GraphCL-DTA: A Graph Contrastive Learning With Molecular Semantics for Drug-Target Binding Affinity Prediction.
CoRR(2024)
摘要
Drug-target binding affinity prediction plays an important role in the early stages of drug discovery, which can infer the strength of interactions between new drugs and new targets. However, the performance of previous computational models is limited by the following drawbacks. The learning of drug representation relies only on supervised data without considering the information in the molecular graph itself. Moreover, most previous studies tended to design complicated representation learning modules, while uniformity used to measure representation quality is ignored. In this study, we propose GraphCL-DTA, a graph contrastive learning with molecular semantics for drug-target binding affinity prediction. This graph contrastive learning framework replaces the dropout-based data augmentation strategy by performing data augmentation in the embedding space, thereby better preserving the semantic information of the molecular graph. A more essential and effective drug representation can be learned through this graph contrastive framework without additional supervised data. Next, we design a new loss function that can be directly used to adjust the uniformity of drug and target representations. By directly optimizing the uniformity of representations, the representation quality of drugs and targets can be improved. The effectiveness of the above innovative elements is verified on two real datasets, KIBA and Davis. Compared with the GraphDTA model, the relative improvement of the GraphCL-DTA model on the two datasets is 2.7% and 4.5%. The graph contrastive learning framework and uniformity function in the GraphCL-DTA model can be embedded into other computational models as independent modules to improve their generalization capability.
更多查看译文
关键词
Drug-target binding affinity prediction,Graph contrastive learning,Molecular graph,Uniformity of representations,Drug discovery
AI 理解论文
溯源树
样例
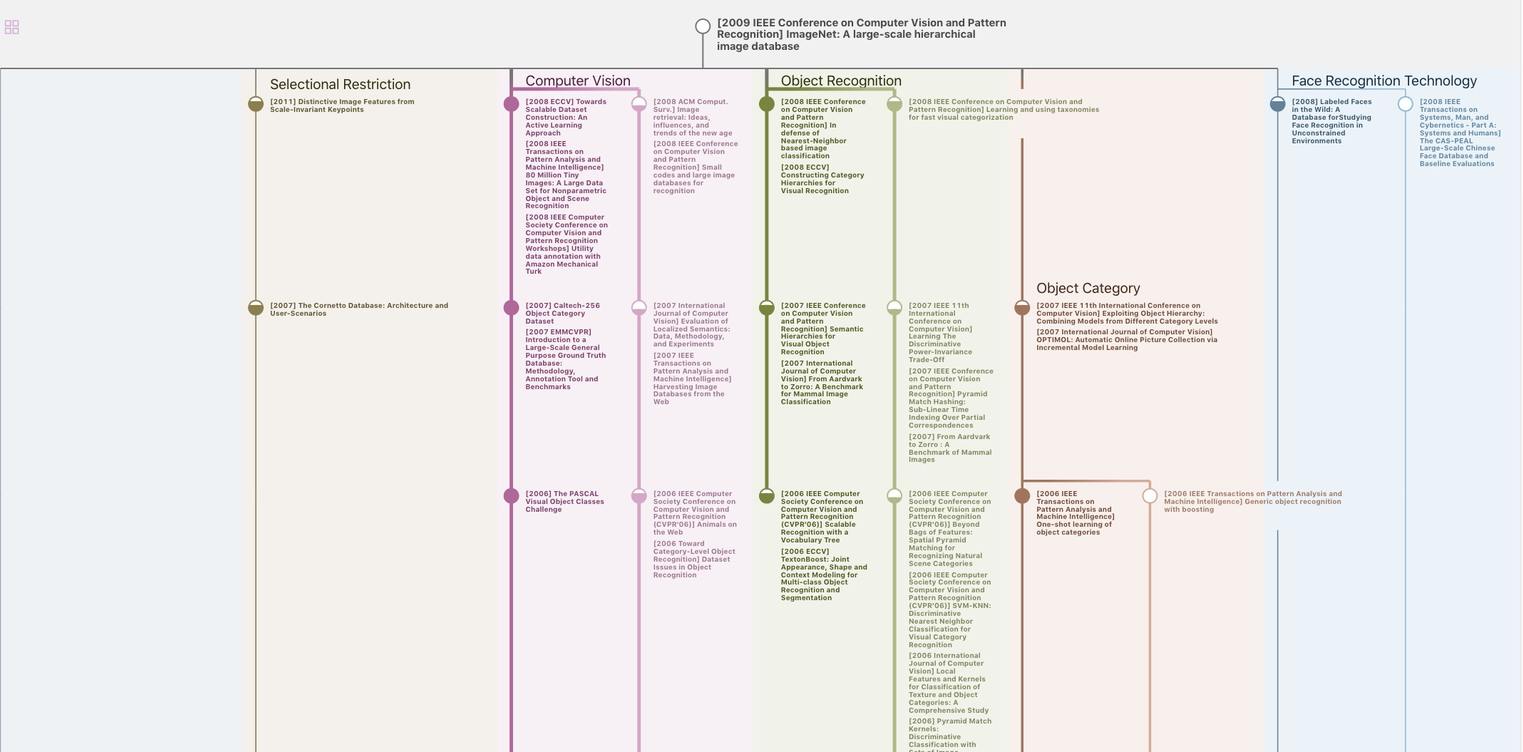
生成溯源树,研究论文发展脉络
Chat Paper
正在生成论文摘要