Adapting Large Language Model with Speech for Fully Formatted End-to-End Speech Recognition
ICASSP 2024 - 2024 IEEE International Conference on Acoustics, Speech and Signal Processing (ICASSP)(2023)
摘要
Most end-to-end (E2E) speech recognition models are composed of encoder and decoder blocks that perform acoustic and language modeling functions. Pretrained large language models (LLMs) have the potential to improve the performance of E2E ASR. However, integrating a pretrained language model into an E2E speech recognition model has shown limited benefits due to the mismatches between text-based LLMs and those used in E2E ASR. In this paper, we explore an alternative approach by adapting a pretrained LLMs to speech. Our experiments on fully-formatted E2E ASR transcription tasks across various domains demonstrate that our approach can effectively leverage the strengths of pretrained LLMs to produce more readable ASR transcriptions. Our model, which is based on the pretrained large language models with either an encoder-decoder or decoder-only structure, surpasses strong ASR models such as Whisper, in terms of recognition error rate, considering formats like punctuation and capitalization as well.
更多查看译文
关键词
Pretrained LM,LLM,fully formatted E2E ASR transcription
AI 理解论文
溯源树
样例
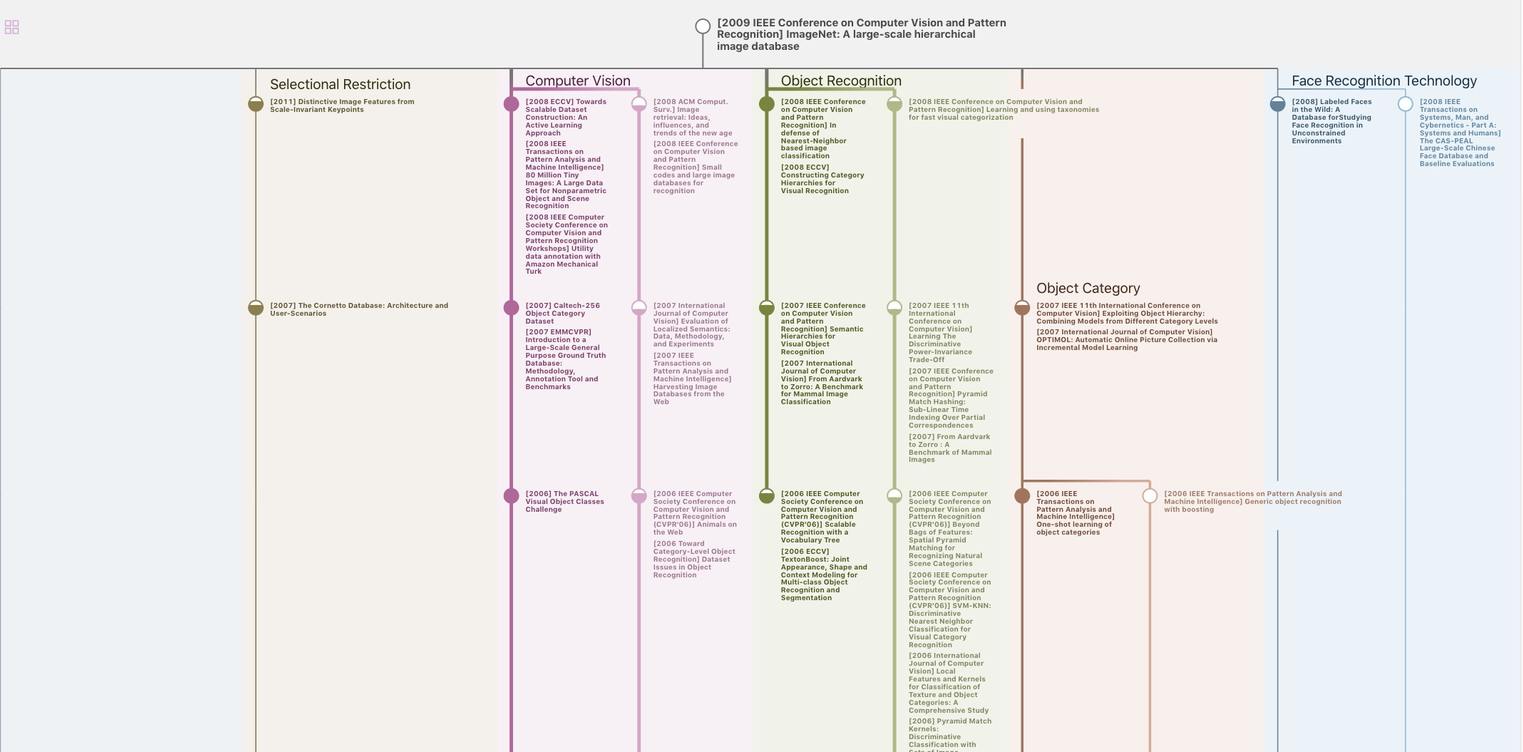
生成溯源树,研究论文发展脉络
Chat Paper
正在生成论文摘要