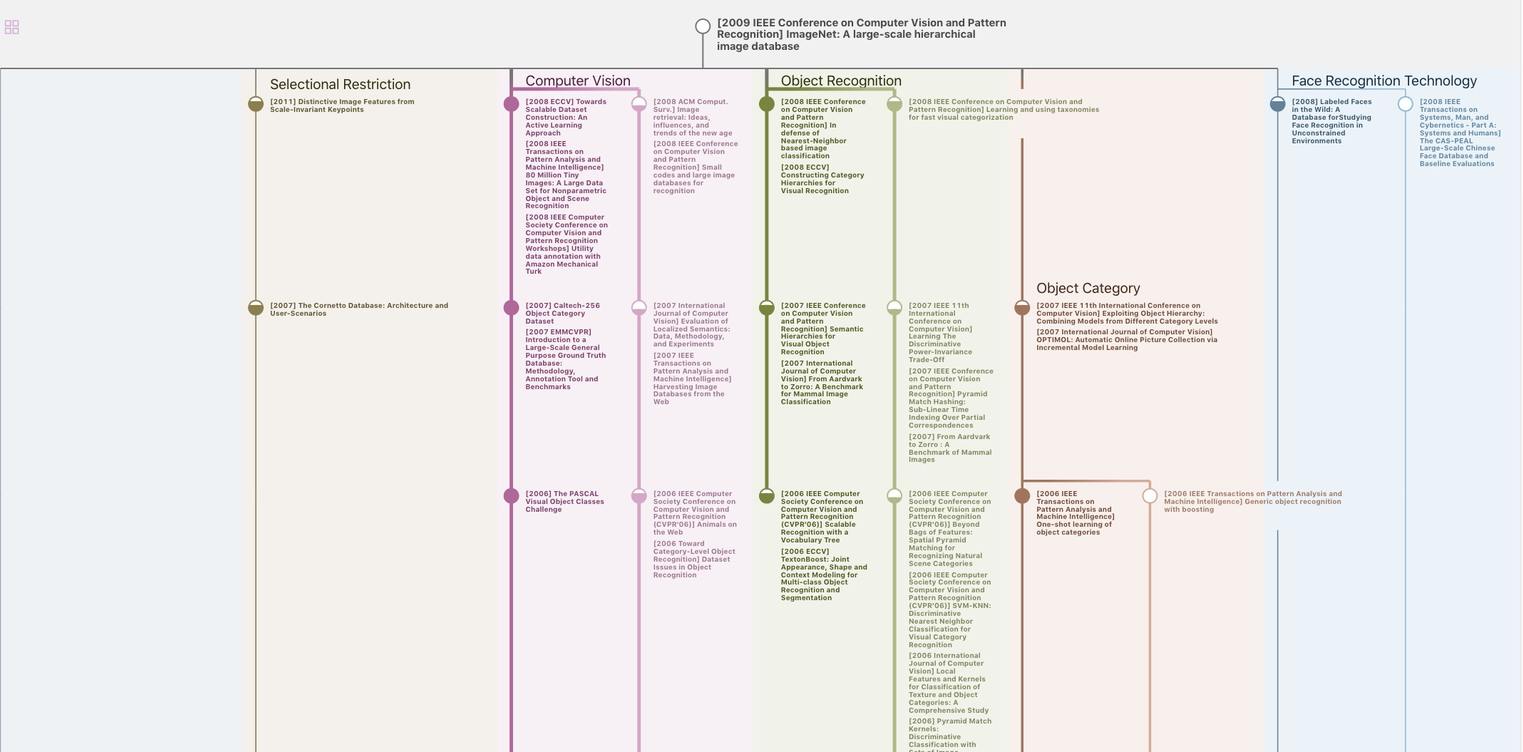
生成溯源树,研究论文发展脉络
Chat Paper
正在生成论文摘要