An explainable ensemble of multi-view deep learning model for fake review detection
JOURNAL OF KING SAUD UNIVERSITY-COMPUTER AND INFORMATION SCIENCES(2023)
摘要
Online reviews significantly impact consumers who are purchasing or seeking services via the Internet. Businesses and review platforms need to manage these online reviews to avoid misleading customers through fake ones. This necessitates developing intelligent solutions to detect these fake reviews and pre-vent their negative impact on businesses and customers. Therefore, many fake review detection models have been proposed to help distinguish fake reviews from genuine ones. However, these techniques depend on a limited perspective of features, mainly review content, to detect fake reviews, leading to poor performance in discovering the new patterns of fake review content and the dynamic behaviour of spammers. Therefore, there is still a need to develop new solutions to detect the new patterns of fake reviews. Hence, this paper proposes an explainable multi-view deep learning model to identify fake reviews based on different feature perspectives and classifiers. The proposed model can extract essential features from different perspectives, including review content, reviewer data, and product description. Moreover, we employ an ensemble approach that combines three popular deep learning algorithms: Bi-LSTM, CNN, and DNN, to enhance the performance of the fake review detection model. The results of two real-life datasets presented demonstrated the efficiency of our proposed model, where it outper-formed the state-of-the-art methods with improvements ranging from 1% to 7% in terms of the AUC met-ric. To provide visibility into the outcomes of our proposed model and demonstrate the trust and transparency in the obtained results, we also offer a comprehensive explanation for our model results using Shapely Additive Explanations (SHAP) method and attention techniques. The experimental results prove that our proposed model can provide reasonable explanations that help users understand why specific reviews are classified as fake.(c) 2023 The Author(s). Published by Elsevier B.V. on behalf of King Saud University. This is an open access article under the CC BY license (http://creativecommons.org/licenses/by/4.0/).
更多查看译文
关键词
Deep learning,Fake reviews,Fake reviews detection,Feature engineering,Explainability
AI 理解论文
溯源树
样例
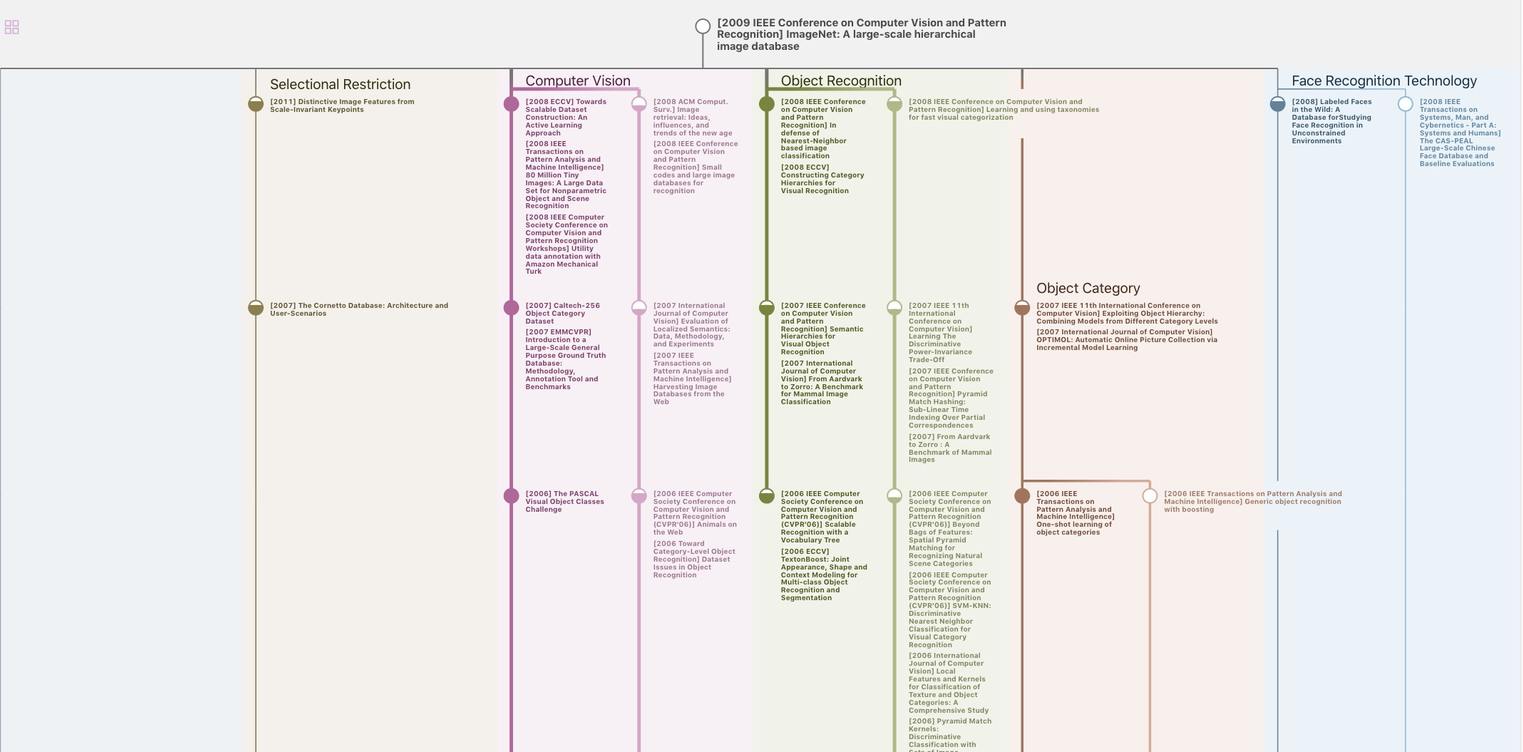
生成溯源树,研究论文发展脉络
Chat Paper
正在生成论文摘要