Deep reinforcement learning for a multi-objective operation in a nuclear power plant
Nuclear Engineering and Technology(2023)
摘要
Nuclear power plant (NPP) operations with multiple objectives and devices are still performed manually by operators despite the potential for human error. These operations could be automated to reduce the burden on operators; however, classical approaches may not be suitable for these multi-objective tasks. An alternative approach is deep reinforcement learning (DRL), which has been successful in automating various complex tasks and has been applied in automation of certain operations in NPPs. But despite the recent progress, previous studies using DRL for NPP operations have limitations to handle complex multi-objective operations with multiple devices efficiently. This study proposes a novel DRL-based approach that addresses these limitations by employing a continuous action space and straightforward binary rewards supported by the adoption of a soft actor-critic and hindsight experience replay. The feasibility of the proposed approach was evaluated for controlling the pressure and volume of the reactor coolant while heating the coolant during NPP startup. The results show that the proposed approach can train the agent with a proper strategy for effectively achieving multiple objectives through the control of multiple devices. Moreover, hands-on testing results demonstrate that the trained agent is capable of handling untrained objectives, such as cooldown, with substantial success.
更多查看译文
关键词
deep reinforcement learning,nuclear power plant,multi-objective
AI 理解论文
溯源树
样例
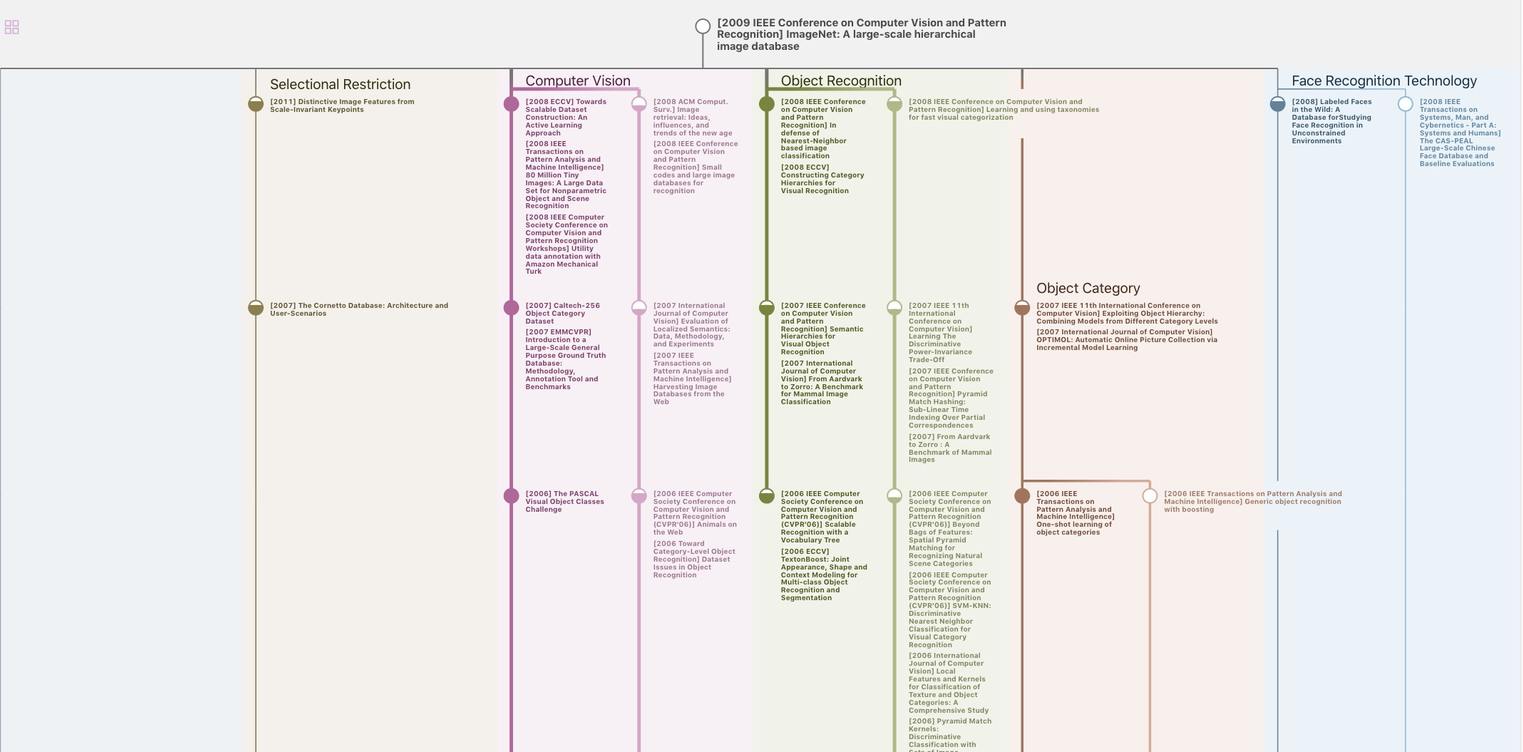
生成溯源树,研究论文发展脉络
Chat Paper
正在生成论文摘要