Predicting mechanical properties of carbon nanotube (cnt) images using multi-layer synthetic finite element model simulations
2023 IEEE INTERNATIONAL CONFERENCE ON IMAGE PROCESSING, ICIP(2023)
摘要
We present a pipeline for predicting mechanical properties of vertically-oriented carbon nanotube (CNT) forest images using a deep learning model for artificial intelligence (AI)-based materials discovery. Our approach incorporates an innovative data augmentation technique that involves the use of multi-layer synthetic (MLS) or quasi-2.5D images which are generated by blending 2D synthetic images. The MLS images more closely resemble 3D synthetic and real scanning electron microscopy (SEM) images of CNTs but without the computational cost of performing expensive 3D simulations or experiments. Mechanical properties such as stiffness and buckling load for the MLS images are estimated using a physics-based model. The proposed deep learning architecture, CNTNeXt, builds upon our previous CNTNet neural network, using a ResNeXt feature representation followed by random forest regression estimator. Our machine learning approach for predicting CNT physical properties by utilizing a blended set of synthetic images is expected to outperform single synthetic image-based learning when it comes to predicting mechanical properties of real scanning electron microscopy images. This has the potential to accelerate understanding and control of CNT forest self-assembly for diverse applications.
更多查看译文
关键词
Quasi-images,SEM Images,Prediction of Mechanical Properties,ResNeXt,Random Forest
AI 理解论文
溯源树
样例
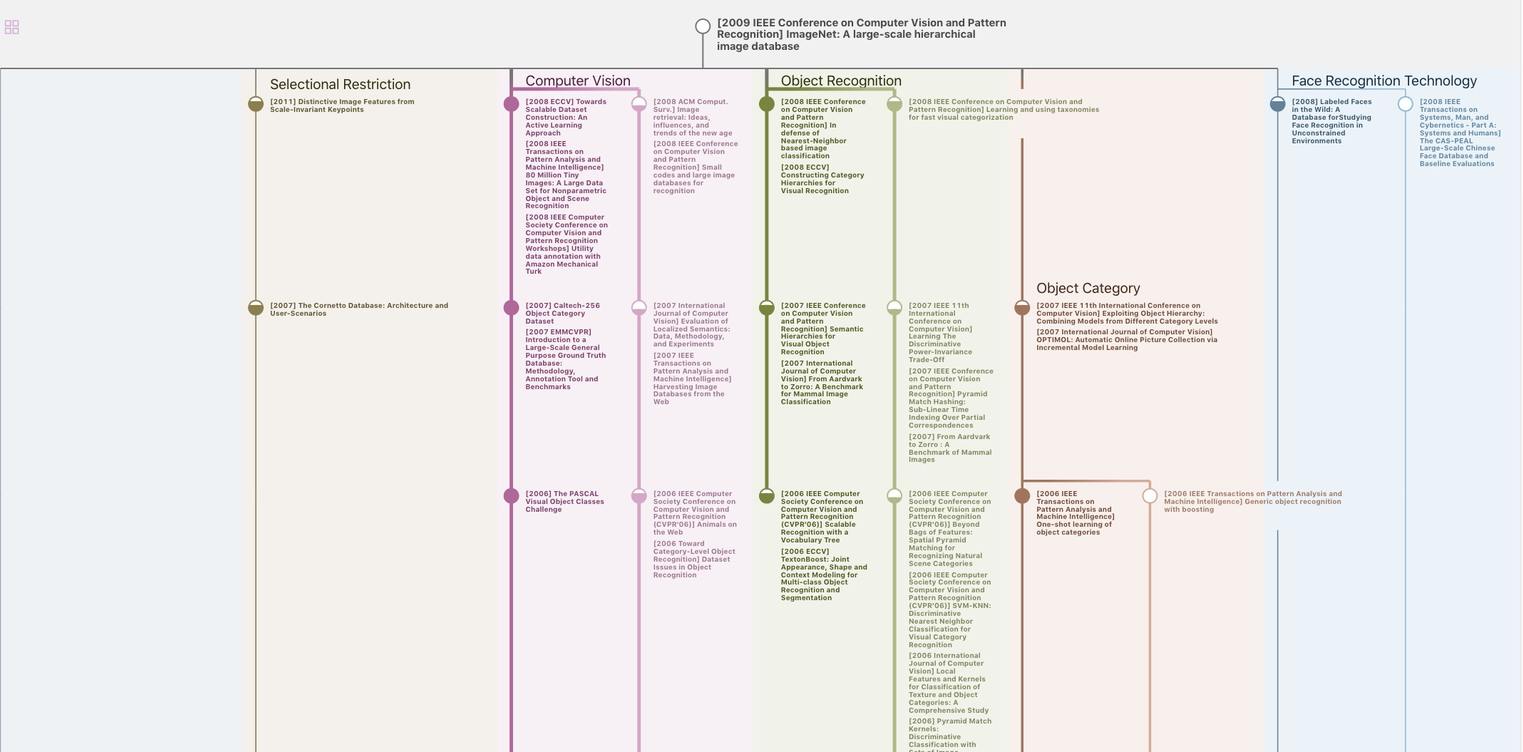
生成溯源树,研究论文发展脉络
Chat Paper
正在生成论文摘要