Physics-informed deep learning of gas flow-melt pool multi-physical dynamics during powder bed fusion
CIRP Annals(2023)
摘要
The effect of inert gas on melt pool dynamics has been largely overlooked but is crucial for laser powder bed fusion (LPBF). Physics-based simulation models are computationally expensive while data-driven models lack transparency and need massive training data. This work presents a physics-informed deep learning (PIDL) model to accurately predict the temperature and velocity fields in the melting domain using only a small training data. The PIDL model can also learn unknown model constants (e.g., Reynolds number and Peclet number) of the governing equations. Furthermore, the robust PIDL algorithm converges very fast by enforcing physics via soft penalty constraints.
更多查看译文
关键词
Selective laser melting,Dynamics,Physics-informed machine learning
AI 理解论文
溯源树
样例
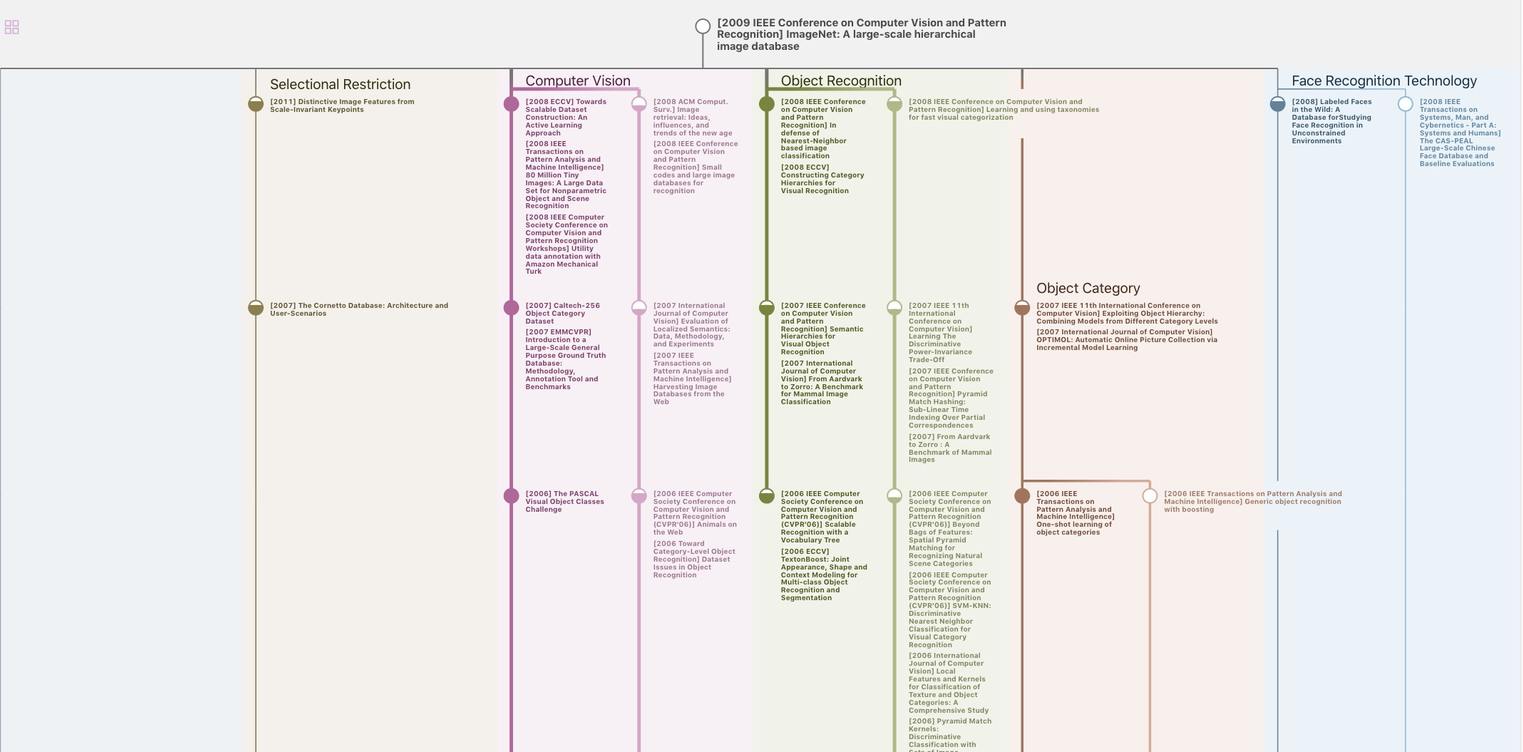
生成溯源树,研究论文发展脉络
Chat Paper
正在生成论文摘要