Non-Stationary Policy Learning for Multi-Timescale Multi-Agent Reinforcement Learning
2023 62ND IEEE CONFERENCE ON DECISION AND CONTROL, CDC(2023)
摘要
In multi-timescale multi-agent reinforcement learning (MARL), agents interact across different timescales. In general, policies for time-dependent behaviors, such as those induced by multiple timescales, are non-stationary. Learning non-stationary policies is challenging and typically requires sophisticated or inefficient algorithms. Motivated by the prevalence of this control problem in real-world complex systems, we introduce a simple framework for learning non-stationary policies for multi-timescale MARL. Our approach uses available information about agent timescales to define and learn periodic multi-agent policies. In detail, we theoretically demonstrate that the effects of non-stationarity introduced by multiple timescales can be learned by a periodic multi-agent policy. To learn such policies, we propose a policy gradient algorithm that parameterizes the actor and critic with phase-functioned neural networks, which provide an inductive bias for periodicity. The framework's ability to effectively learn multi-timescale policies is validated on a gridworld and building energy management environment.
更多查看译文
关键词
reinforcement learning,non-stationary,multi-timescale,multi-agent
AI 理解论文
溯源树
样例
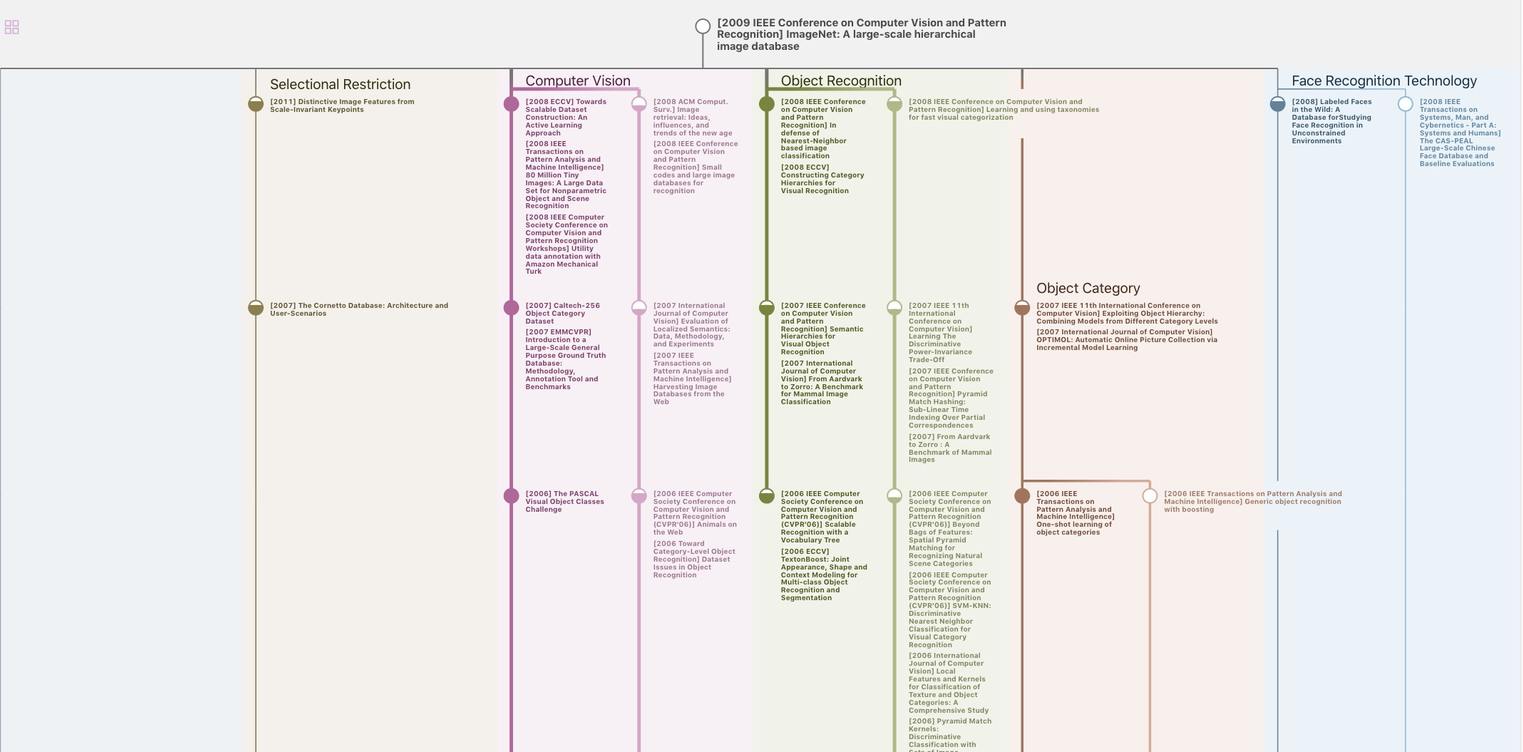
生成溯源树,研究论文发展脉络
Chat Paper
正在生成论文摘要