Jointly Improving the Sample and Communication Complexities in Decentralized Stochastic Minimax Optimization
AAAI 2024(2024)
摘要
We propose a novel single-loop decentralized algorithm, DGDA-VR, for solving the stochastic nonconvex strongly-concave minimax problems over a connected network of agents, which are equipped with stochastic first-order oracles to estimate their local gradients. DGDA-VR, incorporating variance reduction, achieves O(ε^−3) oracle complexity and O(ε^−2) communication complexity without resorting to multi-communication rounds – both are optimal, i.e., matching the lower bounds for this class of problems. Since DGDA-VR does not require multiple communication rounds, it is applicable to a broader range of decentralized computational environments. To the best of our knowledge, this is the first distributed method using a single communication round in each iteration to jointly optimize the oracle and communication complexities for the problem considered here.
更多查看译文
关键词
SO: Non-convex Optimization,ML: Distributed Machine Learning & Federated Learning,ML: Optimization,SO: Distributed Search
AI 理解论文
溯源树
样例
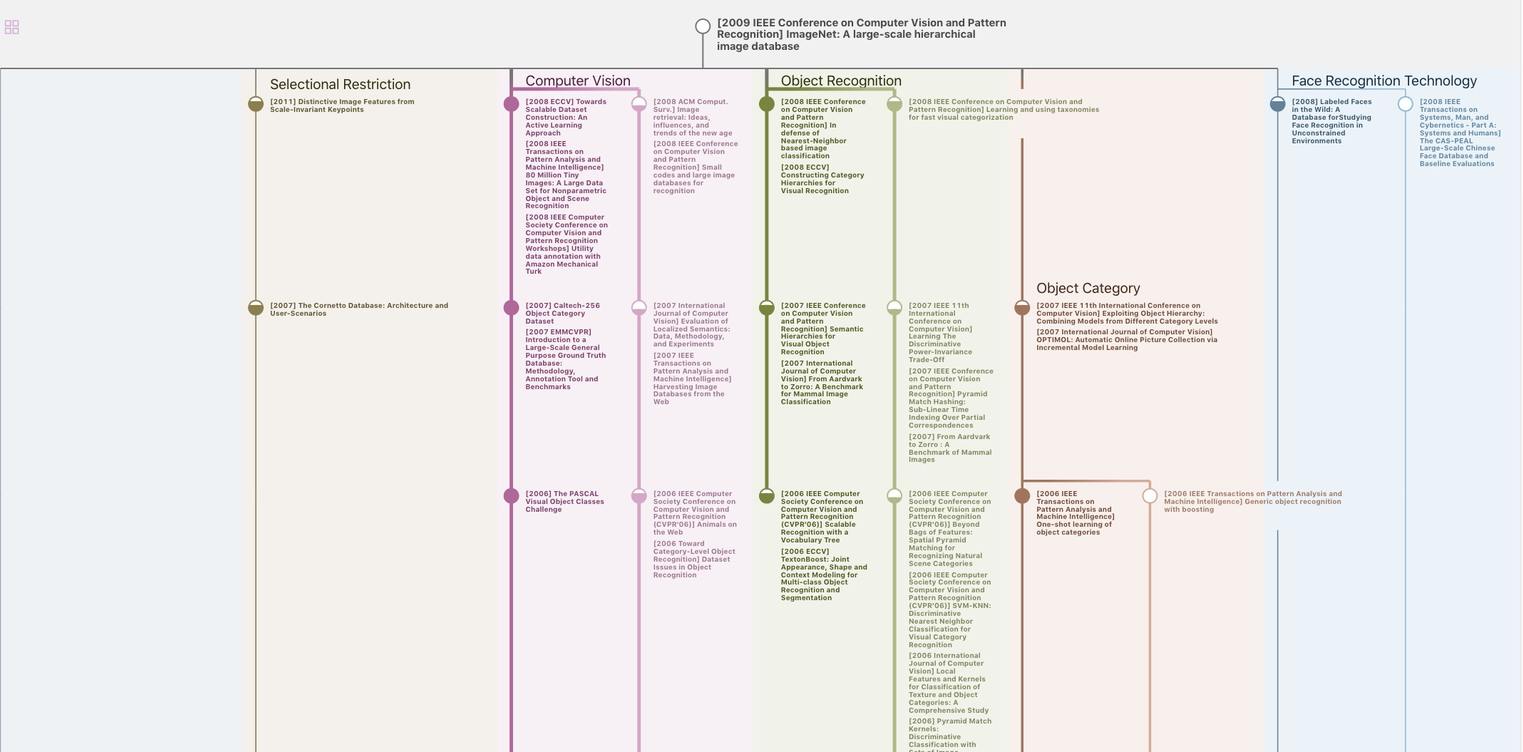
生成溯源树,研究论文发展脉络
Chat Paper
正在生成论文摘要