Advances in Earth observation and machine learning for quantifying blue carbon
Earth-Science Reviews(2023)
摘要
Blue carbon ecosystems (mangroves, seagrasses and saltmarshes) are highly productive coastal habitats, and are considered some of the most carbon-dense ecosystems on Earth. They are an important nature-based solution for both climate change mitigation and adaptation. Quantifying blue carbon stocks and assessing their dynamics at large scales through remote sensing remains challenging due to difficulties of cloud coverage, spectral, spatial and temporal limitations of multispectral sensors and speckle noise of synthetic aperture radar (SAR). Recent advances in airborne and space-borne multispectral and SAR imagery and Light Detection and Ranging (LiDAR) data, sensor platforms such as unmanned aerial vehicles (UAVs), combined with novel machine learning techniques have offered different users with a wide-range of spectral, spatial, and multi-temporal information for quantifying blue carbon from space. However, a large number of challenges are posed by various traits such as atmospheric correction, water penetration, and water column transparency issues in coastal environments, the multi-dimensionality and size of the multispectral and LiDAR data, the limitation of training samples, and backscattering mechanisms of SAR imagery in the acquisition process. As a result, existing methodologies face major difficulties in accurately estimating blue carbon stocks using these datasets. In this context, emerging and innovative machine learning and artificial intelligence methodologies are often required for robustness and reliability of blue carbon estimates, particularly those using open-source software for signal processing and regression tasks. This review provides an overview of Earth Observation data, machine learning and state-of-the-art deep learning techniques that are currently being used to quantify above-ground carbon, below-ground carbon, and soil carbon stocks of mangroves, seagrasses and saltmarshes ecosystems. Some key limitations and future directions for the potential use of data fusion combined with advanced machine learning, deep learning, and metaheuristic optimisation techniques for quantifying blue carbon stocks are also highlighted. In summary, the quantification of blue carbon using remote sensing and machine learning approaches holds great potential in contributing to global efforts towards mitigating climate change and protecting coastal ecosystems.
更多查看译文
关键词
carbon,earth observation,machine learning
AI 理解论文
溯源树
样例
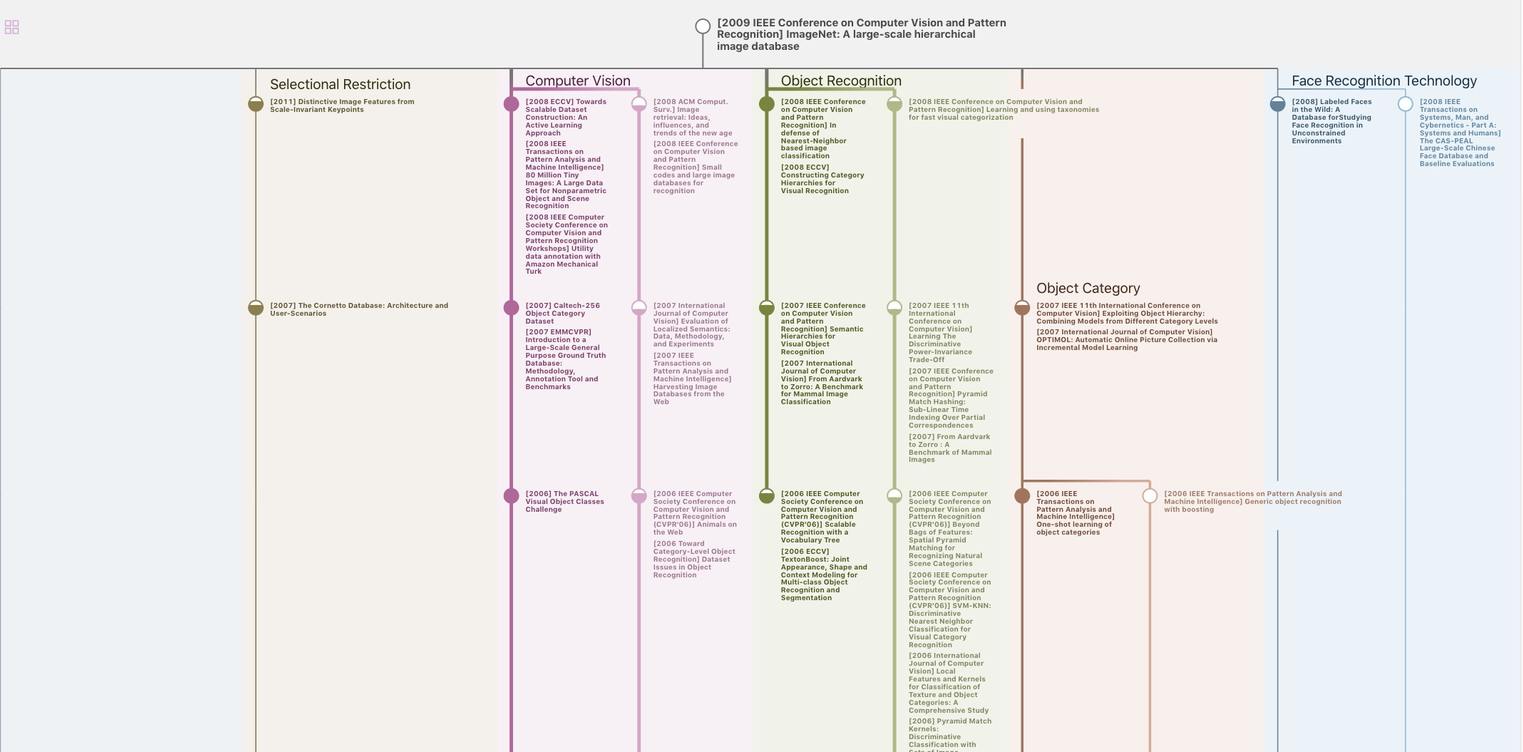
生成溯源树,研究论文发展脉络
Chat Paper
正在生成论文摘要