Differential privacy in deep learning: Privacy and beyond
Future Generation Computer Systems(2023)
摘要
Motivated by the security risks of deep neural networks, such as various membership and attribute inference attacks, differential privacy has emerged as a promising approach for protecting the privacy of neural networks. As a result, it is crucial to investigate the frontier intersection of differential privacy and deep learning, which is the main motivation behind this survey. Most of the current research in this field focuses on developing mechanisms for combining differentially private perturbations with deep learning frameworks. We provide a detailed summary of these works and analyze potential areas for improvement in the near future. In addition to privacy protection, differential privacy can also play other critical roles in deep learning, such as fairness, robustness, and prevention of over-fitting, which have not been thoroughly explored in previous research. Accordingly, we also discuss future research directions in these areas to offer practical suggestions for future studies.
更多查看译文
关键词
Deep learning, Differential privacy, Stochastic gradient descent, Lower bound, Fairness, Robustness
AI 理解论文
溯源树
样例
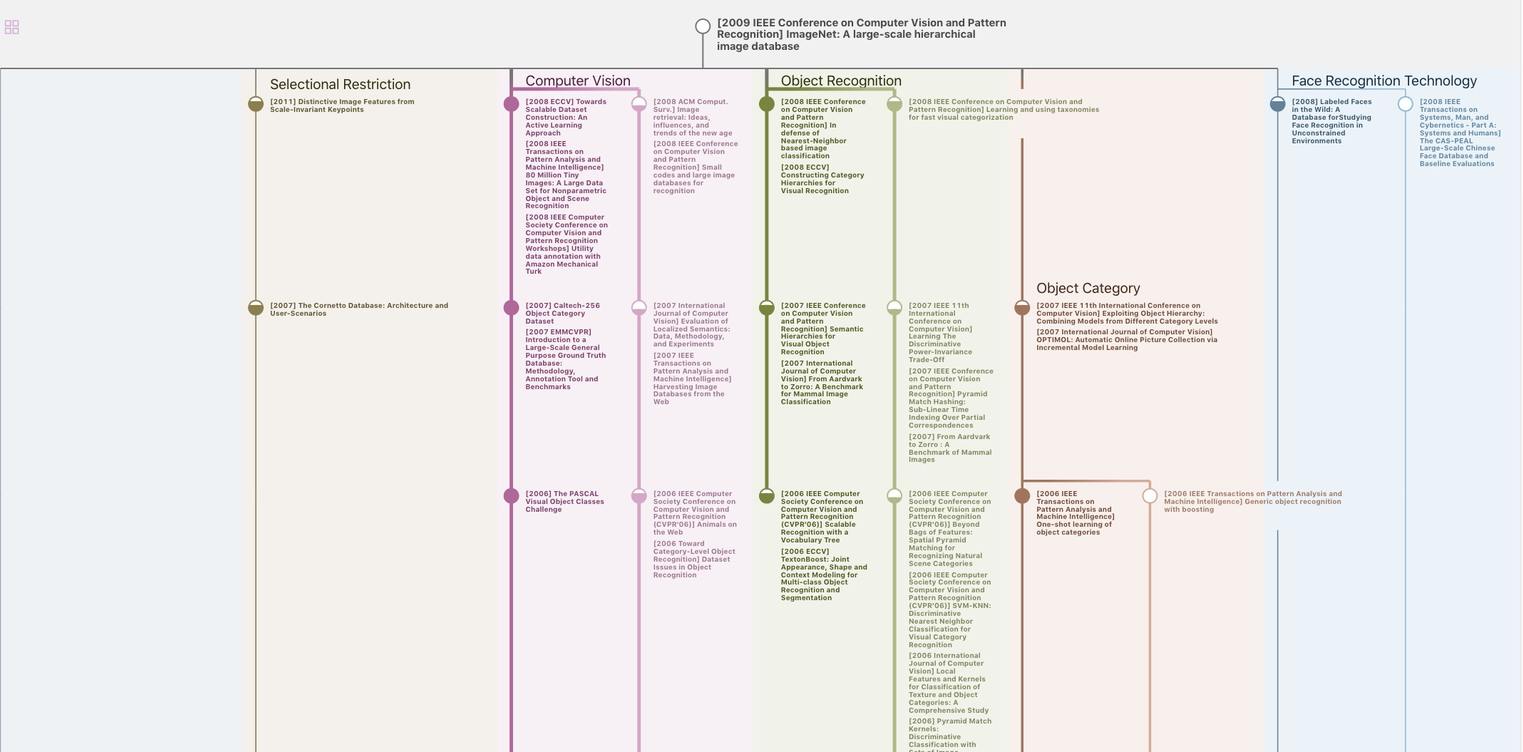
生成溯源树,研究论文发展脉络
Chat Paper
正在生成论文摘要