Conditional pseudo-supervised contrast for data-Free knowledge distillation
Pattern Recognition(2023)
摘要
•We propose a learning paradigm to improve DFKD with a conditional generative adversarial network, which is able to synthesize category-specific images and promote student learning.•We introduce a categorical feature embedding block to effectively distinguish the distribution of different categories of samples, which connect the features and categories embedding in the middle layers.•To our knowledge, we are the first to attempt to utilize the condition annotations to supervise the contrast of features representation of teacher and student in DFKD, which aims to optimize the diversity of synthesized images and improve the effect of distillation.•Massive experiments are conducted on three mainstream benchmark datasets, i.e., CIFAR-10, CIFAR-100, Tiny-ImageNet. The results demonstrate the effectiveness of the proposed CPSCDFKD in both improving the student and generator.
更多查看译文
关键词
Model compression, Knowledge distillation, Representation learning, Contrastive learning, Privacy protection
AI 理解论文
溯源树
样例
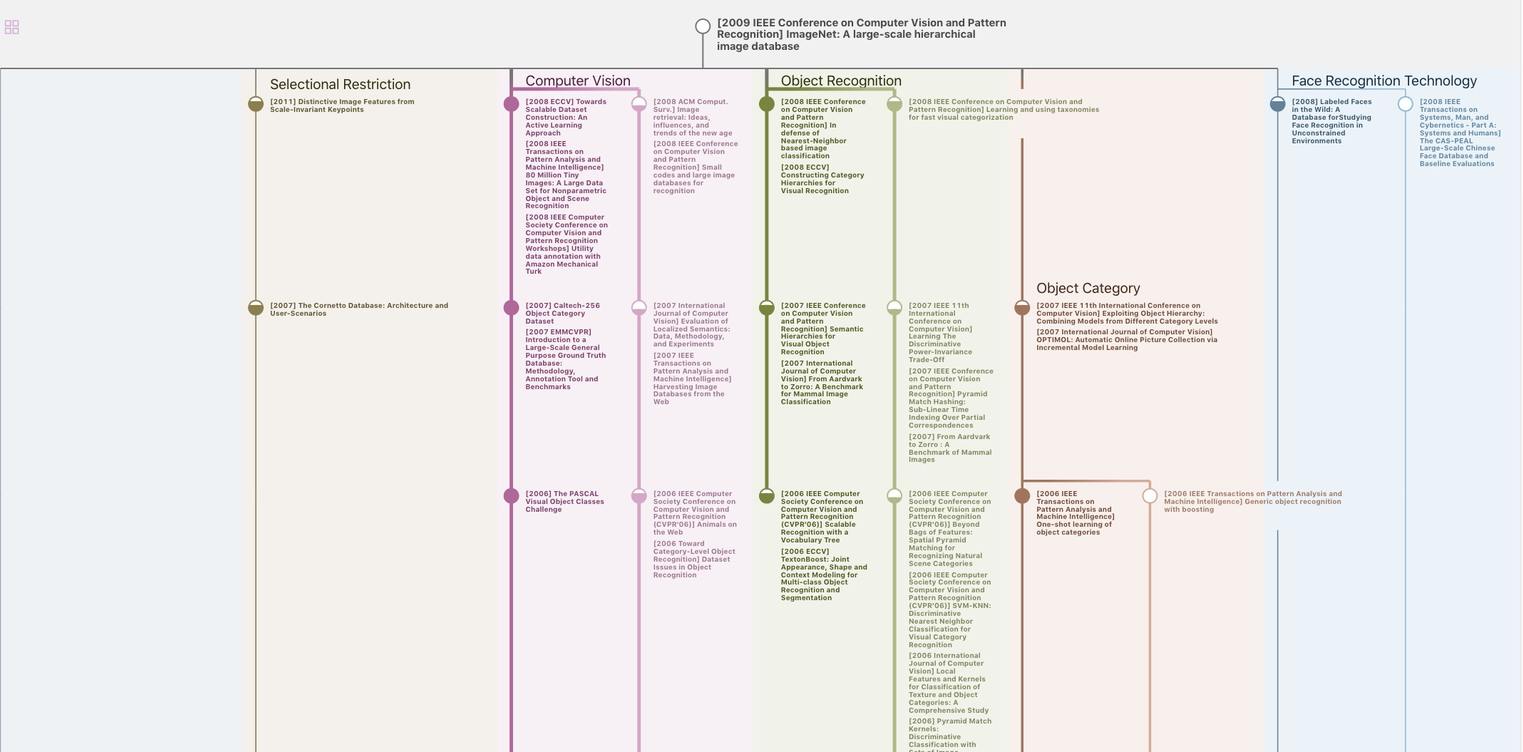
生成溯源树,研究论文发展脉络
Chat Paper
正在生成论文摘要