BASS: Block-wise Adaptation for Speech Summarization
CoRR(2023)
摘要
End-to-end speech summarization has been shown to improve performance over cascade baselines. However, such models are difficult to train on very large inputs (dozens of minutes or hours) owing to compute restrictions and are hence trained with truncated model inputs. Truncation leads to poorer models, and a solution to this problem rests in block-wise modeling, i.e., processing a portion of the input frames at a time. In this paper, we develop a method that allows one to train summarization models on very long sequences in an incremental manner. Speech summarization is realized as a streaming process, where hypothesis summaries are updated every block based on new acoustic information. We devise and test strategies to pass semantic context across the blocks. Experiments on the How2 dataset demonstrate that the proposed block-wise training method improves by 3 points absolute on ROUGE-L over a truncated input baseline.
更多查看译文
关键词
speech summarization,adaptation,bass,block-wise
AI 理解论文
溯源树
样例
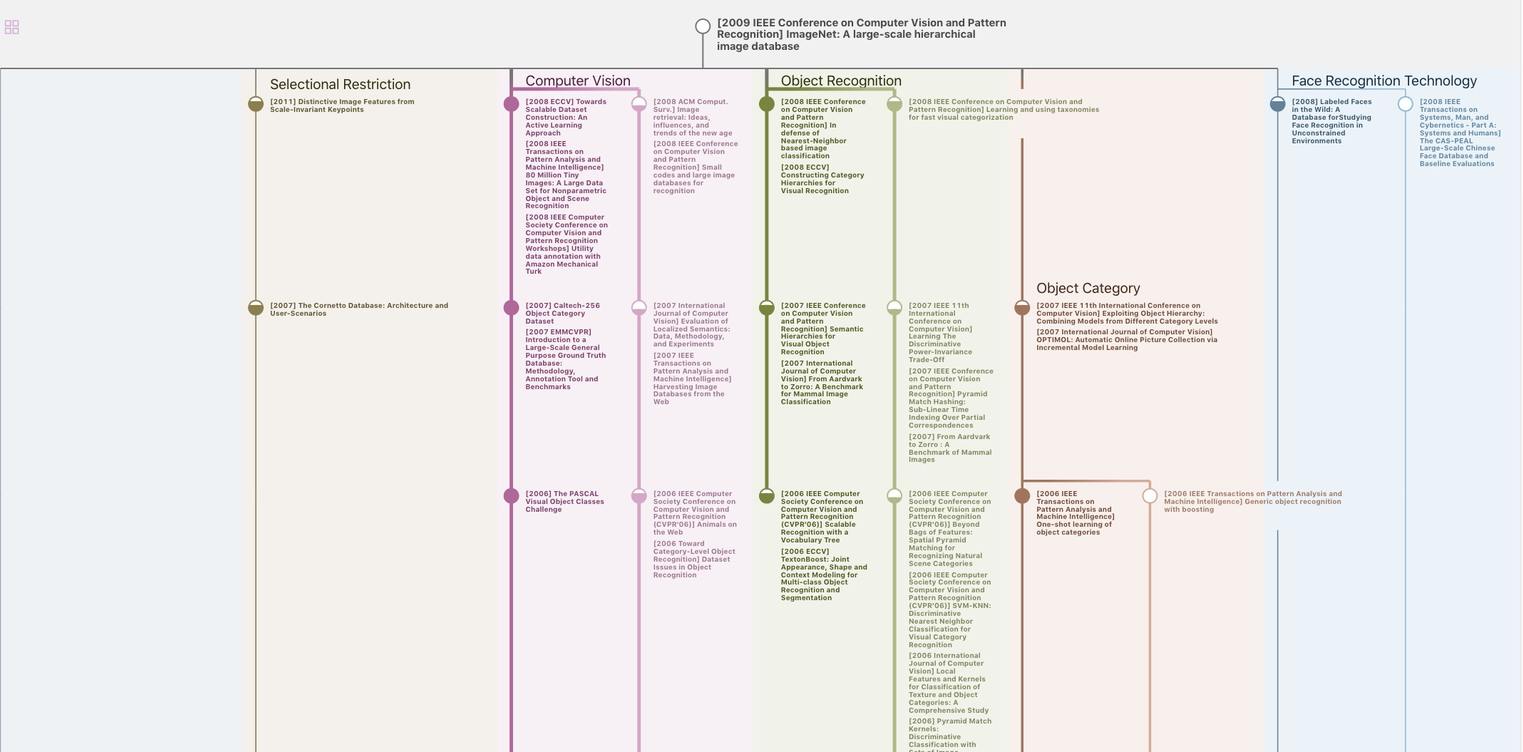
生成溯源树,研究论文发展脉络
Chat Paper
正在生成论文摘要