PT-symmetric solitons and parameter discovery in self-defocusing saturable nonlinear Schr & ouml;dinger equation via LrD-PINN
Chaos (Woodbury, N.Y.)(2023)
摘要
We propose a physical information neural network with learning rate decay strategy (LrD-PINN) to predict the dynamics of symmetric, asymmetric, and antisymmetric solitons of the self-defocusing saturable nonlinear Schrodinger equation with the PT-symmetric potential and boost the predicted evolutionary distance by an order of magnitude. Taking symmetric solitons as an example, we explore the advantages of the learning rate decay strategy, analyze the anti-interference performance of the model, and optimize the network structure. In addition, the coefficients of the saturable nonlinearity strength and the modulation strength in the PT-symmetric potential are reconstructed from the dataset of symmetric soliton solutions. The application of more advanced machine learning techniques in the field of nonlinear optics can provide more powerful tools and richer ideas for the study of optical soliton dynamics.
更多查看译文
关键词
saturable nonlinear schrödinger equation,pt-symmetric,self-defocusing,lrd-pinn
AI 理解论文
溯源树
样例
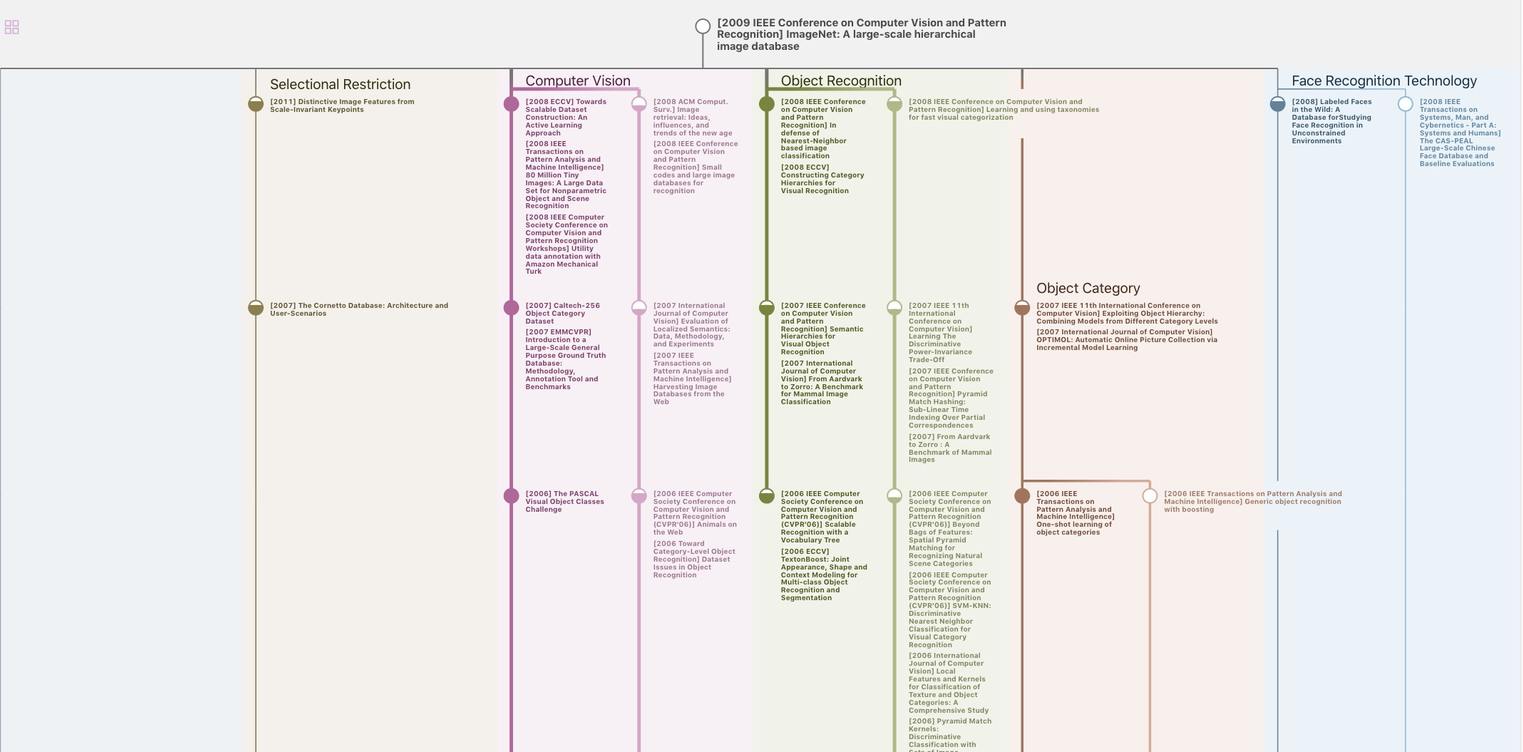
生成溯源树,研究论文发展脉络
Chat Paper
正在生成论文摘要