Deep learning-enabled diagnosis of liver adenocarcinoma
Zeitschrift Fur Gastroenterologie(2023)
摘要
The diagnosis of a gland-forming carcinoma within the liver is a frequent scenario in routine pathology with critical impact on clinical decision-making. However, rendering the correct diagnosis can be challenging and often requires integration of clinical, radiological and immunohistochemical information. We present a deep learning model (HEPNET) to distinguish intrahepatic cholangiocarcinoma (iCCA) from colorectal liver metastasis (CRM) as the most frequent primary and secondary forms of liver adenocarcinoma at clinical-grade accuracy from hematoxylin and eosin-stained whole-slide images. HEPNET was trained on 714 589 image tiles from 456 patients randomly selected in a stratified manner from a pool of 571 patients that underwent surgical resection or biopsy at Heidelberg University Hospital. Model performance was evaluated on a hold-out internal test set comprising 115 patients and externally validated on 90 patients recruited at Mainz University Hospital. On the hold-out internal test set, HEPNET achieved an AUROC of 0.994 and an accuracy of 96.522% on the patient-level. Validation on the external test set yielded an AUROC of 0.997, corresponding to an accuracy of 98.889%. HEPNET significantly outperformed six pathologists with different levels of experience in a reader study on 50 patients, boosted the performance of resident pathologists to the level of senior pathologists and reduced potential downstream analyses. We here provide a ready-to-use tool with clinical-grade performance that may facilitate routine pathology in both rendering a definitive diagnosis and guiding ancillary testing. Incorporation of HEPNET into pathology laboratories may optimize diagnostic workflow complemented by test-related labor and cost savings.
更多查看译文
关键词
liver adenocarcinoma,diagnosis,learning-enabled
AI 理解论文
溯源树
样例
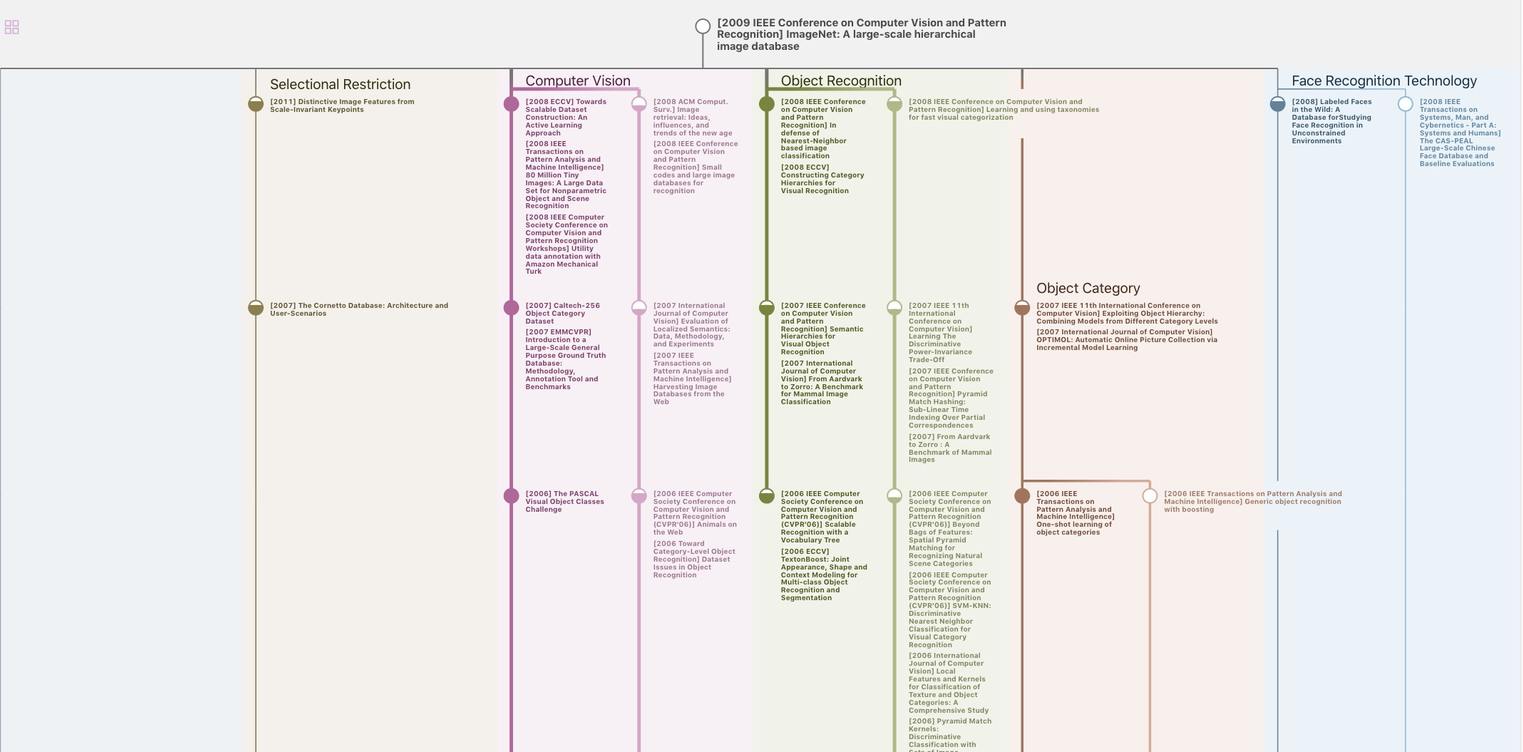
生成溯源树,研究论文发展脉络
Chat Paper
正在生成论文摘要