Construction of a novel predictive model with seven metabolism-related genes for hepatocellular carcinoma by machine learning
GENES & DISEASES(2023)
摘要
Single-cell RNA sequencing (scRNA-seq) and machine learning technologies, developed rapidly in recent years, offer an unparalleled opportunity to study the mechanism of tumorigenesis. Changes in metabolism-related genes (MRGs) result in hepatocellular carcinoma (HCC) progression, and MRGs have the potential to be used as a clinical prognostic indicator. In this study, a machine learning model was built using LASSO regression to find prognostic genes and create a new MRG risk signature of HCC patients. The patients were divided into the MRG high- and low-risk groups based on their risk scores. Significant overall survival advantages were observed in the MRG low-risk group. In addition, risk score and cancer status were used to establish a nomogram with high accuracy. The calibration curves and decision curves further demonstrated the satisfactory agreement between the predicted and observed values in the probability of overall survival. Subsequently, we discovered the different characteristics of the MRG high- and low-risk group patients based on tumor mutation burden, gene set enrichment, and immune infiltration. Our study identifies a novel MRG signature for risk-stratification and accurate identification of HCC patients with poor subtypes, which could precisely predict the prognosis of HCC and may guide personalized treatment for HCC patients.
更多查看译文
关键词
hepatocellular carcinoma,novel predictive model,machine learning,genes,predictive model,metabolism-related
AI 理解论文
溯源树
样例
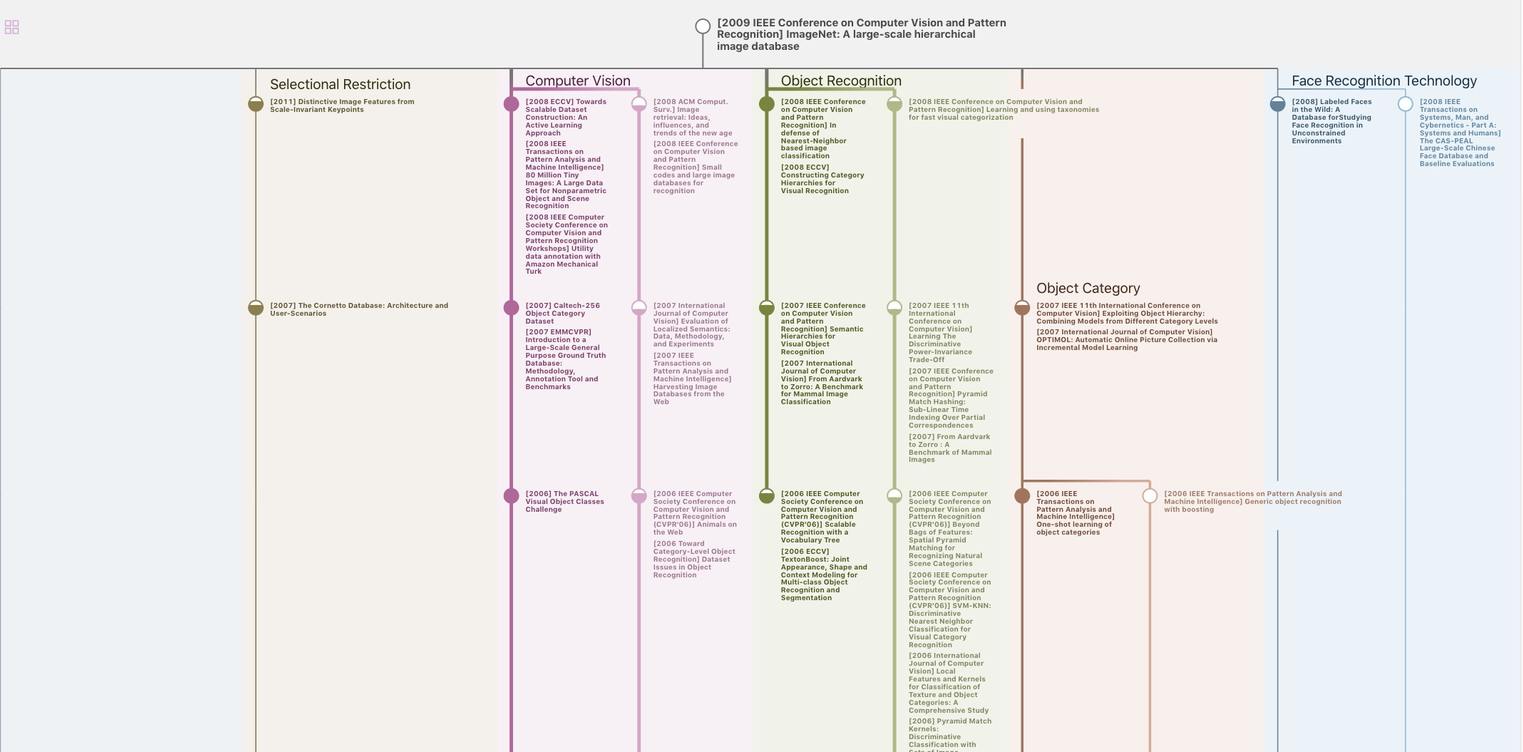
生成溯源树,研究论文发展脉络
Chat Paper
正在生成论文摘要