A benchmark for evaluating Arabic contextualized word embedding models
Information Processing & Management(2023)
摘要
Word embeddings, which represent words as numerical vectors in a high-dimensional space, are contextualized by generating a unique vector representation for each sense of a word based on the surrounding words and sentence structure. They are typically generated using such deep learning models as BERT and trained on large amounts of text data and using self-supervised learning techniques. Resulting embeddings are highly effective at capturing the nuances of language, and have been shown to significantly improve the performance of numerous NLP tasks. Word embeddings represent textual records of human thinking, with all the mental relations that we utilize to produce the succession of sentences that make up texts and discourses. Consequently, the distributed representation of words within embeddings ought to capture the reasoning relations that hold texts together. This paper makes its contribution to the field by proposing a benchmark for the assessment of contextualized word embeddings that probes into their capability for true contextualization by inspecting how well they capture resemblance, contrariety, comparability, identity, relations in time and space, causation, analogy, and sense disambiguation. The proposed metrics adopt a triangulation approach, so they use (1) Hume’s reasoning relations, (2) standard analogy, and (3) sense disambiguation. The benchmark has been evaluated against 22 Arabic contextualized embeddings and has proven to be capable of quantifying their differential performance in terms of these reasoning relations. Results of evaluation of the target embeddings revealed that they do take context into account and that they do reasonably well in sense disambiguation but have weakness in their identification of converseness, synonymy, complementarity, and analogy. Results also show that size of an embedding has diminishing returns because the highly frequent language patterns swamp low frequency patterns. Furthermore, the suggest that future research endeavors should not be concerned with the quantity of data as much as its quality, and that it should focus more on the representativeness of data, and on model architecture, design, and training.
更多查看译文
关键词
arabic contextualized word,benchmark
AI 理解论文
溯源树
样例
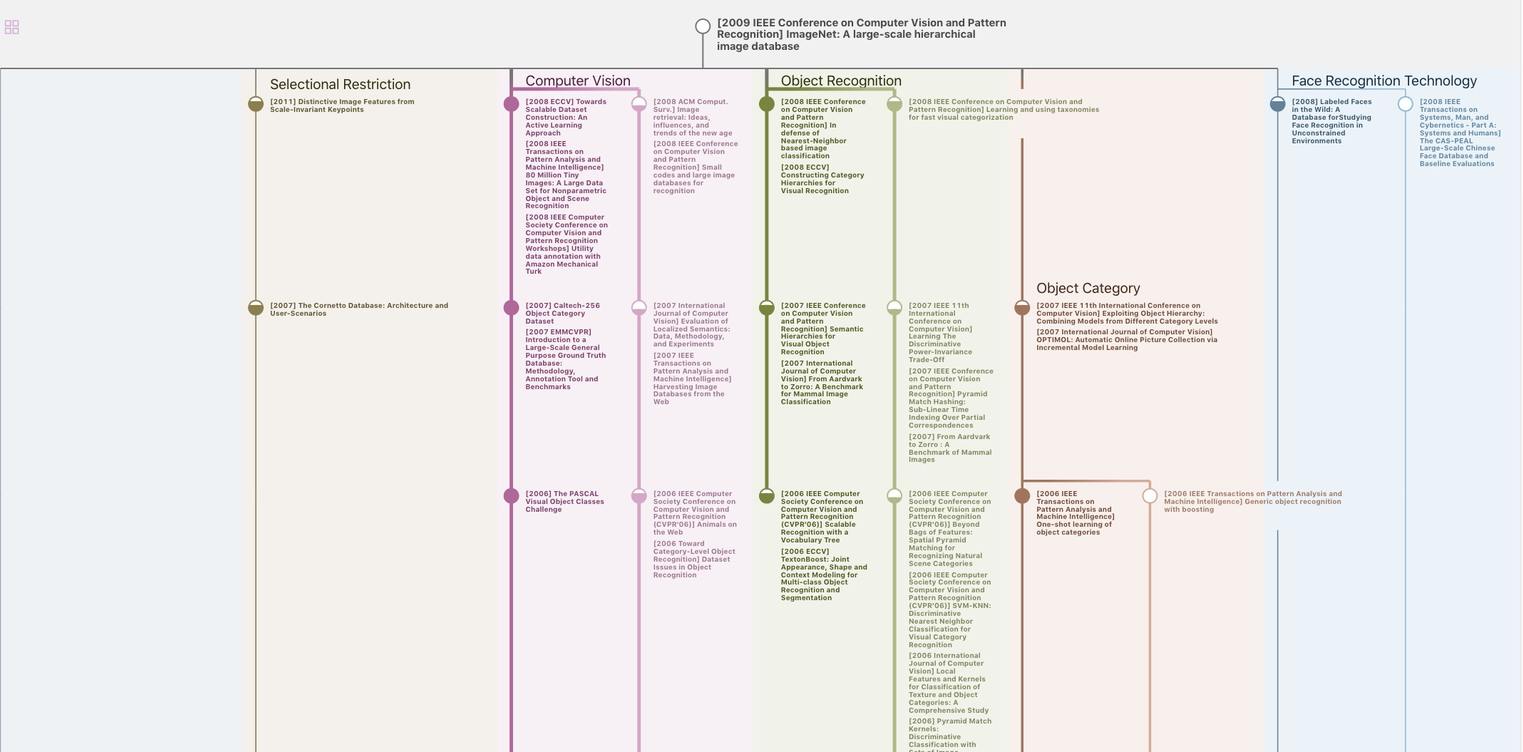
生成溯源树,研究论文发展脉络
Chat Paper
正在生成论文摘要