M-FLAG: Medical Vision-Language Pre-training with Frozen Language Models and Latent Space Geometry Optimization
MEDICAL IMAGE COMPUTING AND COMPUTER ASSISTED INTERVENTION, MICCAI 2023, PT I(2023)
摘要
Medical vision-language models enable co-learning and integrating features from medical imaging and clinical text. However, these models are not easy to train and the latent representation space can be complex. Here we propose a novel way for pre-training and regularising medical vision-language models. The proposed method, named Medical vision-language pre-training with Frozen language models and Latent spAce Geometry optimization (M-FLAG), leverages a frozen language model for training stability and efficiency and introduces a novel orthogonality loss to harmonize the latent space geometry. We demonstrate the potential of the pre-trained model on three downstream tasks: medical image classification, segmentation, and object detection. Extensive experiments across five public datasets demonstrate that M-FLAG significantly outperforms existing medical vision-language pre-training approaches and reduces the number of parameters by 78%. Notably, M-FLAG achieves outstanding performance on the segmentation task while using only 1% of the RSNA dataset, even outperforming ImageNet pre-trained models that have been fine-tuned using 100% of the data. The code can be found in https://github.com/cheliu-computation/M-FLAG-MICCAI2023.
更多查看译文
关键词
Vision-language model,Vision-language pre-training,Self-supervised learning
AI 理解论文
溯源树
样例
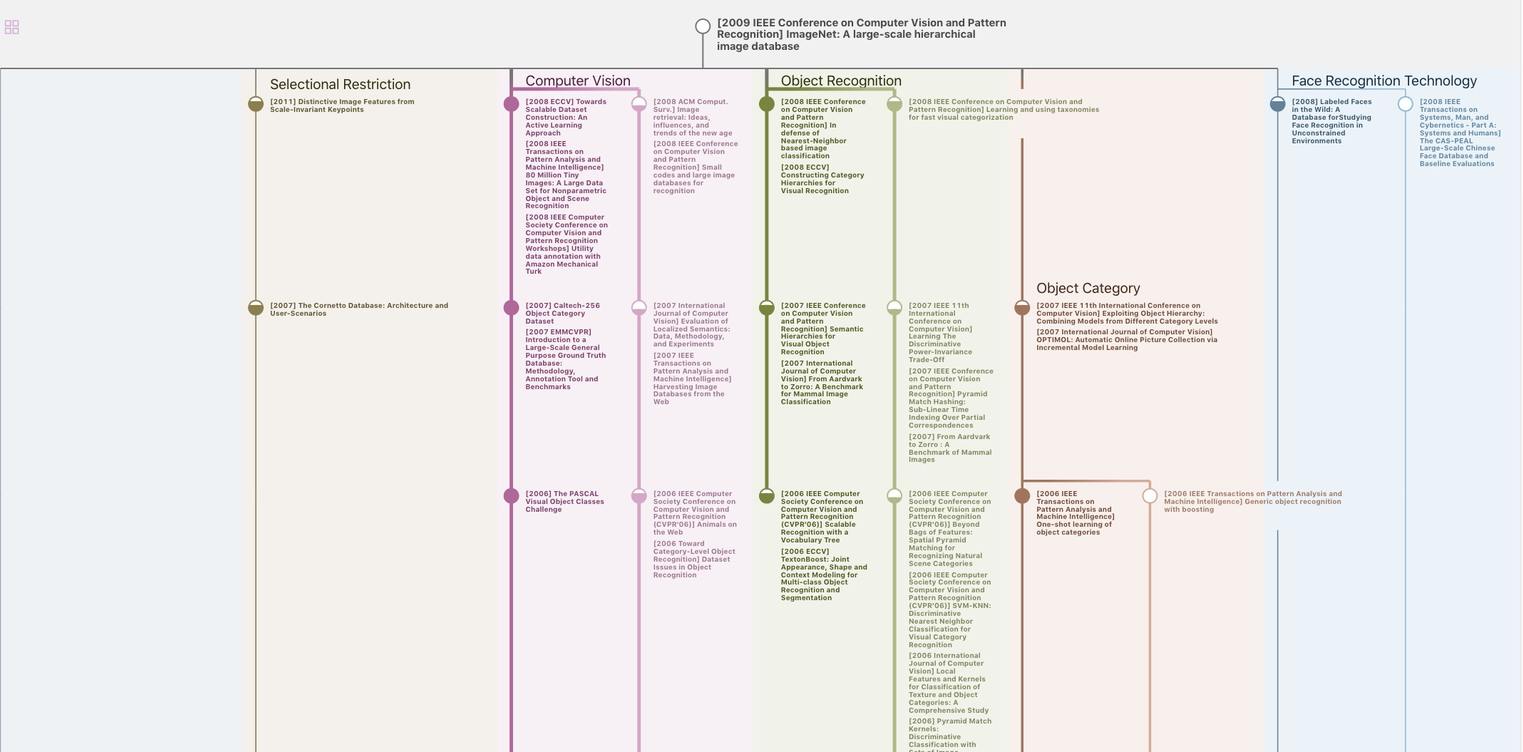
生成溯源树,研究论文发展脉络
Chat Paper
正在生成论文摘要