Privacy-Preserving Decentralized Dual Averaging for Online Optimization Over Directed Networks
IEEE Transactions on Industrial Cyber-Physical Systems(2023)
摘要
In this article, we study a decentralized online constrained optimization problem with a common constraint set, in which the main purpose is to optimize the problem over a certain time interval. Due to the continuously broadcast privacy-related information between nodes, most existing decentralized algorithms that settle this issue may be at risk of privacy leakage. In response to such a dilemma, we propose an effective differentially private decentralized dual averaging algorithm, which takes into account the usages of perturbation with Laplace noise and gradient rescaling strategies to preserve differential privacy and eliminate the unbalancedness of directed networks, respectively. Unlike the existing related methods, the proposed algorithm allows dual variables to have arbitrary initial conditions, which is more applicable in practice. The results indicate that the algorithm we proposed achieves an expected sublinear regret and preserves differential privacy for each node's loss function. The trade-off between the optimization accuracy and the privacy level is also analyzed. In addition, the proposed algorithm also exhibits similar performance for bounded communication delays in the network. Numerical examples are provided for examining the feasibility and performance of the proposed algorithm.
更多查看译文
关键词
dual averaging,online optimization,privacy-preserving
AI 理解论文
溯源树
样例
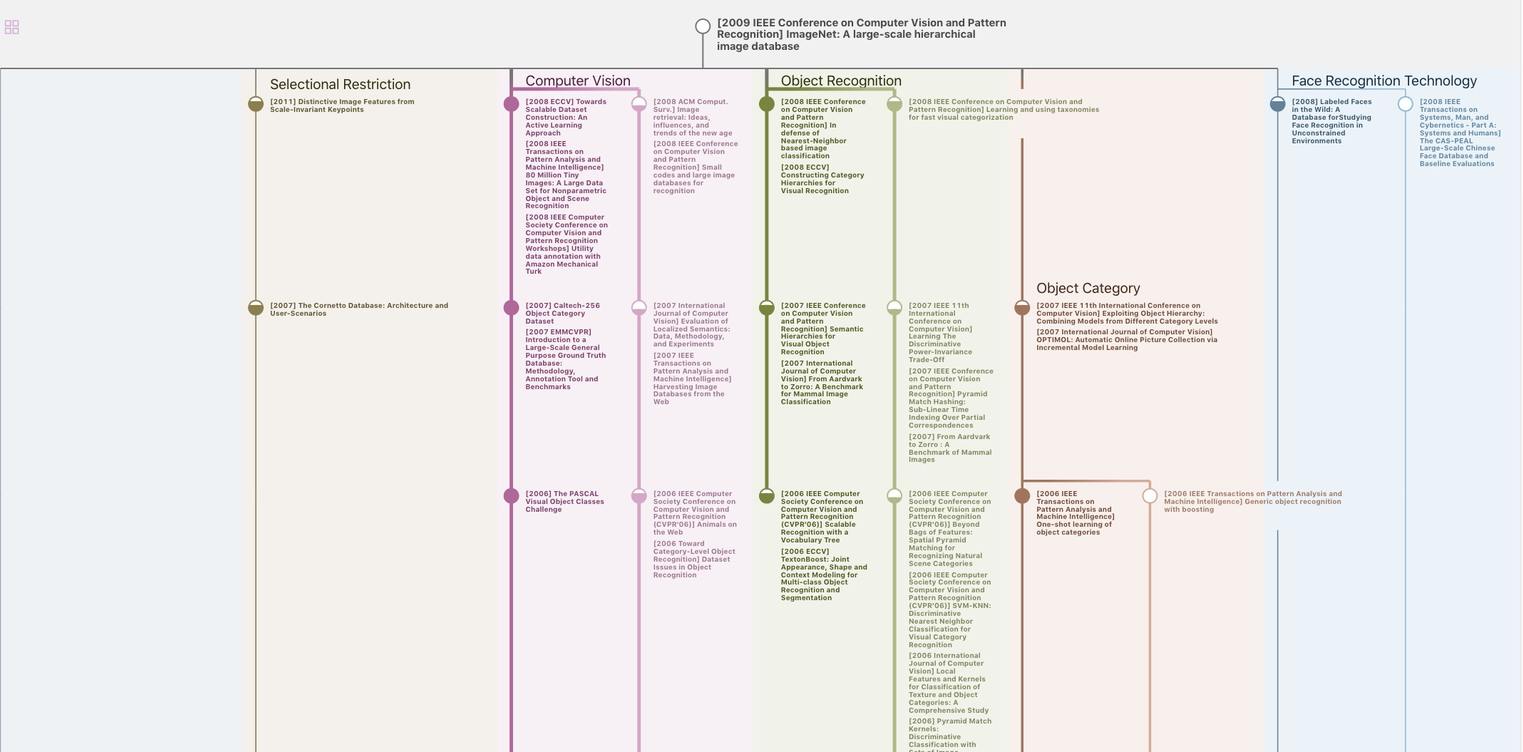
生成溯源树,研究论文发展脉络
Chat Paper
正在生成论文摘要