A Novel Application of Conditional Normalizing Flows: Stellar Age Inference with Gyrochronology
CoRR(2023)
摘要
Stellar ages are critical building blocks of evolutionary models, but challenging to measure for low mass main sequence stars. An unexplored solution in this regime is the application of probabilistic machine learning methods to gyrochronology, a stellar dating technique that is uniquely well suited for these stars. While accurate analytical gyrochronological models have proven challenging to develop, here we apply conditional normalizing flows to photometric data from open star clusters, and demonstrate that a data-driven approach can constrain gyrochronological ages with a precision comparable to other standard techniques. We evaluate the flow results in the context of a Bayesian framework, and show that our inferred ages recover literature values well. This work demonstrates the potential of a probabilistic data-driven solution to widen the applicability of gyrochronological stellar dating.
更多查看译文
关键词
stellar age
AI 理解论文
溯源树
样例
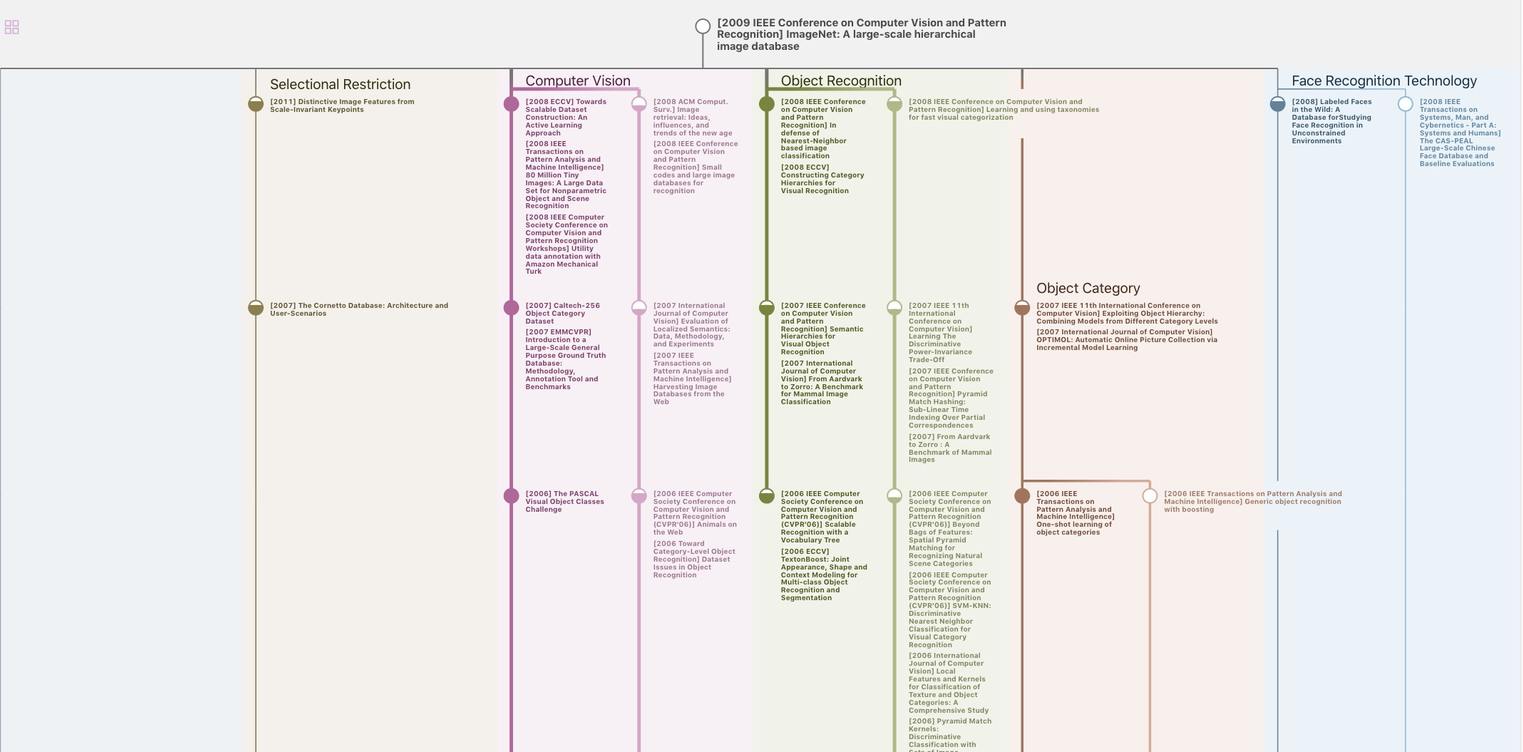
生成溯源树,研究论文发展脉络
Chat Paper
正在生成论文摘要