Graph neural network-based spatio-temporal indoor environment prediction and optimal control for central air-conditioning systems
BUILDING AND ENVIRONMENT(2023)
摘要
Model-based optimal control has proven its effectiveness in optimizing the performance of central airconditioning systems in terms of thermal comfort and energy efficiency. It was often assumed that temperature distribution in the entire air-conditioned space is uniform and can be represented by a single or averaged measurement in optimization. However, actual distribution in the air-conditioned space is usually uneven, which can affect thermal comfort and indoor air quality. The dynamics of air conditioning systems and their interactions with the built environment exist in both time and space domains. Conventional data-driven approaches typically concentrate on the temporal correlations only among building operation data, while neglecting the spatial correlations. This study proposed a spatio-temporal data-driven methodology for optimal control of central air conditioning systems, which aims to address the challenging issue of uneven spatial distributions of environmental parameters in air-conditioned space. The methodology consists of graph-based multisource data integration, graph neural network-based indoor environment modeling and spatio-temporal modelbased online optimal control. The proposed methodology is tested on real air handling unit-variable air volume (AHU-VAV) system in a high-rise office building through a cloud-based platform. Spatio-temporal data from building automation system (BAS), internet of things (IoT) devices and building information modeling (BIM) are integrated and organized as graph structure. Graph neural network models are developed for predicting the evolution of indoor air temperature distribution under different control settings. The developed graph neural network-recurrent neural network (GNN-RNN) model architectures show enhanced accuracy than conventional deep learning models (i.e., convolutional neural network-recurrent neural network (CNN-RNN), Dense-RNN). And the cloud-based online test demonstrates that the proposed model-based control strategy improves the percentage of time achieving Grade I thermal comfort from 36.5% (i.e., existing control strategy) to 81.3%.
更多查看译文
关键词
Machine learning,Graph neural network,Indoor environment,Optimal control,Air conditioning system
AI 理解论文
溯源树
样例
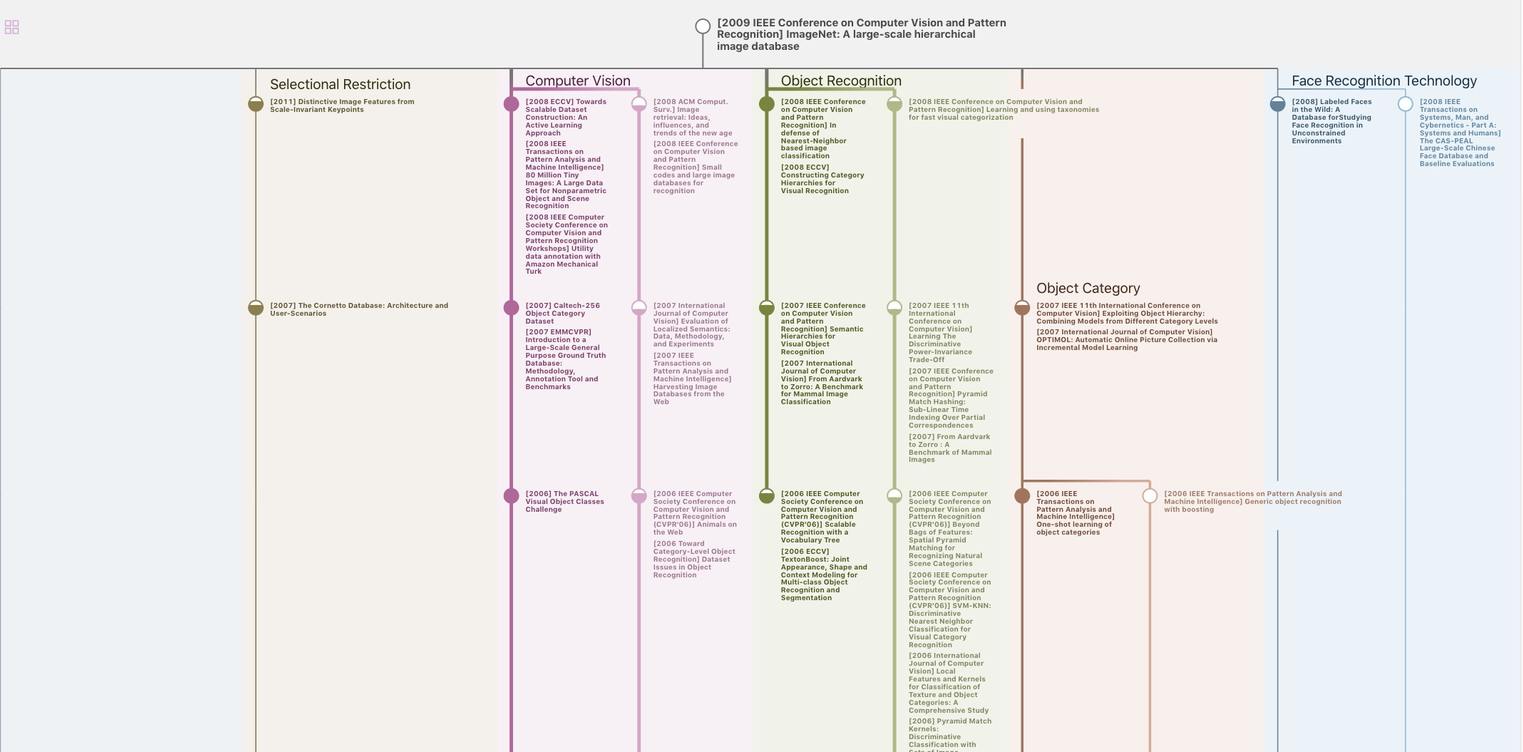
生成溯源树,研究论文发展脉络
Chat Paper
正在生成论文摘要