How time window influences biometrics performance: an EEG-based fingerprints connectivity study
arXiv (Cornell University)(2023)
摘要
EEG-based biometric represents a relatively recent research field that aims to recognize individuals based on their recorded brain activity by means of electroencephalography (EEG). Among the numerous features that have been proposed, connectivity-based approaches represent one of the more promising methods tested so far. In this paper, we investigate how the performance of an EEG biometric system varies with respect to different time windows to understand if it is possible to define the optimal duration of EEG signal that can be used to extract those distinctive features. Overall, the results have shown a pronounced effect of the time window on the biometric performance measured in terms of EER (equal error rate) and AUC (area under the curve), with an evident increase of the biometric performance with an increase of the time window. In conclusion, we want to highlight that EEG connectivity has the potential to represent an optimal candidate as EEG fingerprint and that, in this context, it is very important to define a sufficient time window able to collect the subject specific features. Moreover, our preliminary results show that extending the window size beyond a certain maximum does not improve biometric systems' performance.
更多查看译文
关键词
connectivity,time,performance,eeg-based
AI 理解论文
溯源树
样例
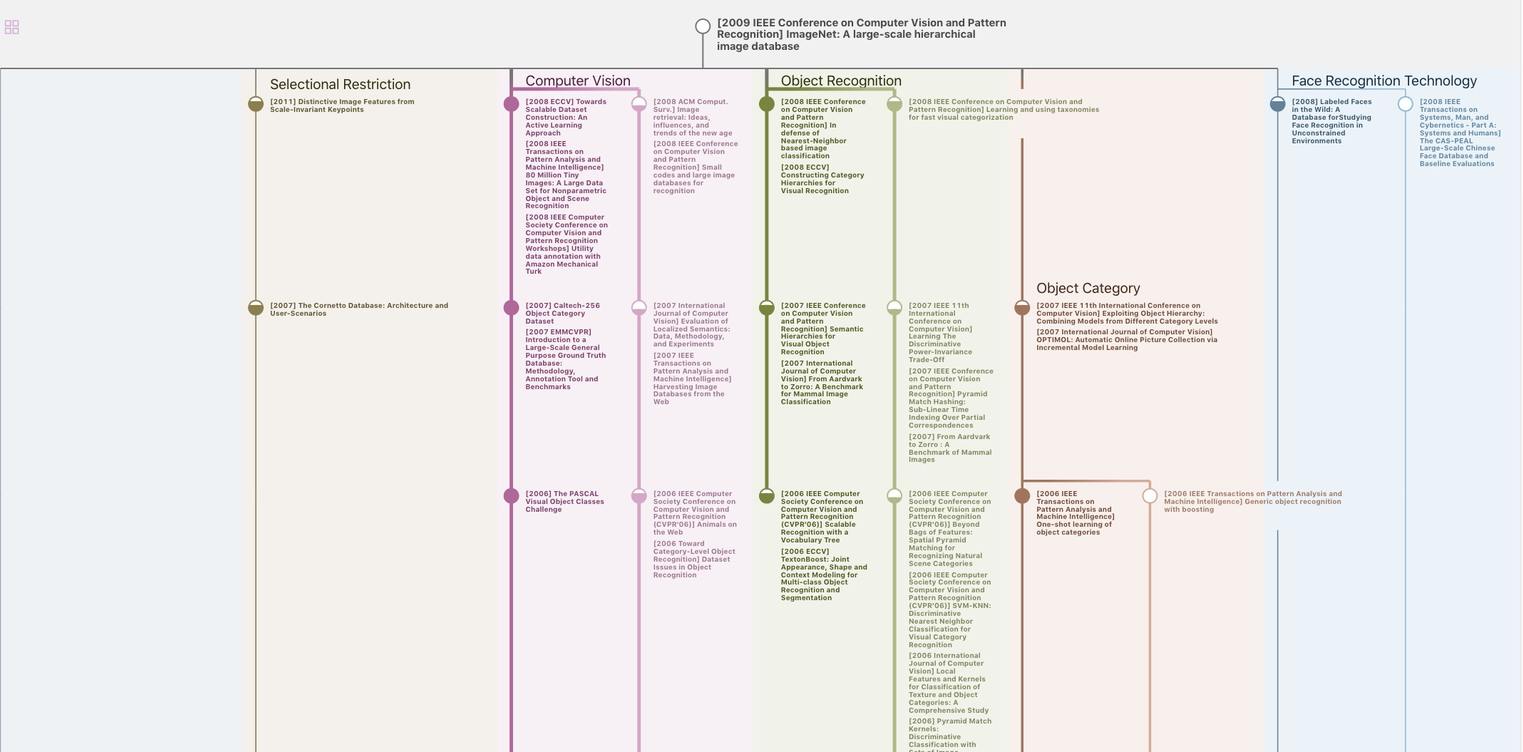
生成溯源树,研究论文发展脉络
Chat Paper
正在生成论文摘要