FedDefender: Backdoor Attack Defense in Federated Learning
CoRR(2023)
摘要
Federated Learning (FL) is a privacy-preserving distributed machine learning technique that enables individual clients (e.g., user participants, edge devices, or organizations) to train a model on their local data in a secure environment and then share the trained model with an aggregator to build a global model collaboratively. In this work, we propose FedDefender, a defense mechanism against targeted poisoning attacks in FL by leveraging differential testing. FedDefender first applies differential testing on clients’ models using a synthetic input. Instead of comparing the output (predicted label), which is unavailable for synthetic input, FedDefender fingerprints the neuron activations of clients’ models to identify a potentially malicious client containing a backdoor. We evaluate FedDefender using MNIST and FashionMNIST datasets with 20 and 30 clients, and our results demonstrate that FedDefender effectively mitigates such attacks, reducing the attack success rate (ASR) to 10% without deteriorating the global model performance.
更多查看译文
关键词
backdoor attack defense,learning
AI 理解论文
溯源树
样例
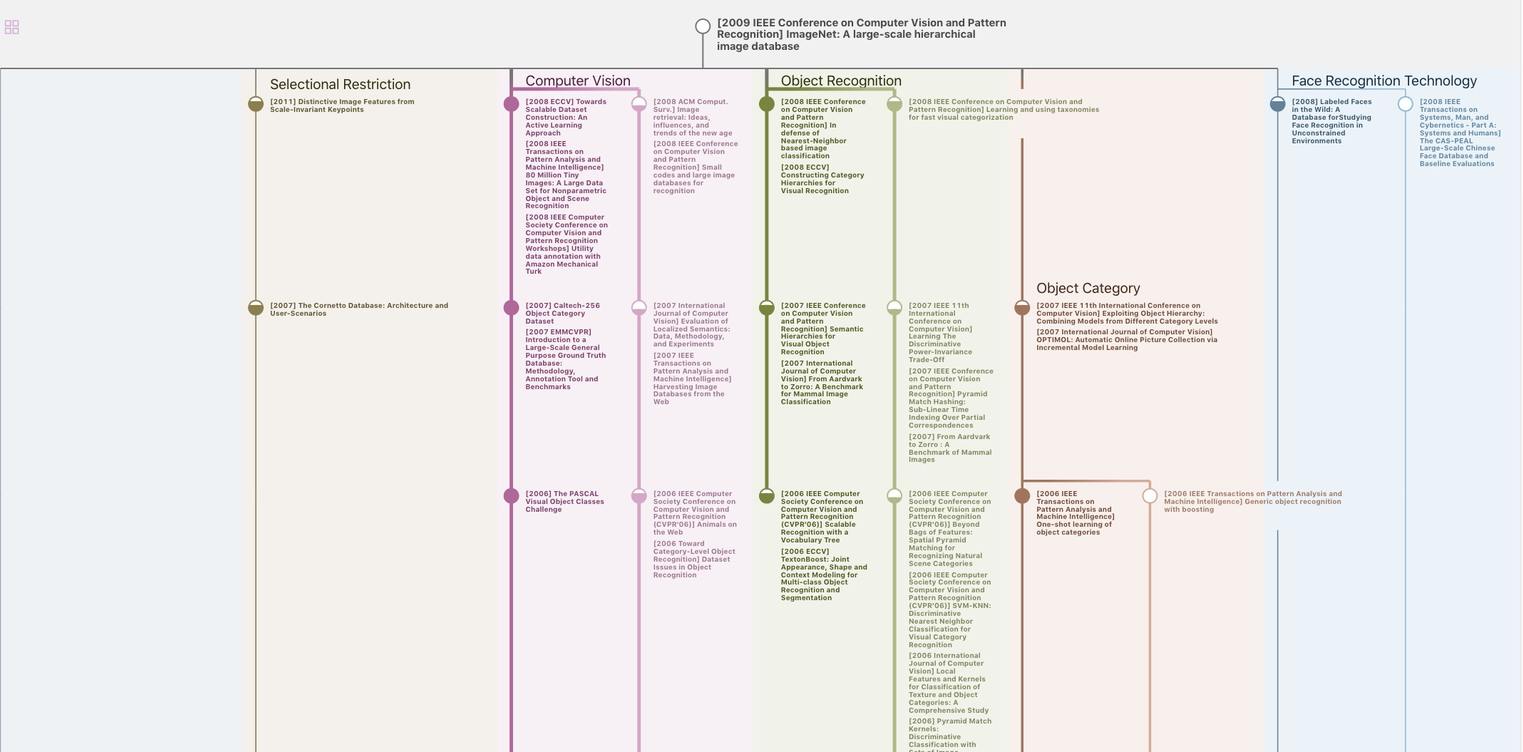
生成溯源树,研究论文发展脉络
Chat Paper
正在生成论文摘要