NeuroPNM: Model reduction of pore network models using neural networks
PARTICUOLOGY(2024)
摘要
Reacting particle systems play an important role in many industrial applications, for example biomass drying or the manufacturing of pharmaceuticals. The numerical modeling and simulation of such systems is therefore of great importance for an efficient, reliable, and environmentally sustainable operation of the processes. The complex thermodynamical, chemical, and flow processes that take place in the particles are a particular challenge in a simulation. Furthermore, typically a large number of particles is involved, rendering an explicit treatment of individual ones impossible in a reactor-level simulation. One approach for overcoming this challenge is to compute effective, physical parameters from single-particle, high-resolution simulations. This can be combined with model reduction methods if the dynamical behaviour of particles must be captured. Pore network models with their unrivaled resolution have thereby been used successfully as high-resolution models, for instance to obtain the macroscopic diffusion coefficient of drying. Both parameter identification and model reduction have recently gained new impetus by the dramatic progress made in machine learning in the last decade. We report results on the use of neural networks for parameter identification and model reduction based on three-dimensional pore network models (PNM). We believe that our results provide a powerful complement to existing methodologies for reactor-level simulations with many thermally-thick particles. & COPY; 2023 Chinese Society of Particuology and Institute of Process Engineering, Chinese Academy of Sciences. Published by Elsevier B.V. All rights reserved.
更多查看译文
关键词
Pore network models,Neural networks,Parameter estimation,Reduced order model
AI 理解论文
溯源树
样例
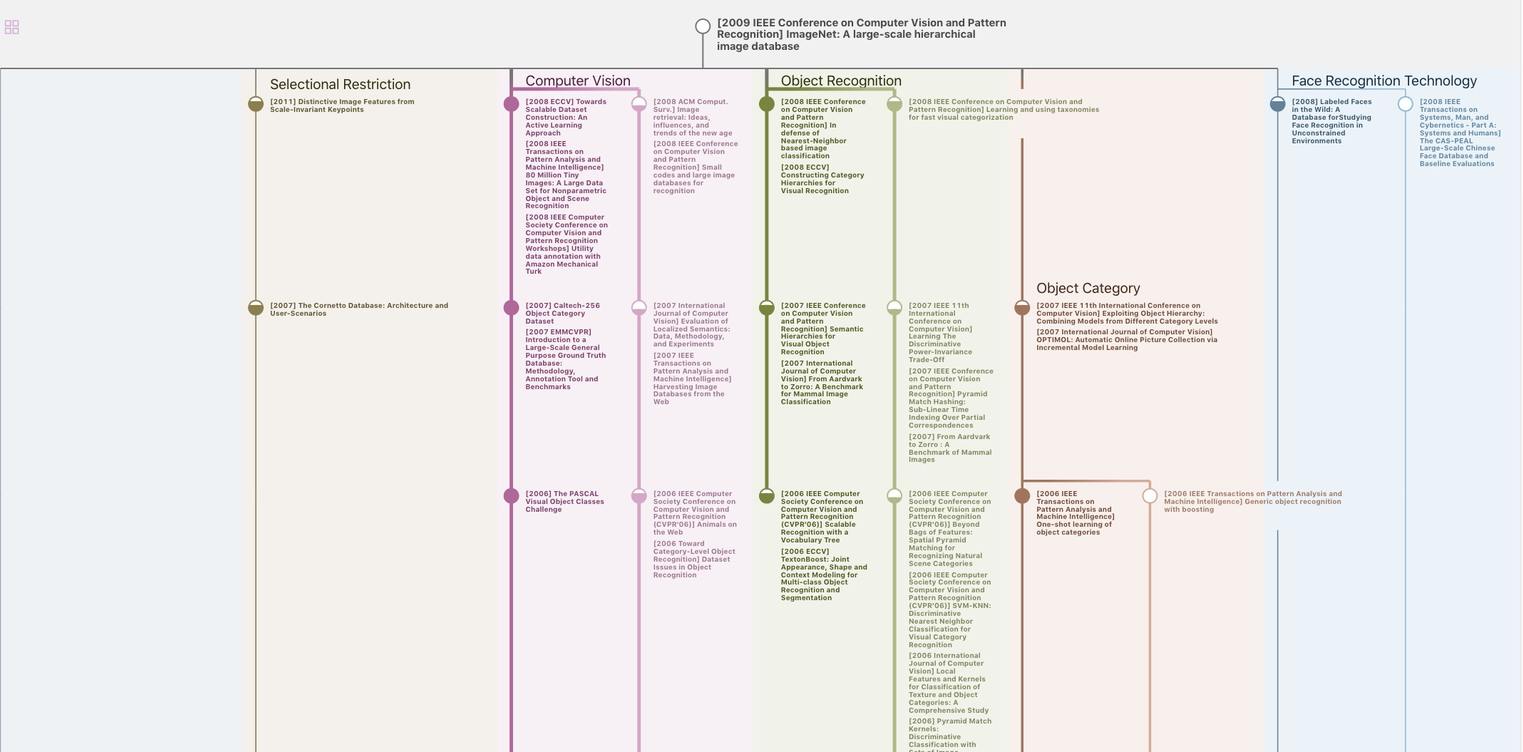
生成溯源树,研究论文发展脉络
Chat Paper
正在生成论文摘要