Discriminating functional and non-functional nuclear-receptor ligands with a conformational selection-inspired machine learning algorithm
Cell Reports Physical Science(2023)
摘要
By targeting the ligand-binding pocket (LBP) and stabilizing the corresponding state (agonistic or antagonistic) of a nuclear receptor (NR), functional ligands, namely agonists and antagonists, can regulate the expression of the downstream genes. In addition to the functional ligands, there exists another category of ligands that can target the LBP of an NR, but without downstream functions, which are called non-functional binders (NFBs). In practice, it is difficult to determine the molecular category of an LBP-bound ligand since these molecules bind to the same position in an NR. Therefore, in this study, we propose a series of structure-based machine-learning (ML) models to better characterize the molecular category of the LBP-bound ligands. Our result shows that the best-performing model achieves good performance in predicting these molecules with an accuracy >0.85 and is robust enough to be applied in real-world tasks, such as identification of a ligand’s category targeting out-of-training-set (or external) NRs.
更多查看译文
关键词
ligands,machine learning,non-functional,nuclear-receptor,selection-inspired
AI 理解论文
溯源树
样例
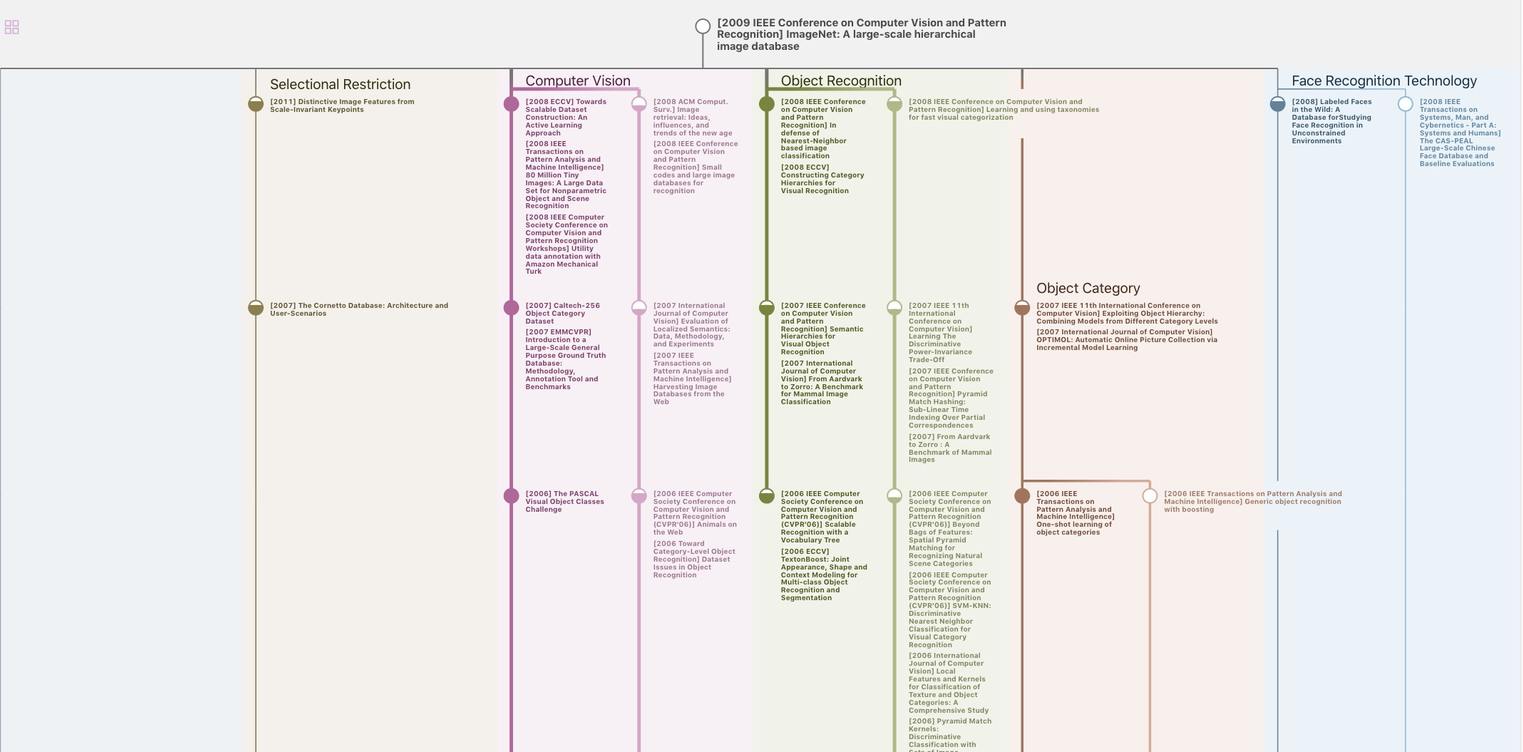
生成溯源树,研究论文发展脉络
Chat Paper
正在生成论文摘要