Boundary-Weighted Logit Consistency Improves Calibration of Segmentation Networks
MEDICAL IMAGE COMPUTING AND COMPUTER ASSISTED INTERVENTION, MICCAI 2023, PT III(2023)
摘要
Neural network prediction probabilities and accuracy are often only weakly-correlated. Inherent label ambiguity in training data for image segmentation aggravates such miscalibration. We show that logit consistency across stochastic transformations acts as a spatially varying regularizer that prevents overconfident predictions at pixels with ambiguous labels. Our boundary-weighted extension of this regularizer provides state-of-the-art calibration for prostate and heart MRI segmentation. Code is available at https://github.com/neerakara/BWCR.
更多查看译文
关键词
calibration,consistency regularization,segmentation
AI 理解论文
溯源树
样例
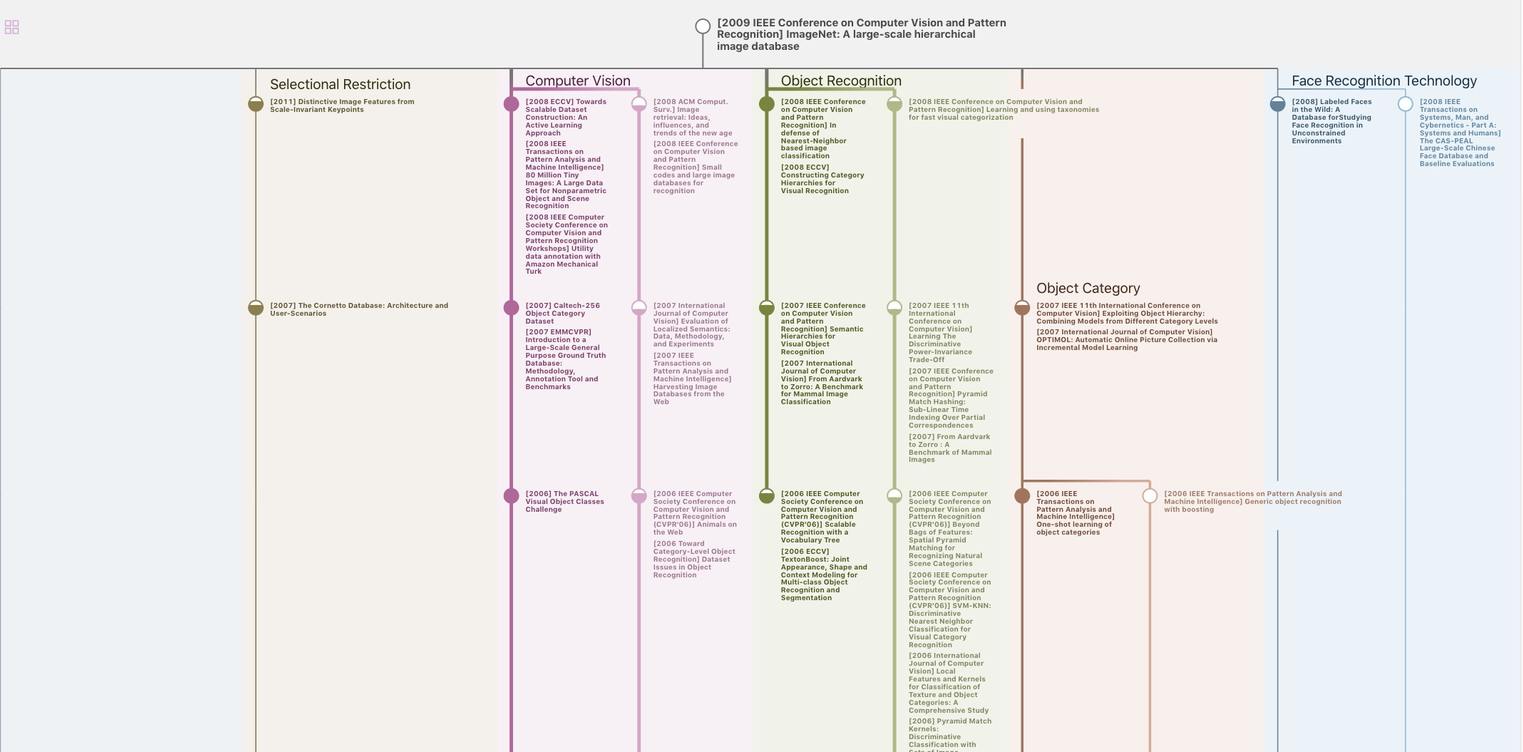
生成溯源树,研究论文发展脉络
Chat Paper
正在生成论文摘要