A hybrid machine learning-genetic algorithm (ML-GA) model to predict optimal process parameters of nanoporous Cu for CO2 reduction
Materials Today Energy(2023)
摘要
The electrocatalytic performance of nanoporous copper (NPC) in carbon dioxide reduction reaction (CO2RR) varies based on given features during the sample preparation phases and reaction conditions of each sample. This work aims to improve the CO2RR capabilities of NPC regarding C2 products in a flow cell setup by altering process parameters during electrode preparation. A dataset consisting of five electrode process parameters and five Faradaic efficiencies (FE) of major products was employed as the respective inputs and outputs. A machine learning-genetic algorithm (ML-GA) structure was subsequently used to discover NPC's maximum sum of C2-specific faradaic efficiencies. Our results show that the extreme gradient boosting regression (XGBR) algorithm would be the favorable option as the base model and is later combined with the evolutionary GA to predict the best process parameters for optimal C2 FE. A validation test using the best results shows that the sum of C2-specific FE (ethylene, ethanol and acetic acid) was 66.71%. The space-time yield of C2 products in this work, 4693 mmol/(h*g), was higher than that of other Cu catalysts. The results of this work demonstrate the potential of machine learning methods in effectively finding the optimal NPC electrode process parameters for enhanced CO2RR results. & COPY; 2023 Elsevier Ltd. All rights reserved.
更多查看译文
关键词
Nanoporous copper (NPC),Carbon dioxide reduction reaction(CO2RR),Machine learning (ML),Genetic algorithm (GA)
AI 理解论文
溯源树
样例
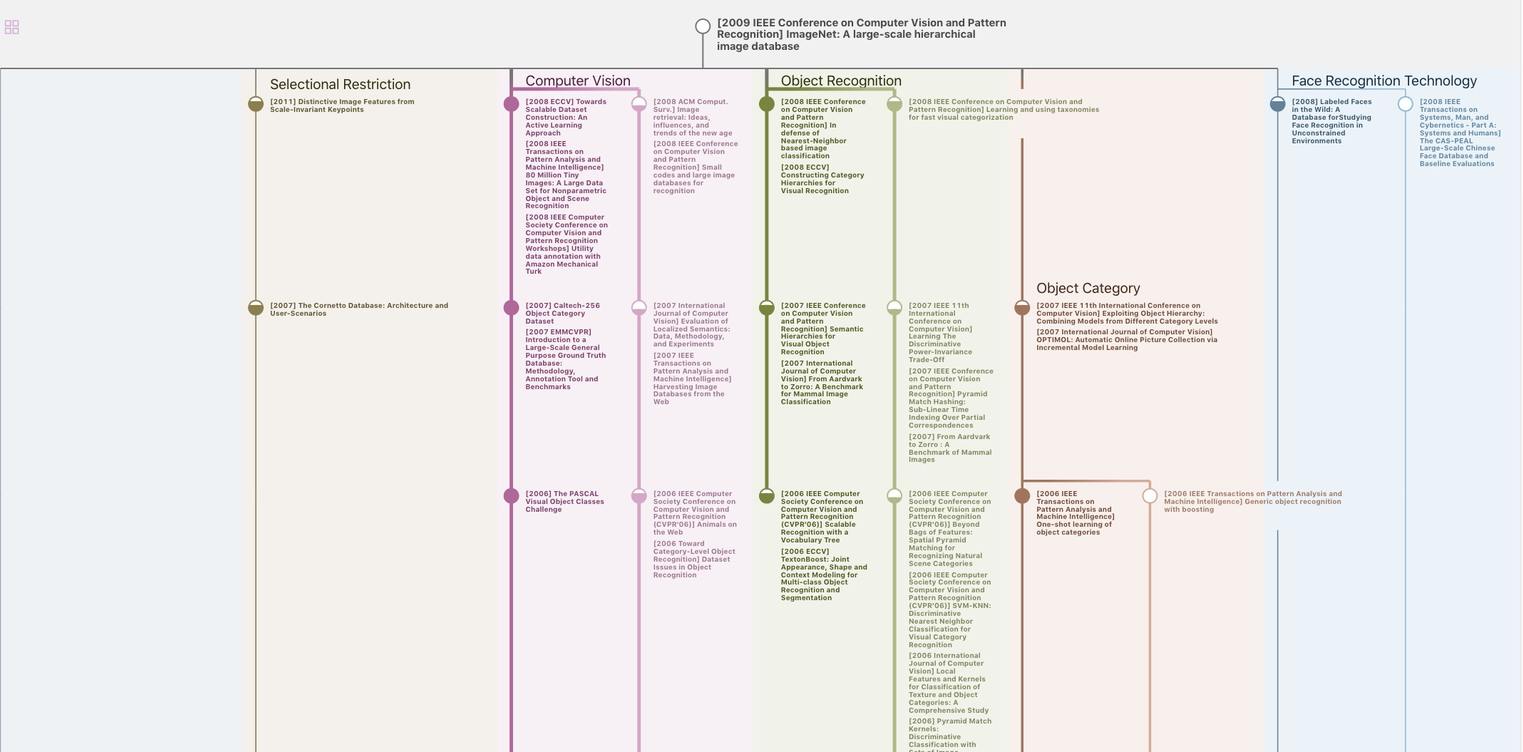
生成溯源树,研究论文发展脉络
Chat Paper
正在生成论文摘要